FLI Podcast: Lessons from COVID-19 with Emilia Javorsky and Anthony Aguirre
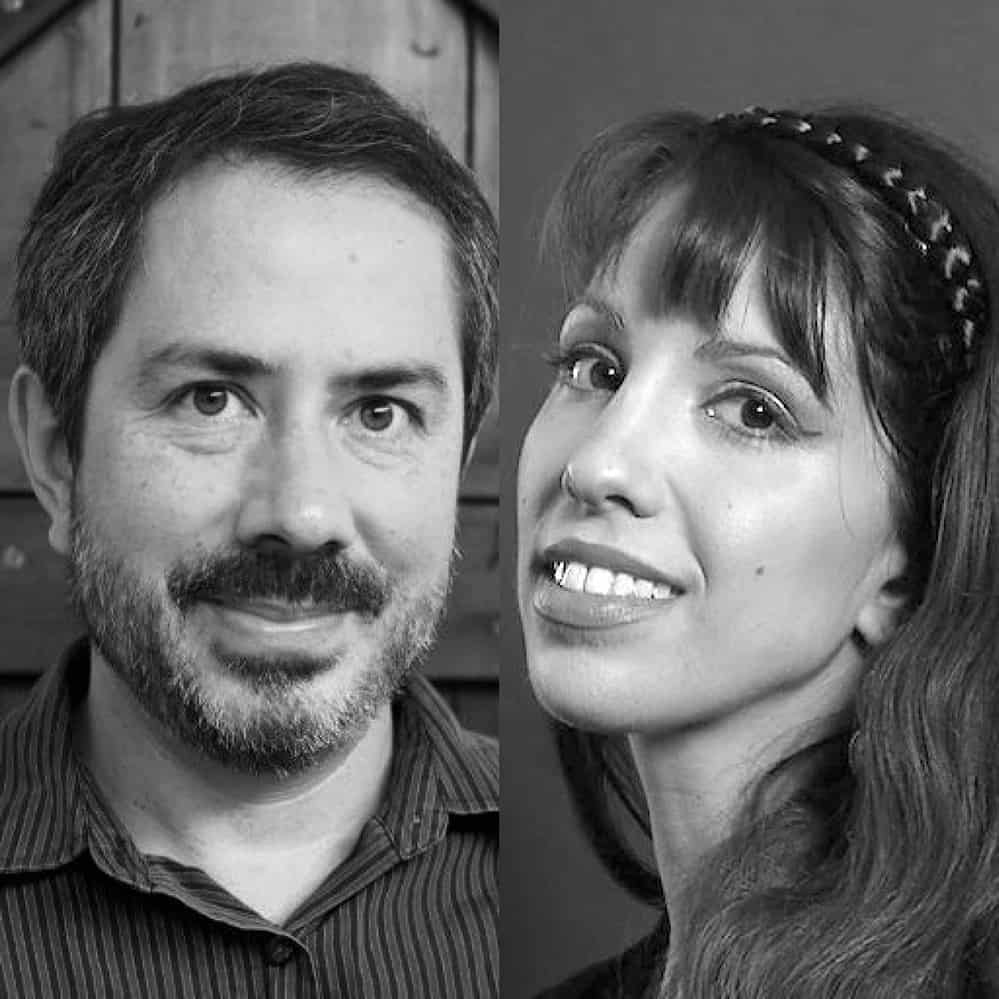
The global spread of COVID-19 has put tremendous stress on humanity’s social, political, and economic systems. The breakdowns triggered by this sudden stress indicate areas where national and global systems are fragile, and where preventative and preparedness measures may be insufficient. The COVID-19 pandemic thus serves as an opportunity for reflecting on the strengths and weaknesses of human civilization and what we can do to help make humanity more resilient. The Future of Life Institute's Emilia Javorsky and Anthony Aguirre join us on this special episode of the FLI Podcast to explore the lessons that might be learned from COVID-19 and the perspective this gives us for global catastrophic and existential risk.
Topics discussed in this episode include:
- The importance of taking expected value calculations seriously
- The need for making accurate predictions
- The difficulty of taking probabilities seriously
- Human psychological bias around estimating and acting on risk
- The massive online prediction solicitation and aggregation engine, Metaculus
- The risks and benefits of synthetic biology in the 21st Century
Timestamps:
0:00 Intro
2:35 How has COVID-19 demonstrated weakness in human systems and risk preparedness
4:50 The importance of expected value calculations and considering risks over timescales
10:50 The importance of being able to make accurate predictions
14:15 The difficulty of trusting probabilities and acting on low probability high cost risks
21:22 Taking expected value calculations seriously
24:03 The lack of transparency, explanation, and context around how probabilities are estimated and shared
28:00 Diffusion of responsibility and other human psychological weaknesses in thinking about risk
38:19 What Metaculus is and its relevance to COVID-19
45:57 What is the accuracy of predictions on Metaculus and what has it said about COVID-19?
50:31 Lessons for existential risk from COVID-19
58:42 The risk of synthetic bio enabled pandemics in the 21st century
01:17:35 The extent to which COVID-19 poses challenges to democratic institutions
This podcast is possible because of the support of listeners like you. If you found this conversation to be meaningful or valuable consider supporting it directly by donating at futureoflife.blackfin.biz/donate. Contributions like yours make these conversations possible.
All of our podcasts are also now on Spotify and iHeartRadio! Or find us on SoundCloud, iTunes, Google Play and Stitcher.
Transcript
Lucas Perry: Welcome to the Future of Life Institute Podcast. I’m Lucas Perry. Today’s episode is a special focused on lessons from COVID-19 with two members of the Future of Life Institute team, Anthony Aguirre and Emilia Javorsky. The ongoing coronavirus pandemic has helped to illustrate the frailty of human systems, the difficulty of international coordination on global issues and our general underpreparedness for risk. This podcast is focused on what COVID-19 can teach us about being better prepared for future risk from the perspective of global catastrophic and existential risk. The AI Alignment Podcast and the end of the month Future of Life Institute podcast will release as normally scheduled.
Anthony Aguirre has been on the podcast recently to discuss the ultimate nature of reality and problems of identity. He is a physicist that studies the formation, nature, and evolution of the universe, focusing primarily on the model of eternal inflation—the idea that inflation goes on forever in some regions of universe—and what it may mean for the ultimate beginning of the universe and time. He is the co-founder and Associate Scientific Director of the Foundational Questions Institute and is also a Co-Founder of the Future of Life Institute. He also co-founded Metaculus, which is something we get into during the podcast, which is an effort to optimally aggregate predictions about scientific discoveries, technological breakthroughs, and other interesting issues.
Emilia Javorsky develops tools to improve human health and wellbeing and has a background in healthcare and research. She leads clinical research and work on translation of science from academia to commercial setting at Artic Fox, and is the Chief Scientific Officer and Co-Founder of Sundaily, as well as the Director of Scientists Against Inhumane Weapons. Emilia is an advocate for the safe and ethical deployment of technology, and is currently heavily focused on lethal autonomous weapons issues.
And with that, let’s get into our conversation with Anthony and Emilia on COVID-19.
We're here to try and get some perspective on COVID-19 for how it is both informative surrounding issues regarding global catastrophic and existential risk and to see ways in which we can learn from this catastrophe and how it can inform existential risk and global catastrophic thought. Just to start off then, what are ways in which COVID-19 has helped demonstrate weaknesses in human systems and preparedness for risk?
Anthony Aguirre: One of the most upsetting things I think to many people is how predictable it was and how preventable it was with sufficient care taken as a result of those predictions. It's been known by epidemiologists for decades that this sort of thing was not only possible, but likely given enough time going by. We had SARS and MERS as kind of dry runs that almost were pandemics, but didn't have quite the right characteristics. Everybody in the community of people thinking hard about this, and I would like to hear more of Emilia's perspective on this knew that something like this was coming eventually. That it might be a few percent probable each year, but after 10 or 20 or 30 years, you start to get large probability of something like this happening. So it was known that it was coming eventually and pretty well known what needed to happen to be well prepared for it.
And yet nonetheless, many countries have found themselves totally unprepared or largely unprepared and unclear on what exactly to do and making very poor decisions in response to things that they should be making high quality decisions on. So I think part of what I'm interested in doing is thinking about why has that happened, even though we scientifically understand what's going on? We numerically model what could happen, we know many of the things that should happen in response. Nonetheless, as a civilization, we're kind of being caught off guard in a way and making a bad situation much, much worse. So why is that happening and how can we do it better now and next time?
