James Manyika on Global Economic and Technological Trends
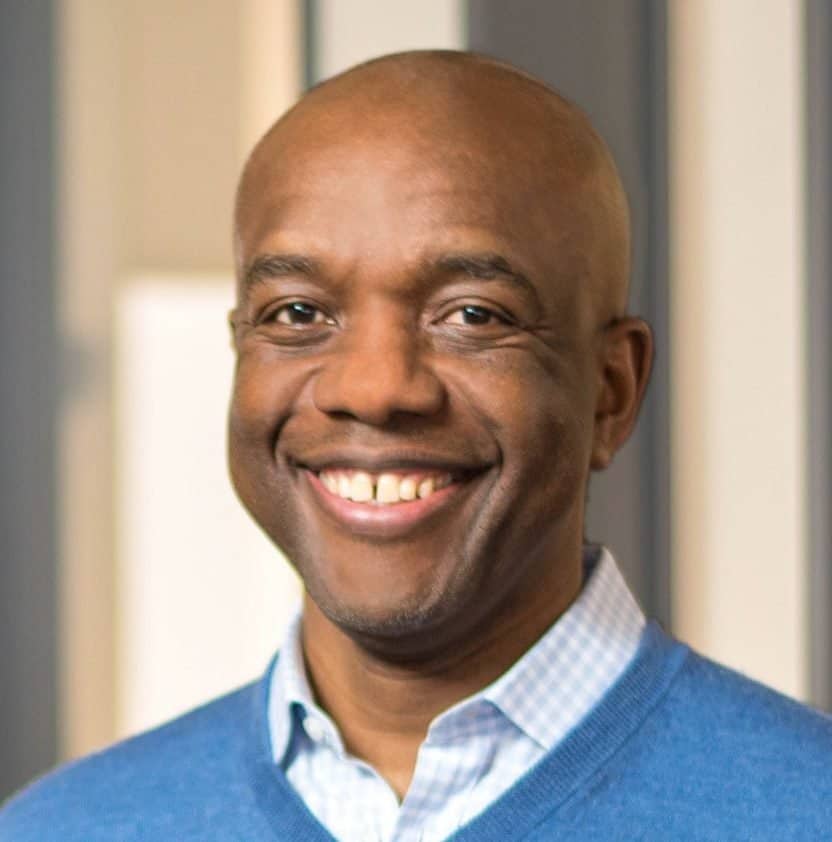
James Manyika, Chairman and Director of the McKinsey Global Institute, joins us to discuss the rapidly evolving landscape of the modern global economy and the role of technology in it.
- The modern social contract
- Reskilling, wage stagnation, and inequality
- Technology induced unemployment
- The structure of the global economy
- The geographic concentration of economic growth
Watch the video version of this episode here
29:28 How does AI automation affect the virtuous and vicious versions of productivity growth?
38:06 Automation and reflecting on jobs lost, jobs gained, and jobs changed
43:15 AGI and automation
48:00 How do we address the issue of technology induced unemployment
58:05 Developing countries and economies
1:01:29Â The central forces in the global economy
1:07:36 The global economic center of gravity
1:09:42 Understanding the core impacts of AI
1:12:32 How do global catastrophic and existential risks fit into the modern global economy?
1:17:52 The economics of climate change and AI risk
1:20:50 Will we use AI technology like we've used fossil fuel technology?
1:24:34 The risks of AI contributing to inequality and bias
1:31:45 How do we integrate developing countries voices in the development and deployment of AI systems
1:33:42 James' core takeaway
1:37:19 Where to follow and learn more about James' work
Transcript
Lucas Perry: Welcome to the Future of Life Institute Podcast. I'm Lucas Perry. Today's episode is with James Manyika, and is focused on global economic and technological trends. As the Agricultural and Industrial Revolutions both led to significant shifts in human labor and welfare, so too is the ongoing Digital Revolution, driven by innovations, such as big data, AI, the digital economy, and robotics also radically affecting productivity, labor markets, and the future of work. And being in the midst of such radical change ourselves, it can be quite difficult to keep track of where we exactly are and where we're heading. While this particular episode is not centrally focused on existential risk, we feel that it's important to understand the current and projected impacts of technologies like AI, and the ongoing benefits and risks of their use to society at large, in order to increase our wisdom and understanding of what beneficial futures really consist of.