Lucas Perry: So in short, the ways in which this is frustrating is that it was very predictable and was likely to happen given computational models and then also, lived experience given historical cases like SARS and MERS.
Anthony Aguirre: Right. This was not some crazy thing out of the blue, this was just a slightly worse version of things that have happened before. Part of the problem, in my mind, is the sort of mismatch between the likely cost of something like this and how many resources society is willing to put into planning and preparing and preventing it. And so here, I think a really important concept is expected value. So, the basic idea that when you're calculating the value of something that is unsure that you want to think about different probabilities for different values that that thing might have and combine them.
So for example, if I'm thinking I'm going to spend some money on something and there's a 50% chance that it's going to cost a dollar and there's a 50% chance that it's going to cost $1,000, so how much should I expect to pay for it? So on one hand, I don't know, it's a 50/50 chance, it could be a dollar, it could be $1,000, but if I think I'm going to do this over and over again, you can ask how much am I going to pay on average? And that's about 50% of a dollar plus 50% of $1,000 so about $500, $500 and 50 cents. The idea of thinking in terms of expected value is that when I have probabilities for something, I should always think as if I'm going to do this thing many, many, many times, like I'm going to roll the dice many, many times and I should reason in a way that makes sense if I'm going to do it a lot of times. So I'd want to expect that I'm going to spend something like $500 on this thing, even though that's not either of the two possibilities.
So, if we're thinking about a pandemic, if you imagine the cost just in dollars, let alone all the other things that are going to happen, but just purely in terms of dollars, we're talking about trillions of dollars. So if this was something that is going to cost trillions and trillions of dollars and there was something like a 10% chance of this happening over a period of a decade say, we should have been willing to pay hundreds and hundreds of billions of dollars to prevent this from happening or to dramatically decrease the cost when it does happen. And that is way, way, way orders of magnitude, more money than we have in fact spent on that.
So, part of the tricky thing is that people don't generally think in these terms, they think of "What is the most likely thing?" And then they plan for that. But if the most likely thing is relatively cheap and a fairly unlikely thing is incredibly expensive, people don't like to think about the incredibly expensive, unlikely thing, right? They think, "That's scary. I don't want to think about it. I'm going to think about the likely thing that's cheap." But of course, that's terrible planning. You should put some amount of resources into planning for the unlikely incredibly expensive thing.
And it's often, and it is in this case, that even a small fraction of the expected cost of this thing could have prevented the whole thing from happening in the sense that there's going to be trillions and trillions of dollars of costs. It was anticipated at 10% likely, so it's hundreds of billions of dollars that in principle society should have been willing to pay to prevent it from happening, but even a small fraction of that, in fact, could have really, really mitigated the problem. So it's not even that we actually have to spend exactly the amount of money that we think we will lose in order to prevent something from happening.
Even a small fraction would have done. The problem is that we spend not even close to that. These sorts of situations where there's a small probability of something extraordinarily costly happening, our reaction in society tends to be to just say, "It's a small probability, so I don't want to think about it." Rather than "It's a small probability, but the cost is huge, so I should be willing to pay some fraction of that small probability times that huge cost to prevent it from happening." And I think if we could have that sort of calculation in mind a little bit more firmly, then we could prevent a lot of terrible things from happening at a relatively modest investment. But the tricky thing is that it's very hard to take seriously those small probability, high cost things without really having a firm idea of what they are, what the probability of that happening is and what the cost will be.
Emilia Javorsky: I would add to that, but in complete agreement with Anthony, part of what is at issue here too is needing to think overtime scales, because if something has a certain probability that is small at any given short term horizon, but that probability rises to something that's more significant with a tremendously high cost over a longer term time scale, you need to be able to be willing to think on those longer term timescales in order to act. And from the perspective of medicine, this is something we've struggled with a lot, at both the individual level, at the healthcare system level and at the societal public health policy level, is that prevention, while we know it's much cheaper to prevent a disease than to treat it, the same thing with pandemic preparedness, a lot of the things we're talking about were actually quite cheap mitigation measures to put in place. Right now, we're seeing a crisis of personal protective equipment.
We're talking about basic cheap supplies like gloves and masks and then national stockpiles of ventilators. These are very basic, very conserved across any pandemic type, right? We know that in all likelihood when a pandemic arises, it is some sort of respiratory borne illness. Things like masks and respirators are a very wise thing to stockpile and have on hand. Yet despite having several near misses, even in the very recent past, we're talking about the past 20 years, there was not a critical will or a critical lobby or a critical voice that enabled us to do these very basic, relatively cheap measures to be prepared for something like this to happen.
If you talk about something like vaccine development, that's something that you need to prepare pretty much in real time. That's pathogen specific, but the places that were fumbling to manage this epidemic today are things that were totally basic, cheap and foreseeable. We really need to find ways in the here and now to motivate thinking on any sort of longterm horizon. Not even 50 years, a hundred years down the line, but one to five years are things that we struggle with.
Anthony Aguirre: To me, another surprising thing has been the sudden discovery of how important it is to be able to predict things. It's of course, always super important. This is what we do throughout our life. We're basically constantly predicting things, predicting the consequences of certain actions or choices we might make, and then making those choices dependent on which things we want to have happen. So we're doing it all the time and yet when confronted with this pandemic, suddenly, we extra super realize how important it is to have good predictions, because what's unusual I would say about a situation like this is that all of the danger is sort of in the future. If you look at it in any given time, you say, "Oh, there's a couple of dozen cases here in my county, everything's under control." Unbelievably ineffective and wishful thinking, because of course, the number of cases is growing exponentially and by the time you notice that there's any problem that's of significance at all, the next day or the next few days, it's going to be doubly as big.
So the fact that things are happening exponentially in a pandemic or an epidemic, makes it incredibly vital that you have the ability to think about what's going to happen in the future and how bad things can get quite quickly, even if at the moment, everything seems fine. Everybody who thinks in this field or who just is comfortable with how exponentials work know this intellectually, but it still isn't always easy to get the intuitive feeling for that, because it just seems like so not a big deal for so long, until suddenly it's the biggest thing in the world.
This has been a particularly salient lesson that we really need to understand both exponential growth and how to do good projections and predictions about things, because there could be lots of things that are happening under the radar. Beyond the pandemic, there are lots of things that are exponentially growing that if we don't pay attention to the people who are pointing out those exponentially growing things and just wait until they're a problem, then it's too late to do anything about the problem.
At the beginning stages, it's quite easy to deal with. If we take ourselves back to sometime in late December, early January or something, there was a time where this pandemic could have easily been totally prevented by the actions of the few people, if they had just known exactly what the right things to do were. I don't think you can totally blame people for that. It's very hard to see what it would turn into, but there is a time at the beginning of the exponential where action is just so much easier and every little bit of delay just makes it incredibly harder to do anything about it. It really brings home how important it is to have good predictions about things and how important it is to believe those predictions if you can and take decisive action early on to prevent exponentially growing things from really coming to bite you.
Lucas Perry: I see a few central issues here and lessons from COVID-19 that we can draw on. The first is that this is something that was predictable and was foreseeable and that experts were saying had a high likelihood of happening, and the ways in which we failed were either in the global system, there aren't the kinds of incentives for private organizations or institutions to work towards mitigating these kinds of risks or people just aren't willing to listen to experts making these kinds of predictions. The second thing seems to be that even when we do have these kinds of predictions, we don't know how basic decision theory works and we're not able to feel and intuit the reality of exponential growth sufficiently well. So what are very succinct ways of putting solutions to these problems?
Anthony Aguirre: The really hard part is having probabilities that you feel like you can trust. If you go to a policy maker and tell them there's a danger of this thing happening, maybe it's a natural pandemic, maybe it's a human engineered pandemic or a AI powered cyber attack, something that if it happens, is incredibly costly to society and you say, "I really think we should be devoting some resources to preventing this from happening, because I think there's a 10% chance that this is going to happen in the next 10 years." They're going to ask you, "Where does that 10% chance come from?" And "Are you sure that it's not a 1% chance or a 0.1% chance or a .00001% chance?" And that makes a huge difference, right? If something really is a tiny, tiny fraction of a percent likely, then that plays directly into how much effort you should go in to preventing it if it has some fixed cost.