It's in the spirit of this that we explore global economic and technological trends with James Manyika in this episode. James received a PhD from Oxford in AI and robotics, mathematics, and computer science. He is a senior partner at McKinsey & Company, as well as chairman and director of McKinsey Global Institute. James advised the chief executives and founders of many of the world's leading tech companies on strategy and growth, product, and business innovation, and was also appointed by President Barack Obama to serve as vice chair of the Global Development Council at the White House. James is most recently the author of the book, No Ordinary Disruption: The Four Global Forces Breaking All the Trends. And it's with that, I'm happy to present this interview with James Manyika.
To start things off here, I'm curious if you could start by explaining what you think are some of the most important problems in the world today.
James Manyika: Well, first of all, thank you for having me. Gosh, one of the most important problems in the world, I think we have the challenge of climate change, I think we have the challenge of inequality, I think we have the challenge that economic growth and development is happening unevenly. So I should say that the inequality question, I think is most in inequality within countries, but to some extent also between countries. And this idea of uneven development is that some countries are surging ahead and some parts of the world are potentially being left behind. I think we have other social political questions, but those I'm not qualified to about those, I don't really spend my time, I'm not a sociologist or political scientist, but I think we do have some social-political challenges too.
Lucas Perry: We have climate change, we have social inequality, we have the ways in which different societies are progressing at different rates. So given these issues in the world, what do you think it is that humanity really needs to understand or get right in the century given these problems?
James Manyika: Yeah, by the way, I should also, before I dive into that, also say, even though we have these problems and challenges, we also have incredible opportunities, quite frankly, for breakthrough, progress, and prosperity to solve some of these issues. And quite frankly, do things that are going to transform humanity for the better. So these are challenges at a time of, I think, unprecedented opportunity and possibility. So I just want to make sure we acknowledge both sides of that issue. It terms of what we need to do about these challenges. I think part of it is also just quite frankly, facing them head on. I think the question of climate change is one that is an existential challenge that we just need to face head on. And quite frankly, get on with doing everything we can both to mitigate the effects of climate change, and also quite frankly, to start to adapt how our society, our economy works to, again, address what is essentially an existential challenge.
So I think what we do in the next 10 years is going to matter more than what we do in the 10 years after that. So there's some urgency to the climate change and climate risk question. I think with regards to the issue of inequality, I think this is one that is also within our capacity to address. I think it's important to keep in mind that the capitalism and the market economies that we've had, and we do have, have been unbelievably successful in creating growth and economic prosperity for the world and in most places where they've been applied. Particularly in recent years, I think, we've also started to see that in fact, there've been growth in inequality, partly because the structure of our economy is changing and we can get into that conversation.
In fact, some people are doing phenomenally well and others are not, and some places are doing phenomenally well and some other places are not. I mean, it's not lost on me, for example, Lucas, that even if you look at an economy like the United States, something like two thirds of our economic output comes out of 6% of the counties in the country. That's an inequality of place, in addition to the inequalities that we have of people. So I think we have to tackle the issue of inequality quite head-on. Unless we do something, it has the potential to get worse before it gets better. The way our economy now works, and this is quite different by the way than what it might've looked like even as recently as 25 years ago, which is, most of the economic activity, a function of the sectors that are driving economic growth, a function of the types of enterprises and companies that are driving economic growth, they have tended to be much more today than they were 25 years ago to be fairly regionally concentrated and in particular places.
So some of those places include Silicon Valley and other places. Whereas if you had looked for example, 25 years ago where you might've seen the kind of sectors and companies that were doing well were much more geographically distributed, so you had more economic activity coming out of more places across the country than you do now. So this is just, again, a function of not that anybody designed it to be that way, but just a function of the sectors and companies and the way our economy works. A related factor by the way, is even the inequality question is also a function of how the economy works. I mean, it used to be the case that whenever we had economic growth and productivity growth, it also resulted in job growth and wage growth. That's been true for a very long time, but I think in recent years, as in, depending on how you count it, the last 25 years ago or so, when we have productivity growth, it doesn't lift up wages as much as it used to. Again, it's a function of the structure of the economy.
In fact, some of the work we've been doing and other economists have been doing, is actually being to look at this so-called declining labor share. I think a way to understand that declining labor share is to think about the fact that, if you had to set up a factory say in a hundred years ago, most of the inputs into how that factory worked were all labor inputs, so the labor share of economic activity is much higher. But over time, if you're setting up a factory today, sure you have labor input, but you also have a lot of capital input in terms of the equipment, the machinery, the robots, and so forth. So the actual labor portion of, you know, of it as a share of the inputs, it's being going down steadily. And that's just part of how structure of our economy is changing. So all of these effects are some of what is leading to some of the inequality that we see.
Lucas Perry: So before we drill more deeply into climate change and inequality, are there any other issues that you would add as some of the most crucial problems or questions for the 21st century?
James Manyika: The structure of our global economy is changing, and I think it's now getting caught up also in kind of geopolitics. I'm not a geopolitical expert, but it's not lost from a global economy standpoint that, in fact, we now have and will have two very large economies, the United States and China, and China is a very large economy. And it's not just a source of exports or the things that we buy from them, but it's also entangled with say the US and other countries economically in terms of monetary, and debt, and lending, and so forth. But it's also a large economy itself, which is going to have its own consumption. So we now have for the first time, two very large global economies. And so, how that works in a geopolitical sense is one of the complications of the 21st century. So I think that's an important issue. Others who are more qualified to talk about geopolitics can delve into that one, but that's clearly in the mix as part of the challenges of the 21st century.
We also, of course, are going to have to think about the role of technology in our economies and our society. Partly because technology can be a force of massive productivity growth, innovation, and good, and all of that, but at the same time we know that many of these technologies raise new questions about privacy, about how we think about information, disinformation. So I think, you know, if you had to write the list of the questions we're going to need to navigate in the coming decades of the 21st century, it's a meaty list. Climate change is at the top of that list in my view, inequalities is on that list, and these questions of geopolitics are on that list, the role that technology is going to play is on that list, and then also some of these social questions that we now need to wrestle with, issues of social justice, not just economic justice but also social justice. So we have a pretty rich challenge list even at the same time that we have these extraordinary opportunities.
Lucas Perry: So in the realms of climate change, and inequality, and these new geopolitical situations and tensions that are arising, how do you see the role of incentives pushing these systems and problems in certain directions, and how it is that we come up with solutions to them given the power and motivational force of incentives?
James Manyika: Well, I think incentives play an important role. So take the issue of climate change, for example, I think one of the failures of our economics and economic systems is we've never quite priced carbon, and we've never quite built that into our incentive systems, our economic systems so we have a price for it. So that when we put up carbon dioxide into the atmosphere and so forth, there's no economic price for that or incentives, a set of incentives not to do that. We haven't done enough in that regard. So that's an area where incentives would actually make a big difference. In the case of inequality, I think this one's way more complicated beyond just incentives, but I'll point to something that is in the realm of incentives as regards to inequality.
So, for example, take the way we were talking earlier about the importance of labor and capital in our capital inputs, I don't mean capital as in the money necessarily, but just the actual capital, equipment and machines, and so forth in our system, we've built a set of incentives, for example, that encourage companies to invest in capital infrastructure, you know, capital equipment, they can write it off for example. We encourage investments in R&D for example, and the tax incentives to do that, which is wonderful because we need that for the productivity and growth and innovation of our economy, but we haven't done anything nearly enough or equivalent to those kinds of incentives with regards to investments in human capital. So you could imagine in much more the productivity and growth and innovation of our economy, but we haven't done anything nearly enough or equivalent to that, to those kinds of incentives with regards to investments in human capital.
So you could imagine a much more concerted effort to create incentives for companies and others to invest in human capital and be able to write off investments in skilling, for example, to be able to do that at the equivalent scale to the incentives we have for other forms of investment, like in capital or in R&D. So that's an example of where we haven't done enough on the incentive front, and we should. But I think there's more to be done than just incentives for the inequality question, but those kinds of incentives would help.
I should tell you, Lucas, that one of the things that we spent the last year or so looking at is trying to understand how the social contract has evolved in the 21st century so far. So we actually looked at, for example, roughly 23 of the OECD countries, about 37 or 38 of them, but we looked at about 23 of them in detail just to understand how the social contract had evolved. And here because we're not sociologists, we looked to the social contract in really three ways, right? How people participate in the economy as workers, because that's part of, you know, when people work hard, and probably the exchange is that they get jobs, and they get income and wages and training. So people participating as workers is an important part of the social contract. People participating as consumers and households who consume products and services, and then people as savers who are kind of saving money for a future, you know, for their kids or for their future, et cetera.