So I think the reaction that people have often to low probability, high cost things is to doubt exactly what the probability is and having that doubt in their mind, just avoid thinking about the issue at all, because it's so easy to not think about it if the probability is really small. A big part of it is really understanding what the probabilities are and taking them seriously. And that's a hard thing to do, because it's really, really hard to estimate what the probabilities say of a gigantic AI powered cyber attack is, where do you even start with that? It has all kinds of ingredients that there's no model for, there's no set quantitative assessment strategy for it. That's a part of the root of the conundrum that even for things like this pandemic that everybody knew was coming at some level, I would say nobody knew whether it was a 5% chance over 10 years or a 50% chance over 10 years.
It's very hard to get firm numbers, so one thing I think we need are better ways of assessing probabilities of different sorts of low probability, high cost things. That's something I've been working a lot on over the past few years in the form of Metaculus which maybe we can talk about, but I think in general, most people and policy makers can understand that if there's some even relatively low chance of a hugely costly thing that we should do some planning for it. We do that all the time, we do it with insurance, we do it with planning for wars. There are all kinds of low probability things that we plan for, but if you can't tell people what the probability is and it's small and the thing is weird, then it's very, very hard to get traction.
Emilia Javorsky: Part of this is how do we find the right people to make the right predictions and have the ingredients to model those out? But the other side of this is how do we get the policy makers and decision makers and leaders in society to listen to those predictions and to have trust and confidence in them? From the perspective of that, when you're communicating something that is counterintuitive, which is how many people end up making decisions, there really has to be a foundation of trust there, where you're telling me something that is counterintuitive to how I would think about decision making and planning in this particular problem space. And so, it has to be built on a foundation and trust. And I think one of the things that characterize good models and good predictions is exactly as you say, they're communicated with a lot of trepidation.
They explain what the different variables are that go into them and the uncertainty that bounds each of those variables and an acknowledgement that some things are known and unknown. And I think that's very hard in today's world where information is always at maximum volume and it's very polarized and you're competing against voices, whether they be in a policy maker's ear or a CEO's ear, that will speak in absolutes and speak in levels of certainty, overestimating risk, or underestimating risk.
That is the element that is necessary for these predictions to have impact is how do you connect ambiguous and qualified and cautious language that characterizes these kind of long term predictions with a foundation of trust so people can hear and appreciate those and you don't get drowned out by the noise on either side of things that are much likely to be less well founded if they're speaking in absolutes and problem spaces that we know just have a tremendous amount of uncertainty.
Anthony Aguirre: That's a very good point. You're mentioning of the kind of unfamiliarity with these things is an important one in the sense that, as an individual, I can think of improbable things that might happen to me and they seem, well, that's probably not going to happen to me, but I know intellectually it will and I can look around the world and see that that improbable thing is happening to lots of people all the time. Even if there's kind of a psychological barrier to my believing that it might happen to me, I can't deny that it's a thing and I can't really deny what sort of probability it might have to happen to me, because I see it happening all around. Whereas when we're talking about things that are happening to a country or a civilization, we don't have a whole lot of statistics on them.
We can't just say of all the different planets that are out there with civilizations like ours, 3% of them are undergoing pandemics right now. If we could do that then we could really count on those probabilities. We can't do that. We can look historically at what happened in our world, but of course, since it's really changing dramatically over the years, that's not always such a great guide and so, we're left with reasoning by putting together scientific models, all the uncertainties that you were mentioning that we have to feed into those sorts of models or just other ways of making predictions about things through various means and trying to figure out how can we have good confidence in those predictions. And this is an important point that you bring up, not so much in terms of certainty, because there are all of these complex things that we're trying to predict about the possibility of good or bad things happening to our society as a whole, none of them can be predicted with certainty.
I mean, almost nothing in the world can be predicted with certainty, certainly not these things, and so it's always a question of giving probabilities for things and both being confident in those probabilities and taking seriously what those probabilities mean. And as you say, people don't like that. They want to be told what is going to happen or what isn't going to happen and make a decision on that basis. That is unfortunately not information that's available on most important things and so, we'd have to accept that they're going to be probabilities, but then where do we them from? How do we use them? There's a science and an art to that I think, and a subtlety to it as you say, that we really have to get used to and get comfortable with.
Lucas Perry: There seems to be lots of psychological biases and problems around human beings understanding and fully integrating probabilistic estimations into our lives and decision making. I'm sure there's probably literature that already exists upon this, but it would be skillful I think to apply it to existential and global catastrophic risk. So, assuming that we're able to sufficiently develop our ability to generate accurate and well-reasoned probabilistic estimations of risks, and Anthony, we'll get into Metaculus shortly, then you mentioned that the prudent and skillful thing to do would be to feed that into a proper decision theory, which explain a little bit more about the nerdy side of that if you feel it would be useful, and in particular, you talked a little bit about expected value, could you say a little bit more about how if policy and government officials were able to get accurate probabilistic reasoning and then fed it into the correct decision theoretic models that it would produce better risk mitigation efforts?
Anthony Aguirre: I mean, there's all kinds of complicated discussions and philosophical explorations of different versions of decision theory. We really don't need to think about things in such complicated terms in the sense that what it really is about is just taking expected values seriously and thinking about actions we might take based on how much value we expect given each decision. When you're gambling, this is exactly what you're doing, you might say, "Here, I've got some cards in my hand. If I draw, there's a 10% chance that I'll get nothing and a 20% chance that I'll get a pair and a tiny percent chance that I'll fill out my flush or something." And with each of those things, I want to think of, "What is the probable payoff when I have that given outcome?" And I want to make my decisions based on the expected value of things rather than just what is the most probable or something like that.
So it's a willingness to quantitatively take into account, if I make decision A, here is the likely payoff of making decision A, if I make decision B, here's the likely payoff that is the expected value of my payoff in decision B, looking at which one of those is higher and making that decision. So it's not very complicated in that sense. There are all kinds of subtleties, but in practice it can be very complicated because usually you don't know, if I make decision A, what's going to happen? If I make decision B, what's going to happen? And exactly what value can I associate with those things? But this is what we do all the time, when we weigh the pros and cons of things, we're kind of thinking, "Well, if I do this, here are the things that I think are likely to happen. Here's what I think I'm going to feel and experience and maybe gain in doing A, let me think through the same thing in my mind with B and then, which one of those feels better is the one that I do."
So, this is what we do all the time on an intuitive level, but we can do quantitative and systematic method of it. If we are more carefully thinking about what the actual numerical and quantitative implications of something are and if we have actual probabilities that we can assign to the different outcomes in order to make our decision. All of this, I think, is quite well known to decision makers of all sorts. What's hard is that often decision makers won't really have those sorts of tools in front of them. They won't have ability to look at different possibilities, ability to attribute probabilities and costs and payoffs to those things in order to make good decisions. So those are tools that we could put in people's hands and I think would just allow people to make better decisions.
Emilia Javorsky: And what I like about what you're saying, Anthony, implicit in that is that it's a standardized tool. The way you assign the probabilities and decide between different optionalities is standardized. And I think one thing that can be difficult in the policy space is different advocacy groups or different stakeholders will present data and assign probabilities based on different assumptions and vested interests, right? So, when a policy maker is making a decision, they're using probabilities and using estimates and outcomes that are developed using completely different models with completely different assumptions and different biases baked into them and different interests baked into them. What I think is so vital is to make sure as best one can, again knowing the inherent ambiguity that's existing in modeling in general, that you're having an apples to apples comparison when you're assigning different probabilities and making decisions based off of them.
Anthony Aguirre: Yeah, that's a great point that part of the problem is that people are just used to probabilities not meaning anything because they're often given without context, without explanation and by groups that have a vested interest in them looking a certain way. If I ask someone, what's the probability that this thing is going to happen, and they'd tell me 17%, I don't know what to do with that. Do I believe them? I mean, on what basis are they telling me 17%? In order for me to believe that, I have to either have an understanding of what exactly went into that 17% and really agree step-by-step with all their assumptions and modeling and so on, or maybe I have to believe them from some other reason.
Like they've provided probabilities for lots of things before, and they've given accurate probabilities for all these different things that they provided, so I kind of trust their ability to give accurate probabilities. But usually that's not available. That's part of the problem. Our general lesson has been if people are giving you probabilities, usually they don't mean much, but that's not always the case. There are probabilities we use all the time, like for the weather where we more or less know what they mean. You see that there's a 15% chance of rain.
That's a meaningful thing, and it's meaningful because both of you sort of trust that the weather people know what they're doing, which they sort of do, and it's meaningful in that it has a particular interpretation, which is that if I look at the weather forecast for a year and look at all the days where it said that there was a 15% chance of rain, about 15% of all those days it will have been raining. There's a real meaning to that, and those numbers come from a careful calibration of weather models for exactly that reason. When you get 15% chance of rain from the weather forecast, what that generally means is that they've run a whole bunch of weather models with slightly different initial conditions and in 15% of them it's raining today in your location.
They're carefully calibrated usually, like the National Weather Service calibrates them, so that it really is true that if you look at all the days of, whatever, it's 15% chance, about 15% of those days it was in fact raining. Those are probabilities that you can really use and you can say, "15% chance of rain, is it worth taking an umbrella? The umbrella is kind of annoying to carry around. Am I willing to take my chances for 15%? Yeah, maybe. If it was 30%, I'd probably take the umbrella. If it was 5%, I definitely wouldn't." That's a number that you can fold into your decision theory because it means something. Whereas when somebody says, "There's a 18% chance at this point that some political thing is going to happen, that some bill is going to pass," maybe that's true, but you have no idea where that 18% comes from. It's really hard to make use of it.
Lucas Perry: Part of them proving this getting prepared for risks is better understanding and taking seriously the reasoning and reasons behind different risk estimations that experts or certain groups provide. You guys explained that there are many different vested interests or interest groups who may be biasing or framing percentages and risks in a certain way, so that policy and action can be directed towards things which may benefit them. Are there other facets to our failure to respond here other than our inability to take risks seriously?
Emilia Javorsky: If we had a sufficiently good understanding of the probabilities and we were able to see all of the reasons behind the probabilities and take them all seriously, and then we took those and we fed them into a standardized and appropriate decision theory, which used expected value calculations and some agreed upon risk tolerance to determine how much resources should be put into mitigating risks, are there other psychological biases or weaknesses in human virtue that would still lead to us insufficiently acting on these risks? An example that comes to mind maybe of something like a diffusion of responsibility.
That's very much what COVID-19 in many ways has played out to be, right? We kind of started this with the assumptions that this was quite a foreseeable risk, and any which way you looked at the probabilities, it was a sufficiently high probability that basic levels of preparedness and a robustness of preparedness should have been employed. I think what you allude to in terms of diffusion of responsibility is certainly one aspect of it. It's difficult to say where that decision-making fell apart, but we did hear very early on a lot of discussion of this is something that is a problem localized to China.
Anyone that has any familiarity with these models would have told you, "Based on the probabilities we already knew about, plus what we're witnessing from this early data, which was publicly available in January, we had a pretty good idea of what was going on, that this would become something that was in all likelihood be global." This next question becomes, why wasn't anything done or acted on at that time? I think part of that comes with a lack of advocacy and a lack of having the ears of the key decision makers of what was actually coming. It is very, very easy when you have to make difficult decisions to listen to the vocal voices that tell you not to do something and provide reasons for inaction.
Then the voices of action are perhaps more muted coming from a scientific community, spoken in language that's not as definitive as the other voices in the room and the other stakeholders in the room that have a vested interest in policymaking. The societal incentives to act or not act aren't just from a pure, what's the best long-term course of action, they're very, very much vested in what are the loudest voices in the room, what is the kind of clout and power that they hold, and weighing those. I think there's a very real political and social atmosphere and economic atmosphere that this happens in that dilutes some of the writing that was very clearly on the wall of what was coming.
Anthony Aguirre: I would add I think that it's especially easy to ignore something that is predicted and quite understandable to experts who understand the dynamics of it, but unfamiliar or where historically you've seen it turn out the other way. Like on one hand, we had multiple warnings through near pandemics that this could happen, right? We had SARS and MERS and we had H1N1 and there was Ebola. All these things were clear indications of how possible it was for this to happen. But at the same time, you could easily take the opposite lesson, which is yes, an epidemic arises in some foreign country and people go and take care of it and it doesn't really bother me.
You can easily take the lesson from that that the tendency of these things is to just go away on their own and the proper people will take care of them and I don't have to worry about this. What's tricky is understanding from the actual characteristics of the system and your understanding of the system what makes it different from those other previous examples. In this case, something that is more transmissible, transmissible when it's not very symptomatic, yet has a relatively high fatality rate, not very high like some of these other things, which would have been catastrophic, but a couple of percent or whatever it turns out to be.
I think people who understood the dynamics of infectious disease and saw high transmissibility and potential asymptomatic transmission and a death rate that was much higher than the flu immediately put those three things together and saw, oh my god, this is a major problem and a little bit different from some of those previous ones that had a lower fatality rate or were very, very obviously symptomatic when they were transmissible, and so it was much easier to quarantine people and so on. Those characteristics you can understand if you're trained for that sort of thing to look for it, and those people did, but if not, you just sort of see it as another far away disease in a far off land that people will take care of and it's very easy to dismiss it.
I think it's not really a failure of imagination, but a failure to take seriously something that could happen that is perfectly plausible just because something like it hasn't really happened like that before. That's a very dangerous one I think.
Emilia Javorsky: It comes back to human nature sometimes and the frailty of our biases and our virtue. It's very easy to convince yourself and recall examples where things did not come to pass. Because dealing with the reality of the negative outcome that you're looking at, even if it looks like it has a fairly high probability, is something that is innately adverse for people, right? We look at negative outcomes and we look for reasons that those negative outcomes will not come to pass.
It's easy to say, "Well, yes, it's only let's say a 40% probability and we've had these before," and it becomes very easy to identify reasons and not look at a situation completely objectively as to why the best course of action is not to take the kind of drastic measures that are necessary to avoid the probability of the negative outcome, even if you know that it's likely to come to pass.
Anthony Aguirre: It's even worst that when people do see something coming and take significant action and mitigate the problem, they rarely get the sort of credit that they should.
Emilia Javorsky: Oh, completely.
Anthony Aguirre: Because you never see the calamity unfold that they avoided.
Emilia Javorsky: Yes.
Anthony Aguirre: The tendency will be, "Oh, you overreacted, or oh, that was never a big problem in the first place." It's very hard to piece together like Y2K. I think it's still unclear, at least it is to me, what exactly would have happened if we hadn't made a huge effort to mitigate Y2K. There are many similar other things where it could be that there really was a calamity there and we totally prevented it by just being on top of it and putting a bunch of effort in, or it could be that it wasn't that big of a deal, and it's very, very hard to tell in retrospect.
That's another unfortunate bias that if we could see the counterfactual world in which we didn't do anything about Y2K and saw all this terrible stuff unfold, then we could make heroes out of the people that put all that effort in and sounded the warning and did all the mitigation. But we don't see that. It's rather unrewarding in a literal sense. It's just you don't get much reward for preventing catastrophes and you get lots of blame if you don't prevent them.
Emilia Javorsky: This is something we deal with all the time on the healthcare side of things. This is why preventative health and public health and basic primary care really suffer to get the funding, get the attention that they really need. It's exactly this. Nobody cares about the disease that they didn't get, the heart attack they didn't have, the stroke that they didn't have. For those of us that come from a public health background, it's been kind of a collective banging our head against the wall for a very long time because we know looking at the data that this is the best way to take care of population level health.
Emilia Javorsky: Yet knowing that and having the data to back it up, it's very difficult to get the attention across all levels of the healthcare system, from getting the individual patient on board all the way up to how do we fund healthcare research in the US and abroad.
Lucas Perry: These are all excellent points. What I'm seeing from everything that you guys said is to back it up to what Anthony said quite while ago, there is a kind of risk exceptionalism where we feel that our country or ourselves won't be exposed to catastrophic risks. It's other people's families who lose someone in a car accident but not mine, even though the risk of that is fairly high. There's this second kind of bias going on that acting on risk in order to mitigate it based off pure reasoning alone seems to be very difficult, especially when the intervention to mitigate the risk is very expensive because it requires a lot of trust in the experts and the reasoning that goes behind it, like spending billions of dollars to prevent the next pandemic.
It feels more tangible and intuitive now, but maybe for people of newer generations it felt a little bit more silly and would have had to have been more of a rational cognitive decision. Then the last thing here seems to be that there's asymmetry between different kinds of risks. Like if someone mitigates a pandemic from happening, it's really hard to appreciate how good that was of a thing to do, but that seems to not be true of all risks. For example, with risks where the risk actually just exists somewhere like in a lab or a nuclear missile silo. For example, people like Stanislav Petrov and Vasili Arkhipov we're able to appreciate it very easily just because there was a concrete event and there was a big dangerous thing and they have stopped it from happening.