And when you look at those three aspects of the social contract in the 21st century so far, it's really quite stunning. So take the worker piece of that, for example, what has happened is that in across most countries, we've actually grown jobs despite the recession, I guess the recession in 2001, but also the bigger one in 2008, we've actually grown jobs. So there're actually more jobs now than there were at this time of the 21st century. However, what has happened is that many of those jobs don't pay as well, so the wage associated with that, the picture has actually shifted quite a bit.
The other thing about what's happened with work is it's becoming a little bit more brittle in the fact that job certainty has certainly gone down, there's much more income and wage variability. So we've created more fragile jobs relatively to what we had at the start of the 21st century. So you could say, for workers, it's a mixed story, right? Job growth, yes, wage growth, not so much, job certainty and job fragility has gone up. When you look at people as consumers and households, it also paints an interesting story. And the picture you see there is the fact that, if households and consumers are consuming things like, think about, you know, buying cars or wide goods products, or electronics, basically things that are globally competed and traded, the cost of those has gone down dramatically in the 21st century. So the 21st century in that sense, at least globalization has been great because it's delivered these very cheap products and services.
But if you look at other products and services that households and consumers consume such as education, housing, healthcare, and in some places, depending which country or place you're in, transportation, those have actually gone up dramatically, far, far higher and faster than inflation, far higher and faster than wage growth. In fact, if you are in the bottom half of the social income scale, those things have come to dominate your income in terms of what you spend money on. So for those people, it hasn't worked out so well actually in terms of the social contract. And then on the savers side, people as savers are, very few people now can afford to save for the future. And one of the things that you see is that the growth of indebtedness in the 21st century so far has gone up for most people, especially the middle wage and low wage households and people, their ability to save for the future has gone down.
What's interesting is, it's not just that the levels of indebtedness have gone up, but it's the fact that the people who are indebtedness look a little bit different. They're younger, they're also, in many cases, college educated, which is different than what you might've seen 25 years ago in terms of who was indebted and what do they kind of look like?
And then finally, just to finish, the 21st century in the social contract sense, also hasn't worked out very well for women who still earn less than men, for example, and don't quite have the opportunities as much as others, as well as for people of color. It hasn't, so they still earn a lot less, employment rates are still much lower, their participation in the economy as any of these roles is also much less. So you get a picture that says, while the economy has grown and capitalism has been great, so far in the social contract sense at least, by these measures we've looked, at it hasn't worked out as well for everybody in the advanced economies. This is a picture that emerges from the 23 OECD countries that we looked at. And the United States is on the more extreme end of most of the trends I just described.
Lucas Perry: Emerging from this is a pretty complex picture, I think, of the way in which the world is changing. So you said the United States represents sort of the extreme end of this, where you can see the largest effect size in these areas, I would assume, yet it also seems like there's this picture of the Global East and South generally doing better off, like people being lifted out of poverty.
James Manyika: Yeah, it is true. So one of the wonderful things about the 21st century is in fact, close to a billion people have been lifted out of poverty in those roughly 20, 25 years, which is extraordinary, but we should be clear about where that has happened. Those billion people are mostly in China, and to some extent, India. So while we say we've lifted people out of poverty, we should be very specific about mostly where that has happened. There are parts of the world where that hasn't been the case, parts of Africa, other parts of Asia, and even parts of Latin America. So this lifting people out of poverty has been relatively concentrated in China primarily, and to some extent in India.
One of the things about economics, and this is something that people like Bob Solow and others got Nobel Prizes for, if you think about what is it that drives our economic growth, if economic growth is the way we create economic surpluses, that we can then all enjoy and lead to prosperity, right? The growth desegregation models come down to two things: either you're expanding labor supply, or you are driving productivity. And the two of those when they work well, combine to give you economic GDP growth.
So if you look, for example, at the last 50 years, both across the advanced economies, but even for the United States, the picture that you see is that much of our economic growth has come roughly, so far at least, has come roughly in equal measure from two things: one, you know, this is over the last 50 or so years, half of it has come from expansions in labor supply. You can think about it as a Baby Boomer Generation, more people entering the workforce, et cetera, et cetera. The other half has roughly come from productivity growth. And the two of them have combined to give us roughly the economic GDP growth that we've had.
Now, when you look forward from where we are, we're not likely to get much lift from the labor supply part of it, partly because most advanced economies are aging. And so, the contribution that's going to come from expansions in labor supply, much less. I mean, you can think of it as kind of a plane flying on two engines, right? If one engine has been expansions in labor supply and the other is in productivity, well, the labour supply engine is kind of dying out to or slowing down in its output.
We're going to rely a lot more on productivity growth. And so, where does productivity growth come from? Well, productivity growth comes from things like technological innovation, innovating how we do things and how we create products and services and all of that. And technology is a big part of that. But guess what happens? One of the things that happens with productivity growth is that the role of technology goes up. So I come back to my example of the factory. So if you wanted a highly productive factory, it's likely that your mix of labor inputs and capital inputs, read that as machinery and equipment, is going to change. And that's why your factory a hundred years ago, it looks very different than a factory today. But we need that kind of technological innovation and productivity to drive it the output. And then the output leads to the output in the sector, and ultimately the output in the economy.
So all of that is to say, I don't think we should stop the technological innovation that leads to productivity, we need productivity growth. In fact, going forward, we're going to need productivity growth even more. The question is, how do we make sure that even as we're pursuing that productivity growth that contributes to economic growth, we're also paying attention to how we mitigate or address the impacts on labor and work, which is where most people derive their livelihoods.
So, I don't think you want to set up a set of system of incentives that slows down the technological innovation and product activity growth, because otherwise, we're all going to be fighting over a diminishing economic pie. I think you want to invest in that and continue to drive that, but at the same time find ways and think about how to address some of the work implications of that, or the impacts on work and workers. And that's been one of the challenges that we've had. I mean, we've all seen the hollowing out, if you like, of the middle class in advanced economies like America, where a big part of that is that much of that middle class or middle income workers have been working in these sectors and occupations where the impact of technology and productivity have actually had a huge impact on those jobs and those incomes.
And so, even though we have work in the economy, the occupations and jobs in sectors that are growing have tended to be in the service sectors and less in places like manufacturing. I mean, it's the reason why I love it when politicians talk about good manufacturing jobs. I mean, they have a point in the sense that historically, those have been good, well paying jobs, but manufacturing today is only what? 8% of the labor force in America, right? It's diminished, at its peak is probably at best close to the mid forties, 40% as a share of the labor markets back in the '50s, right? It's being going down ever since, right? And yet the service sector economy has been growing dramatically. And many, not all, but many of the jobs in the service sector economy don't pay as much.
My point is, we have to think about not just incentives but the structure of our economy. So if you look forward, for example, over the next few decades, what of the jobs that are going to grow as a function of both demand for that work in the economy, but also as a result of what's less likely to be automated by technology and AI and so forth? You end up with a list that includes care work, for example, and so forth. And even work that we say is valuable, which it is, like teachers and others that are harder to automate. But labor market system doesn't reward and pay those occupations as much as some of the occupations that are declining. So those are some of what, when I talk about the changes in the structure of our economy, in a way that goes a little bit beyond just local incentives, is how do we address that? How do we make sure as those parts of our economy grow, which they will naturally. How do we make sure people are earning enough to live as they work in those occupations? And by the way, those occupations are many of the ones that in our current or recent COVID moment or period here, many are where the essential work and workers are by the way, people have come to rely on mostly in those service sector economies that we haven't historically paid well. Those are real challenges.
Lucas Perry: There was that really compelling figure that you gave at the beginning of our conversation, where you said 6% of counties account for two thirds of our economic output. And so, there's this change in dynamic between productivity and labor force. And the productivity you're suggesting is what is increasing, and that is related to and contingent upon AI automation technology. Is that right?
James Manyika: Well, first of all, we need productivity to increase. It's been kind of sluggish in the last several years. In fact, it's one of the key questions that economists worry about, which is, how can we increase the growth of our economic productivity? It hasn't been doing as well as we'd like it to do. So we'd like to actually increase it, partly because, as I said, we needed more than we've done in the last 50 years because of the labor supply pieces declining. So we actually would like productivity to go up even more.