It seems also skillful here to at least appreciate which kinds of risks are the kinds where if they would have happened, but they didn't because we prevented them, we can notice that versus the kinds of risks where if we stop them from happening, we can't even notice that we stopped it from happening. Adjusting our attitude towards those with each feature would seem skillful. Let's focus in then on making good predictions. Anthony, earlier you brought up Metaculus, could you explain what Metaculus is and what it's been doing and how it's been involved in COVID-19?
Anthony Aguirre: Metaculus is at some level an effort to deal with precisely the problem that we've been discussing, that it's difficult to make predictions and it's difficult to have a reason to trust predictions, especially when they're probabilistic ones about complicated things. The idea of Metaculus is sort of twofold or threefold maybe I would say. One part of it is that it's been shown through the years and this is work by Tetlock and The Good Judgment Project and a whole series of projects within IARPA, the Intelligence Advanced Research Projects Agency, that groups of people making predictions about things and having those predictions carefully combined can make better predictions often than even small numbers of experts. There tend to be kind of biases on different sides.
If you carefully aggregate people's predictions, you can at some level wash out those biases. As well, making predictions is something that some people are just really good at. It's a skill that varies person to person and can be trained. There are people who are just really good at making predictions across a wide range of domains. Sometimes in making a prediction, general prediction skill can trump actual subject matter expertise. Of course, it's good to have both if you possibly can, but lots of times experts have a huge understanding of the subject matter.
But if they're not actually practiced or trained or spend a lot of time making predictions, they may not make better predictions than someone who is really good at making predictions, but has less depth of understanding of the actual topic. That's something that some of these studies made clear. The idea of combining those two is to create a system that solicits predictions from lots of different people on questions of interest, aggregates those predictions, and identifies which people are really good at making predictions and kind of counts their prediction and input more heavily than other people.
So that if someone has just a year's long track record of over and over again making good predictions about things, they have a tremendous amount of credibility and that gives you a reason to think that they're going to make good predictions about things in the future. If you take lots of people, all of whom are good at making predictions in that way and combine their predictions together, you're going to get something that's much, much more reliable than just someone off the street or even an expert making a prediction in a one-off way about something.
That's one aspect of it is identify good predictors, have them accrue a very objective track record of being right, and then have them in aggregate make predictions about things that are just going to be a lot more accurate than other methods you can come up with. Then the second thing, and it took me a long time to really see the importance of this, but I think our earlier conversation has kind of brought this out, is that if you have a single system or a single consistent set of predictions and checks on those predictions. Metaculus is a system that has many, many questions that have had predictions made on them and have resolved that has been checked against what actually happened.
What you can do then is start to understand what does it mean when Metaculus as a system says that there's a 10% chance of something happening. You can really say of all the things on Metaculus that have a 10% chance of happening, about 10% of those actually happen. There's a meaning to the 10%, which you can understand quite well, that if you say I went to Metaculus and where to go and make bets based on a whole bunch of predictions that were on it, you would know that the 10% predictions on Metaculus come true about 10% of the time, and you can use those numbers and actually making decisions. Whereas when you go to some random person and they say, "Oh, there's a 10% chance," as we discussed earlier, it's really hard to know what exactly to make of that, especially if it's a one-off event.
The idea of Metaculus was to both make a system that makes highly accurate predictions as best as possible, but also a kind of collection of events that have happened or not happened in the world that you can use to ground the probabilities and give meaning to them, so that there's some operational meaning to saying that something on the system has a 90% chance of happening. This has been going on since about 2014 or '15. It was born basically at the same time as the Future of Life Institute actually for very much the same reason, thinking about what can we do to positively affect the future.
In my mind, I went through exactly the reasoning of, if we want to positively affect the future, we have to understand what's going to happen in probabilistic terms and how to think about what we can decide now and what sort of positive or negative effects will that have. To do that, you need predictions and you need probabilities. That got me thinking about, how could we generate those? What kind of system could give us the sorts of predictions and probabilities that we want? It's now grown pretty big. Metaculus now has 1,800 questions that are live on the site and 210,000 predictions on them, sort of of order of a hundred predictions per question.
The questions are all manner of things from who is going to be elected in some election to will we have a million residents on Mars by 2052, to what will the case fatality rate be for COVID-19. It spans all kinds of different things. The track record has been pretty good. Something that's unusual in the world is that you can just go on the site and see every prediction that the system has made and how it's turned out and you can score it in various ways, but you can get just a clear sense of how accurate the system has been over time. Each user also has a similar track record that you can see exactly how accurate each person has been over time. They get a reputation and then the system folds that reputation in when it's making predictions about new things.
With COVID-19, as I mentioned earlier, lots of people suddenly realized that they really wanted good predictions about things. We've had a huge influx of people and interest in the site focused on the pandemic. That suggested to us that this was something that people were really looking for and was helpful to people, so we put a bunch of effort into creating a kind of standalone subset of Metaculus called pandemic.metaculus.com that's hosting just COVID-19 and pandemic related things. That has 120 questions or so live on it now with 23,000 predictions on them. All manner of how many cases, how many deaths will there be and various things, what sort of medical interventions might turn out to be useful, when will a lock down in a certain place be lifted. Of course, all these things are unknowable.
But again, the point here is to get a best estimate of the probabilities that can be folded into planning. I also find that even when it's not a predictive thing, it's quite useful as just an information aggregator. For example, one of the really frustratingly hard to pin down things in the COVID-19 pandemic is the infection or case fatality, like what is the ratio of fatalities to the total number of identified cases or symptomatic cases or infections. Those really are all over the place. There's a lot of controversy right now about whether that's more like 2% or more like 0.2% or even less. There are people advocating views like that. It's a little bit surprising that it's so hard to pin down, but that's all tied up in the prevalence of testing and asymptomatic cases and all these sorts of things.
Even a way to have a sort of central aggregation place for people to discuss and compare and argue about and then make numerical estimates of this rate, even if it's less a prediction, right, because this is something that exists now, there is some value of this ratio, so even something like that, having people come together and have a specific way to put in their numbers and compare and combine those numbers I think is a really useful service.
Lucas Perry: Can you say a little bit more about the efficacy of the predictions? Like for example, I think that you mentioned that Metaculus predicted COVID-19 at a 10% probability?
Anthony Aguirre: Well, somewhat amusingly, somewhat tragically, I guess, there was a series of questions on Metaculus about pandemics in general long before this one happened. In December, one of those questions closed, that is no more predictions were made on it, and that question was, will there be a naturally spawned pandemic leading to at least a hundred million reported infections or at least 10 million deaths in a 12 month period by the end of 2025? The probability that was given to that was 36% on Metaculus. It's a surprisingly high number. We now know that that was more like 100% but of course we didn't know that at the time, but I think that was a much higher number than a fair number of people would have given it and certainly a much higher number than we were taking into account in our decisions. If anyone in a position of power had really believed that there were 36% chance of that happening, that would have led, as we discussed earlier, to a lot different actions taken. So that's one particular question that I found interesting, but I think the more interesting thing really is to look across a very large number of questions and how accurate the system is overall. And then again, to have a way to say that there's a meaning to the probabilities that are generated by the system, even for things that are only going to happen once and never again.
Like there's just one time that chloroquine is either going to work or not work. We're going to discover that it does or that it doesn't. Nonetheless, we can usefully take probabilities from the system predicting it, that are more useful than probabilities you're going to get through almost any other way. If you ask most doctors what's the probability that chloroquine is going to turn out to be useful? They'll say, "Well we don't know. Let's do the clinical trials" and that's a perfectly good answer. That's true. We don't know. But if you wanted to make a decision in terms of resource allocation say, you really want to know how is it looking, what's the probability of that versus some other possible things that I might put resources into. Now in this case, I think we should just put resources into all of them if we possibly can because it's so important that it makes sense to try everything.
But you can imagine lots of cases where there would be a finite set of resources and even in this case there is a finite set of resources. You might want to think about where are the highest probability things and you'd want numbers ideally associated with those things. And so that's the hope is to help provide those numbers and more clarity of thinking about how to make decisions based on those numbers.
Lucas Perry: Are there things like Metaculus for experts?
Anthony Aguirre: Well, I would say that it is already for experts in that we certainly encourage people with subject matter expertise to be involved and often they are. There are lots of people who have training in infectious disease and so on that are on pandemic.metaculus and I think hopefully that expertise will manifest itself in being right. Though as I said, you could be very expert in something but pretty bad at making predictions on it and vice versa.