Mike Spence and I just wrote a paper recently on the hopeful possibility that in fact we could see a revival in productivity growth coming out of COVID. We hope that happens, but it's not assured. So we need more productivity growth. And the way you get productivity growth, technology and innovation is a big part of it. The other part of it is just managerial innovation that happens inside companies in sectors where those companies and sectors figure out ways to organize and do what they do in innovative, but highly productive ways. So it's the combination of technology and those kinds of managerial and other innovations, usually in a competitive context, that's what drives productivity.
Lucas Perry: Does that lead to us requiring less human labor?
James Manyika: It shouldn't. One of the things about productivity is, it's actually, in some ways, labor productivity is a very simple equation, right? It has on the numerator, value-added output, divided by hours worked or labor input, if you like. So you can have, what I think of as a virtuous version of productivity growth versus a vicious one. So let me describe the virtuous one. The virtuous one, which actually leads to job growth, is when in fact you expand the numerator. So in other words, there's innovations, use of technology in the ways that I talked about before, that means to companies and sectors creating more valuable output, more of it and more valuable output. So you expand the numerator. So if you do that, and you expand the numerator much higher and faster than you're reducing the denominator, which is the labor hours worked, you end up with a virtuous cycle in the sense that the economy grows, productivity grows, everything expands, the demand for work actually goes up. And that's a virtuous cycle.
The last time we saw a great version of that was actually in the late '90s. This is, if you recall before that, Bob Solow kind of framed what ended up being called the Solow Paradox, which is this idea that before the mid and late '90s, you saw computers everywhere except in the productivity figures, right? And that's because we hadn't seen the kinds of deployment of technology, the managerial innovations do the kind of, what I call the numerator-driven productivity growth, which, when it did happen in the mid to late '90s, it created this virtuous cycle.
Now let me describe the vicious cycle, which is the, if you like, the not so great version of productivity growth. It's when you don't expand the numerator, but what you do is simply reduce the denominator. So in other words, you reduce the hours worked. In other words, you become very efficient at delivering the same output or maybe even less of the output. So you reduce the denominator, that lead to productivity, but it's off the vicious kind, right? Because you're not expanding the output, it's simply reducing the inputs, or the labor inputs. Therefore, you end up with less employment, fewer jobs, and that's not great. That's when you get what you asked about, which is, where you need less labor. And that's the vicious version of productivity, you don't want that either.
Lucas Perry: I see. How does the reality of AI and automation replacing human labor and human work essentially increasingly completely over time factor into and affect the virtuous and vicious versions of productivity?
James Manyika: Well, first of all, we shouldn't assume that AI is simply going to replace work. I think we should think about this in this context of what you might call complements and substitutes. So if our AI technology is developed and then deployed in a way that is entirely substitutive of work, then you could have work decline. But there's also other ways to deploy AI technology, where it's complementary and it complements work. And in that case, you shouldn't have to think about it as much about losing jobs.
Let me give you some specifics on this. So we've done research, and others have too, but let me describe what we've done, but I think the general consensus is emerging that it's close to what at least we found in our research, which is that, so we looked at, so the Bureau of Labor Statistics kind of tracks in the US, tracks roughly 800 plus occupations. We looked at all those occupations in the economy. We also looked at the actual particular tasks and activities that people actually do, this because any of our jobs and occupations are not monolithic, right? They're made up of several different tasks, right?
I spent part of my day typing, or talking to people, or analyzing things, so we're all an amalgam of different tasks. And we looked at over 2000 tasks that go into these different occupations, but let me get to where we ended up. So where we ended up was, we looked at what current and expected AI technology and artificial technologies can do. And we came to the conclusion that at least over the next couple of decades at the task level, and I emphasize the task level, not the job level, these are tasks, I'll come back to jobs, at the task level, these technologies look like they could automate as much as 50% of the tasks and activities that people do. And it's important to, again, emphasize those are tasks, not jobs.
Now, when you take those highly automatable tasks back and map them to the occupations in the economy, what we concluded was that something like at most 10% of the occupations look like they have all of their constituent tasks automatable. And that's a very important thing to note, right? 10% of all the occupations look like they have close to a hundred percent of their tasks that are automatable.
Lucas Perry: In what timeline?
James Manyika: This is over the next couple of decades.
Lucas Perry: Okay. Is that like two decades or?
James Manyika: We looked at this over two decades, right? We have scenarios around that because it's very hard to be precise because you can imagine the rate of technologies development speeding up, I'll come back to that, but the point is, it's only 10% of the, in our analysis anyway, 10% of the occupations look like they have all of their constituent tasks that are automatable in that rough timeframe. But at the same time, what we also found is that something like 60% of the occupations have something like a third of their constituent tasks that are automatable in that same period. Well, what does that mean? What that actually means is that many more jobs and occupations are going to change than get fully automated away. Because what happens is, sure, some activity that I used to do myself, now that activity can be done in an automated fashion, but I still do other things too, right? So this effect of kind of the jobs that will change is actually a bigger effect than the jobs that will disappear completely.
Now that's not to say there won't be any occupations that will decline. In fact, what we found in our research, and we ended up kind of titling the research report, Jobs Lost and Jobs Gained. We probably should have fully titled the Jobs Lost, Jobs Gained, and Jobs Changed because all three phenomena will happen, right? Yes, there'll be occupations that will decline, but there will also be occupations that'll grow actually. And then there'll be lots more occupations that will change. So I think we need to take the full picture into account. It's a bit like, I guess a good example of the jobs changed portion is the one of the bank teller, right? Which is, if you had looked at what a bank teller did in 1968 versus what a bank teller does now, it's very, very different, right? The bank teller back then spent all their time counting money either to take it from you or to give it back to you when you went up to the bank teller. Or the advent of ATM machines or the ATM machine automated much of that.
So we still have bank tellers today, the majority of the time isn't spent doing that, right? They may do that on an exception basis, but their jobs have changed dramatically, but there's still an occupation called a bank teller. And in fact, until about, I think the precise date is something like 2006, I think, what we actually had was a number of bank tellers in the US economy had actually grown since the early '70s to about 2006. And that's because the demand for bank tellers went up, not on a per bank basis, but on a economy-wide basis because we ended up opening up so many more branch banks by 2006 than we had in 1968. So the collective demand for banking actually drove the growth in the number of bank tellers, even though the number of bank tellers per branch might've gone down.
So that's an example of where a growing economy can create its own demand for work back to this virtuous cycle that I was talking about as opposed to the vicious cycle that I was talking about. So this phenomenon of jobs changing is an important one that often gets lost in the conversation about technology and automation and jobs. And so, to come back to your original question about substitutes, we shouldn't just think of technology substituting for jobs as the only thing that happens, but also that technology can complement work and jobs. In fact, one of the things to think about, particularly for AI researchers or people who develop these automation technologies, I think, on the one hand, while it's actually certainly useful to think of human benchmarks when we say, how do we build machines and systems that match human vision or human dexterity and so forth? That's a useful way to set goals and targets for technology development and AI development. But in an economic sense, it's actually less useful because it's less likely to lead to technologies that are more substitutes because we've built them to match what humans can do.
Imagine if we said, let's build technology machines that can see around corners or do the kinds of things that humans can't do, then we're more likely in that case to build more complementing technologies than substituted technologies. I think that's one of the things that we should be thinking about and doing a heck of a lot more to achieve.
Lucas Perry: This is very interesting. So you can think of every job as basically a list of tasks, and AI technology can automate say some number of tasks per job, but then the job changes in a sense that either you can spend more time on the tasks that remain and increase productivity by just focusing on those tasks, or the fact that AI technology is being integrated into the job process will create a few new tasks. The tension I see though is that we're headed towards a generality with AI where we're moving towards all tasks being automated. Perhaps over shorter timescales it seems like we'll be able to spend more time on fewer tasks or our jobs will change in order to meet and work on the new tasks that AI technology demands of us, but generality is a movement towards the end of human level problem solving on work and objective-related tasks. So it seems like it would be increasingly shrinking. Is that a view that you share? Does that make sense?