So I think there's already a fairly high level of expertise, and I should plug this for the listeners. If you like making or reading predictions and having in depth discussions and getting into the weeds about the numbers. Definitely check this out. Metaculus could use more people making predictions and making discussion on it. And I would also say we've been working very hard to make it useful for people who want accurate predictions about things. So we really want this to be helpful and useful to people and if there are things that you'd like to see on it, questions you'd like to have answered, capabilities whatever. The system is there, ask for those, give us feedback and so on. So yeah, I think Metaculus is already aimed at being a system that experts in a given topic would use but it doesn't base its weightings on expertise.
We might fold this in at some point if it proves useful, it doesn't at the moment say, oh you've got a PhD in this so I'm going to triple the weight that I give to your prediction. It doesn't do that. Your PhD should hopefully manifest itself as being right and then that would give you extra weight. That's less useful though in something that is brand new. Like when we have lots of new people coming in and making predictions. It might be useful to fold in some weighting according to what their credentials or expertise are or creating some other systems where they can exhibit that on the system. Like say, "Here I am, I'm such and such an expert. Here's my model. Here are the details, here's the published paper. This is why you should believe me". That might influence other people to believe their prediction more and use it to inform their prediction and therefore could end up having a lot of weight. We're thinking about systems like that. That could add to just the pure reputation based system we have now.
Lucas Perry: All right. Let's talk about this from a higher level. From the view of people who are interested and work in global catastrophic and existential risks and the kinds of broader lessons that we're able to extract from COVID-19. For example, from the perspective of existential risk minded people, we can appreciate how disruptive COVID-19 is to human systems like the economy and the healthcare system, but it's not a tail risk and its severity is quite low. The case fatality rate is somewhere around a percent plus or minus 0.8% or so and it's just completely shutting down economies. So it almost makes one feel worse and more worried about something which is just a little bit more deadly or a little bit more contagious. The lesson or framing on this is the lesson of the fragility of human systems and how the world is dangerous and that we lack resilience.
Emilia Javorsky: I think it comes back to part of the conversation on a combination of how we make decisions and how decisions are made as a society being one part, looking at information and assessing that information and the other part of it being experience. And past experience really does steer how we think about attacking certain problem spaces. We have had near misses but we've gone through quite a long period of time where we haven't had anything this in the case of pandemic or we can think of other categories of risk as well that's been sufficient to disturb society in this way. And I think that there is some silver lining here that people now acutely understand the fragility of the system that we live in and how something like the COVID-19 pandemic can have such profound levels of disruption. Where on the spectrum of the types of risks that we're assessing and talking about. This would be on the more milder end of the spectrum.
And so I do think that there is an opportunity potentially here where people now unfortunately have had the experience of seeing how severely life can be disrupted, and how quickly our systems break down, and that absence of fail-safes and sort of resilience baked into them to be able to deal with these sorts of things. From one perspective I can see how you would feel worse. From another perspective I definitely think there's a conversation to have. And start to take seriously some of the other risks that fall into the category of being catastrophic on a global scale and not entirely remote in terms of their probabilities. Now that people are really listening and paying attention.
Anthony Aguirre: The risk of a pandemic has probably been going up with population density and people pushing into animals habitats and so on, but not maybe dramatically increasing with time. Whereas there are other things like a deliberately or accidentally human caused pandemic where people have deliberately taken a pathogen and made it more dangerous in one way or another. And there are risks, for example, in synthetic biology where things that would never have occurred naturally can be designed by people. These are risks and possibilities that I think are growing very, very rapidly because the technology is growing so rapidly and may therefore be very, very underestimated when we're basing our risks on frequencies of things happening in the past. This really gets worse the more you think about it because the idea that a naturally occurring thing could be so devastating and that when you talk to people in infectious disease about what in principle could be made, there are all kinds of nasty properties of different pathogens that if combined would be something really, really terrible and nature wouldn't necessarily combine them like that. There's no particular reason to, but humans could.
Then you really open up really, really terrifying scenarios. I think this does really drive home in an intuitive, very visceral way that we're not somehow magically immune to those things happening and that there isn't necessarily some amazing system in place that's just going to prevent or stop those things from happening if those things get out into the world. We've seen containment fail, what this lesson tells us that we should be doing and what we should be paying more attention to. And I think it's something we really, really urgently need to discuss.
Emilia Javorsky: So much of the cultural psyche that we've had around these types of risks has focused so much primarily on bad actors. When we talk about the risks that arise from pandemics, tools like genetic engineering and synthetic biology. We hear a lot about bad actors and the risks of bio-terrorism, but what you're discussing, and I think really rightly highlighting, is that there doesn't have to be any sort of ill will baked into these kinds of risks for them to occur. There can just be sloppy science that's part of this or science with inadequate safety engineering. I think that that's something people are starting to appreciate now that we're experiencing a naturally occurring pandemic where there's no actor to point to. There's no ill will, there's no enemy so to speak. Which is how I think so much of the pandemic conversation has happened up until this point and other risks as well where everyone assumes that it's some sort of ill will.
When we talk about nuclear risk, people think about generally the risk of a nuclear war starting. Well we know that the risk of nuclear war versus the risk of nuclear accident, those two things are very different and its accidental risk that is much more likely to be devastating than purposeful initiation of some global nuclear war. So I think that's important too, is just getting an appreciation that these things can happen either naturally occurring or when we think about emerging technologies, just a failure to understand and appreciate and engage in the precautions and safety measures that are needed when dealing with largely unknown science.
Anthony Aguirre: I completely agree with you, while also worrying a little bit that our human tendency is to react more strongly against things that we see as deliberate. If you look at just the numbers of people that have died of terrorist attacks say, they're tiny compared to many, many other causes. And yet we feel as a society very threatened and have spent incredible amounts of energy and resources protecting ourselves against those sorts of attacks. So there's some way in which we tend to take much more seriously for some reason, problems and attacks that are willful and where we can identify a wrongdoer, an enemy.
So I'm not sure what to think. I totally agree with you that there are lots of problems that won't have an enemy to be fighting against. Maybe I'm agreeing with you that I worry that we're not going to take them seriously for that reason. So I wonder in terms of pandemic preparedness, whether we shouldn't keep emphasizing that there are bad actors that could cause these things just because people might pay more attention to that, whereas they seem to be awfully dismissive of the natural ones. I'm not sure how to think about that.
Emilia Javorsky: I actually think I'm in complete agreement with you, Anthony, that my point is coming from perhaps misplaced optimism that this could be an inflection point in that kind of thinking.
Anthony Aguirre: Fair enough.
Lucas Perry: I think that what we like to do is actually just declare war on everything, at least in America. So maybe we'll have to declare a war on pathogens or something and then people will have an enemy to fight against. So continuing here on trying to consider what lessons the coronavirus situation can teach us about global catastrophic and existential risks. We have an episode with Toby Ord coming out tomorrow, at the time of this recording. In that conversation, global catastrophic risk was defined as something which kills 10% of the global population. Coronavirus is definitely not going to do that via its direct effects nor its indirect effects. There are real risks and a real class of risks which are far more deadly and widely impacting than COVID-19 and one of these that I'd like to pivot into now is what you guys just mentioned briefly was the risk of synthetic bio.
So that would be like AI enabled synthetic biology. So pathogens or viruses which are constructed and edited in labs via new kinds of biotechnology. Could you explain this risk and how it may be a much greater risk in the 21st century than naturally occurring pandemics?
Emilia Javorsky: I think what I would separate out is thinking about synthetic biology vs genetic engineering. So there are definitely tools we can use to intervene in pathogens that we already know and exist and one can foresee and thinking down sort of the bad actor train of thought, how you could intervene in those to increase their lethality, increase their transmissibility. The other side of this that's a more unexplored side and you alluded to it being sort of AI enabled. It can be enabled by AI, it can be enabled by human intelligence, which is the idea of synthetic biology and creating life forms, sort of nucleotide by nucleotide. So we now have that capacity to really design DNA, to design life in ways that we previously just did not have that capacity to do. There's certainly a pathogen angle that, but there's also a tremendously unknown element.
We could end up creating life forms that are not things that we would intuitively think of as sort of human designers of life. And so what are the certain risks that are posed by potential entirely new classes of pathogens that we have not yet encountered before? When we talk about tools for either intervening and pathogens that already exist and changing their characteristics or creating designer ones from scratch, is just how cheap and ubiquitous these technologies have become. They're far more accessible in terms of how cheap they are, how available they are and the level of expertise required to work with them. There's that aspect of being a highly accessible, dangerous technology that also changes how we think about that.
Anthony Aguirre: Unfortunately, it seems not hard for me or I think anyone, but unfortunately not also for the biologists that I've talked to, to imagine pathogens that are just categorically worse than the sorts of things that have happened naturally. With AIDS, HIV, it took us decades and we still don't have a vaccine and that's something that was able to spread quite widely before anyone even noticed that it existed. So you can imagine awful combinations of long asymptomatic transmission combined with terrible consequences and difficulty of any kind of countermeasures being deliberately combined into something that just would be really, really orders of magnitude more terrible in the things we've experienced. It's hard to imagine why someone would do that, but there are lots of things that are hard to imagine that people nonetheless do unfortunately. I think everyone whose thought much about this agrees that it's just a huge problem, potentially the sort of super pathogen that could in principle wipe out a significant fraction of the world's population.
What is the cost associated with that? The value of the world is hard to even know how to calculate it. It is just a vast number.
Lucas Perry: Plus the deep future.
Emilia Javorsky: Right.
Anthony Aguirre: I suppose there's a 0.01% chance of someone developing something like that in the next 20 years and deploying it. That's a really tiny chance, probably not going to happen, but when you multiply it by quadrillions of dollars, that still merits a fairly large response because it's a huge expected cost. So we should not be putting thousands or hundreds of thousands or even millions of dollars into worrying about that. We really should be putting billions of dollars into worrying about that, if we were running the numbers even within an order of magnitude correctly. So I think that's an example where our response to a low probability, high impact threat is utterly, utterly tiny compared to where it should be. And there are some other examples, but that's one of those ones where I think it would be hard to find someone who would say that that isn't 0.1 or even 1% likely over the next 20 years.
But if you really take that seriously, we should be doing a ton about this and we're just not. Looking at many such examples and there are not a huge number, but there are enough that it takes a fair amount of work to look at them. And that's part of what the future of Life Institute is here to do. And I'm looking forward to hearing your interview with Toby Ord as well along those lines. We really should be taking those things more seriously as a society and we don't have to put in the right amount of money in the sense that if it's 1% likely we don't have to put in 1% of a quadrillion dollars because fortunately it's way, way cheaper to prevent these things than to actually deal with them. But at some level, money should be no object when it comes to making sure that our entire civilization doesn't get wiped out.
We can take as a lesson from this current pandemic that terrible things do happen even if nobody wants them to or almost nobody wants them to, they can easily outstrip our ability to deal with them after they've happened, particularly if we haven't correctly planned for them. But that we are at a place in the world history where we can see them potentially coming and do something about it. I do think when we're stuck at home thinking about in this terrible case scenario, 1% or even a few percent of our citizens could be killed by this disease. And I think back to what it must've been like in the middle ages when a third of Europe was destroyed by the Black Death and they had no idea what was going on. Imagine how terrifying that was and as bad as it is now, we're not in that situation. We know exactly what's going on at some level. We know what we can do to prevent it and there's no reason why we shouldn't be doing that.
Emilia Javorsky: Something that keeps me up at night about these scenarios is that prevention is really the only key strategy that has a good shot at being effective because we see how much, and I take your HIV example as being a great one, of how long it takes us to even to begin to understand the consequences of a new pathogen on the human body and nevermind to figure out how to intervene. We are at the infancy of our understanding about human physiology and even more so in how do we intervene in it. And when you see the strategies that are happening today with vaccine development, we still know about approximately how long that takes. A lot of that's driven by the need for clinical studies. We don't have good models to predict how things perform in people. That's on the vaccine side, It's also on the therapeutic side.
This is why clinical trials are long and expensive and still fail quite late stage. Even when we get to the point of knowing that something works in a Petri dish and then a mouse and then an early pilot study. At a phase three clinical study, that drug can fail its efficacy endpoint. And that's quite common and that's part of what drives up the cost of drug development. And so from my perspective, having come from the human biology side, it just strikes me given where medical knowledge is and the rate at which it's progressing, which is quick, but it's not revolutionary and it's dwarfed by the rate of progress in some of these other domains, be it AI or synthetic biology. And so I'm just not confident that our field will move fast enough to be able to deal with an entirely novel pathogen if it comes 10, 20 even 50 years down the road. Personally what motivates me and gets me really passionate is thinking about these issues and mitigation strategies today because I think that is the best place for our efforts at the moment.
Anthony Aguirre: One thing that's encouraging I would say about the COVID-19 pandemic is seeing how many people are working so quickly and so hard to do things about it. There are all kinds of components to that. There's vaccine and antivirals and then all of the things that we're seeing play out are inventions that we've devised to fight against this new pathogen. You can imagine a lot of those getting better and more effective and some of them much more effective so you can in principle, imagine really quick and easy vaccine development, that seems super hard.
But you can imagine testing if there were sort of all over the place, little DNA sequencers that could just sequence whatever pathogens are around in the air or in a person and spit out the list of things that are in there. That would seem to be just an enormous extra tool in our toolkit. You can imagine things like, and I suspect that this is coming in the current crisis because it exists in other countries and it probably will exist with us. Something where if I am tested and either have or don't have an infection, that that will go into a hopefully, but not necessarily privacy preserving and encrypted database that will then be coordinated and shared in some way with other people so that the system as a whole can assess the likelihood that the people that I've been in contact with, their risk has gone up and they might be notified, they might be told, "Oh, you should get a test this week instead of next week," or something like that.
So you can imagine the sort of huge amount of data that are gathered on people now, as part of our modern, somewhat sketchy online ecosystem being used for this purpose. I think they probably will, if we could do so in a way that we actually felt comfortable with, like if I had a system where I felt like I can share my personal health data and feel like I've got trust in the system to respect my privacy and my interest, and to be a good fiduciary, like a doctor would, and keeping my interest paramount. Of course I'd be happy to share that information, and in return get useful information from the system.
So I think lots of people would want to buy into that, if they trusted the system. We've unfortunately gotten to this place where nobody trusts anything. They use it, even though they don't trust it, but nobody actually trusts much of anything. But you can imagine having a trusted system like that, which would be incredibly useful for this sort of thing. So I'm curious what you see as the competition between these dangers and the new components of the human immune system.
Emilia Javorsky: I am largely in agreement that on the very short term, we have technologies available today. The system you just described is one of them that can deal with this issue of data, and understanding who, what, when where are these symptoms and these infections. And we can make so much smarter decisions as a society, and really have prevented a lot of what we're seeing today, if such a system was in place. That system could be enabled by the technology we have today. I mean, it's not a far reach to think that that would be out of grasp or require any kind of advances in science and technology to put in place. They require perhaps maybe advances in trust in society, but that's not a technology problem. I do think that's something that there will be a will to do after the dust settles on this particular pandemic.
I think where I'm most concerned is actually our short term future, because some of the technologies we're talking about, genetic engineering, synthetic biology, will ultimately also be able to be harnessed to be mitigation strategies for the kinds of things that we will face in the future. What I guess I'm worried about is this gap between when we've advanced these technologies to a place that we're confident that they're safe and effective in people, and we have the models and robust clinical data in place to feel comfortable using them, versus how quickly the threat is advancing.
So I think in my vision towards the longer term future, maybe on the 100 year horizon, which is still relatively very short, beyond that I think there could be a balance between the risks and the ability to harness these technologies to actually combat those risks. I think in the shorter term future, to me there's a gap between the rate at which the risk is increasing because of the increased availability and ubiquity of these tools, versus our understanding of the human body and ability to harness these technologies against those risks.
So for me, I think there's total agreement that there's things we can do today based on data and tesingt, and rapid diagnostics. We talk a lot about wearables and how those could be used to monitor biometric data to detect these things before people become symptomatic, those are all strategies we can do today. I think there's longer term strategies of how we harness these new tools in biology to be able to be risk mitigators. I think there's a gap in between there where the risk is very high and the tools that we have that are scalable and ready to go are still quite limited.
Lucas Perry: Right, so there's a duality here where AI and big data can both be applied to helping mitigate the current threats and risks of this pandemic, but also future pandemics. Yet, the same technology can also be applied for speeding up the development of potentially antagonistic synthetic biology, organisms which bad actors or people who are deeply misanthropic, or countries wish to gain power and hold the world hostage, may be able to use to realize a global catastrophic or existential risk.
Emilia Javorsky: Yeah, I mean, I think AI's part of it, but I also think that there's a whole category of risk here that's probably even more likely in the short term, which is just the risks introduced by human level intelligence with these pathogens. That knowledge exists of how to make things more lethal and more transmissible with the technology available today. So I would say both.
Lucas Perry: Okay, thanks for that clarification. So there's clearly a lot of risks in the 21st Century from synthetic bio gone wrong, or used for nefarious purposes. What are some ways in which synthetic bio might be able to help us with pandemic preparedness, or to help protect us against bad actors?
Emilia Javorsky: When we think about the tools that are available to us today within the realm of biotechnology, so I would include genetic engineering and synthetic biology in that category. The upside is actually tremendously positive. Where we see the future for these tools, the benefits have the potential to far outweigh the risks. When we talk about using these tools, these are the same tools, very similar to when we think about developing more powerful AI systems that are very fundamental and able to solve many problems. So when you start to be able to intervene in really fundamental biology, that really unlocks the potential to treat so many of the diseases that lack good treatments today, and that are largely incurable.
But beyond that, they can take that a step further, and being able to increase our health spans and our life spans. Even more broadly than that, really are key to some of the things we think about as existential risks and existential hope for our species. Today we are talking in depth about pandemics and the role that biology can play as a risk factor. But those same tools can be harnessed. We're seeing it now with more rapid vaccine development, but things like synthetic biology and genetic engineering, are fundamental leaps forward in being able to protect ourselves against these threats with new mitigation strategies, and making our own biology and immune systems more resilient to these types of threats.
That ability for us to really now engineer and intervene in human biology, and thinking towards the medium to longterm future, unlocks a lot of possibilities for us, beyond just being able to treat and cure diseases. We think about how our own planet and climate is evolving, and we can use these same tools to evolve with it, and evolve to be more tolerant to some of the challenges that lie ahead. We all kind of know that eventually, whether that eventual will be sooner or much later, the survival of our species is contingent on becoming multi planetary. When we think about enduring the kind of stressors that even near term space travel impose and living in alien environments and adapting to alien environments, these are the fundamental tools that will really enable us to do that.
Well today, we're starting to see the downsides of biology and some of the limitations of the tools we have today to intervene, and understanding what some of the near term risks are that the science of today poses in terms of pandemics. But really the future here is very, very bright for how these tools can be used to mitigate risk in the future, but also take us forward.
Lucas Perry:You have me thinking here about a great Carl Sagan quote that I really like where he says, "It will not be who reach Alpha Centauri and the other nearby stars, it will be a species very like us, but with more of our strengths and fewer of our weaknesses." So, yeah, that seems to be in line with the upsides of synthetic bio.
Emilia Javorsky: You could even see the foundations of how we could use the tools that we have today to start to get to Proxima B. I think that quote would be realized in hopefully the not too distant future.
Lucas Perry: All right. So, taking another step back here, let's get a little bit more perspective again on extracting some more lessons.
Anthony Aguirre: There were countries that were prepared for this and acted fairly quickly, and efficaciously, partly because they maybe had more firsthand experience with the previous perspective pandemics, but also maybe they just had a slightly different constituted society and leadership structure. There's a danger here, I think, of seeing that top down and authoritarian governments have seen to be potentially more effective in dealing with this, because they can just take quick action. They don't have to do a bunch of red tape or worry about pesky citizen's rights and things, and they can just do what they want and crush the virus.
I don't think that's entirely accurate, but to the degree that it is, or that people perceive it to be, that worries me a little bit, because I really do strongly favor open societies and western democratic institutions over more totalitarian ones. I do worry that when our society and system of government so abjectly fails in serving its people, that people will turn to something rather different, or become very tolerant of something rather different, and that's really bad news for us, I think.
So that worries me, a kind of competition of forms of government level that I really would like to see a better version of ours making itself seen and being effective in something like this, and sort of proving that there isn't necessarily a conflict between having a right conferring, open society, with a strong voice of the people, and having something that is competent and serves its people well, and is capable in a crisis. They should not be mutually exclusive, and if we make them so, then we do so at great peril, I think.
Emilia Javorsky: That same worry keeps me up at night. I'll try an offer an optimistic take on it.
Anthony Aguirre: Please.
Emilia Javorsky: Which is that authoritarian regimes are also the type that are not noted for their openness, and their transparency, and their ability to share realtime data on what's happening within their borders. And so I think when we think about this pandemic or global catastrophic risk more broadly, the we is inherently the global community. That's the nature of a global catastrophic risk. I think part of what has happened in this particular pandemic is it hit in the time where the spirit of multilateralism and global cooperation is arguably, in modern memory, partially the weakest its been. And so I think that the other way to look at it is, how do we cultivate systems of government that are capable of working together and acting on a global scale, and understanding that pandemics and global catastrophic risk is not confined to national borders. And how do you develop the data sharing, the information sharing, and also the ability to respond to that data in realtime at a global scale?
The strongest argument for forms of government that comes out of this is a pivot towards one that is much more open, transparent, and cooperative than perhaps we've been seeing as of late.
Anthony Aguirre: Well, I hope that is the lesson that's taken. I really do.
Emilia Javorsky: I hope so, too. That's the best perspective I can offer on it, because I too, am a fan of democracy and human rights. I believe these are generally good things.
Lucas Perry: So wrapping things up here, let's try to get some perspective and synthesis of everything that we've learned from the COVID-19 crisis and what we can do in the future, what we've learned about humanity's weaknesses and strengths. So, if you were to have a short pitch each to world leaders about lessons from COVID-19, what would that be? We can start with Anthony.
Anthony Aguirre: This crisis has thrust a lot of leaders and policy makers into the situation where they're realizing that they have really high stakes decisions to make, and simply not the information that they need to make them well. They don't have the expertise on hand. They don't have solid predictions and modeling on hand. They don't have the tools to fold those things together to understand what the results of their decisions will be and make the best decision.
So I think, I would suggest strongly that policy makers put in place those sorts of systems, how am I going to get reliable information from experts that allows me to understand from them, and model what is going to happen given different choices that I could make and make really good decisions so that when a crisis like this hits, we don't find ourselves in the situation of simply not having the tools at our disposal to handle the crisis. And then I'd say having put those things in place, don't wait for a crisis to use them. Just use those things all the time and make good decisions for society based on technology and expertise and understanding that we now are able to put in place together as a society, rather than whatever decision making processes we've generated socially and historically and so on. We actually can do a lot better and have a really, really well run society if we do so.
Lucas Perry: All right, and Emilia?
Emilia Javorsky: Yeah, I want to echo Anthony's sentiment there with the need for evidence based realtime data at scale. That's just so critical to be able to orchestrate any kind of meaningful response. And also to be able to act as Anthony eludes to, before you get to the point of a crisis, because there was a lot of early indicators here that could have prevented this situation that we're in today. I would add that the next step in that process is also developing mechanisms to be able to respond in realtime at a global scale, and I think we are so caught up in sort of moments of an us verse them, whether that be on a domestic or international level, but the spirit of multilateralism is just at an all-time low.
I think we've been sorely reminded that when there's global level threats, they require a global level response. No matter how much people want to be insular and think that their countries have borders, the fact of the matter is is that they do not. And we're seeing the interdependency of our global system. So I think that in addition to building those data structures to get information to policy makers, there also needs to be a sort of supply chain and infrastructure built, and decision making structure to be able to respond to that information in real time.
Lucas Perry: You mentioned information here. One of the things that you did want to talk about on the podcast was information problems and how information is currently extremely partisan.
Emilia Javorsky: It's less so that it's partisan, and more so that it's siloed and biased and personalized. I think one aspect of information that's been very difficult in this current information environment, is the ability to communicate to a large audience accurate information, because the way that we communicate information today is mainly through click bait style titles. When people are mainly consuming information in a digital format, and it's highly personalized, it's highly tailored to their preferences, both in terms of the news outlets that they innately turn to for information, but also their own personal algorithms that know what kind of news to show you, whether it be in your social feeds or what have you.
I think when the structure of how we disseminate information is so personalized and partisan, it becomes very difficult to bring through all of that noise to communicate to people accurate balanced, measured, information. Because even when you do, it's human nature that that's not the types of things people are innately going to seek out. So what in times like this are mechanisms of disseminating information that we can think about that supersede all of that individualized media, and really get through to say, "All right, everyone needs to be on the same page and be operating off the best state of information that we have at this point. And this is what that is."
Lucas Perry: All right, wonderful. I think that helps to more fully unpack this data structure point that Anthony and you were making. So yeah, thank you both so much for your time, and for helping us to reflect on lessons from COVID-19.