James Manyika: Your observation makes sense. I don't know if I fully share it, but just to back up a step, yeah, if you asked me over the next few decades, I mean, our research has looked at the next couple of decades, others have looked at this too by the way, and he'd come up with obviously slightly different numbers and views, but I think they're generally in the same direction that I just described. So if you say over the next couple of decades, what do I worry about? I certainly don't worry about the disappearance of work for sure. But that doesn't mean that all is well, right? There're still things that I worry about. So I still worry about, well, we're going to have work, because I think, you know, what we found for example is the net of jobs lost and jobs gained and jobs changed, the net of all of that in the economies that we've looked at is still a net positive in the sense that there's more work gained net then lost.
That doesn't mean we should all then be, rest in our laurels and be happy that, hey, we're not facing a jobless future. So I think we still have a few other challenges to deal with. And I want to come back to your future AGI question in a second. So one of the things to worry about, even in this stage where I say don't worry about the disappearance of work, well, there're still a few more things to worry about.
I think you want to worry about the transitions, right? The skill transitions. So if some jobs are declining, and some jobs are growing, and some jobs are changing, all of that is going to create a big requirement for skilling and reskilling, either to help people get into these new jobs that are growing, or if their jobs are changing, gain the new skills that work well alongside the task that the machines can do. So all of that says reskilling is a really big deal, which is why everybody's talking about reskilling now, though, I don't think we're doing it fast enough or at scale enough, at the scale and pace that we should be doing it. But that's one thing to worry about.
The other thing to worry about are the effects on wages. So even when you have enough work, if you look at the pattern of the jobs gained, most of them, not all of them, but many of them, many of them are actually jobs that pay less, at least in our current labor market structure, right? So care work is hard to fully automate because it turns out that, hey, it's actually harder to automate somebody doing physical mechanical tasks than say somebody doing analytical work. But it turns out the person doing analytical work, where you can probably automate what they do a lot easier, also happens to be the person who's earning a little bit more than the person doing the physical mechanical tasks. But by the way, that person is one that we don't pay much in the first place. So you end up with physical mechanical activities that are hard to automate also growing and being demanded, but then we don't pay much for them.
So the wage effects are something to worry about. Even in the example I gave you of complementing work, that's great from the point of view of people and machines working alongside each other, but even that has interesting wage effects too, right? Because at one end, which I'll call the happy end, and I'll come back to the challenged end, the happy end is when we automate some of what you do, Lucas, and the combination of what the machine now does for you and what you still do yourself as a human, both are highly valuable, so the combo is even more productive. And this is the example that's often given with the classic story of radiologists, right? So machines can maybe read some of those images way better than the radiologist, but that's not all the radiologist does, there's a whole other value-added activities and tasks that the radiologist does that the machine reading doesn't understand them, MRI doesn't do. But now you've got a radiologist partnered up with a machine, the combination is great. So that's a happy example. Probably the productivity goes up, the wages of that radiologist go up. That's a happy story.
Let me describe the less happy end of that complementing story. The less happy end of that is when the machine automates a portion of your work, but the portion that it automates is actually the value-added portion of that work. And what's left over is even more commoditized, commoditized in the sense that many, many, many more people can do it, and therefore, the skill requirements for that actually go down as opposed to go up, because the hard part of what you used to do is now being done by a machine. The danger with that is that, that then potentially depresses the wages for that work given the way you're complementing. So even the complementing story I described earlier, isn't always in one direction from a wage effect and its impact.
So all of that step back is to say, if the first thing is reskilling, the second thing to worry about are these wage effects. And then the final thing to worry about, how we think about redesigning work itself and the workflows themselves. So all of that is to say, even in a world where we have enough work, that's in the next few decades, we still are going to have to work these issues. Now, you are posing a question about, what about in the long, long future, because I should think it's in the long future that we're going to have AGI. I'm not one who thinks it's as imminent as perhaps others think.
Lucas Perry: Do you have a timeline you'd be willing to share?
James Manyika: No, I don't have a timeline, I just think that there're many, many hard problems that we still seem like a long way from... Now, the reason I don't have a timeline, is that, hey, we could have a breakthrough happen in the next decade that changes the timeline. So we haven't figured out how to do causal reasoning, we haven't figured out how to do what Kahneman called System 2 activities. We've solved System 1 tasks where we assisted... And so, there's a whole bunch of things that, you know, we haven't solved the issues of how we do a higher-level cognition or meta-level cognition, we haven't solved through how we do meta learning, transfer learning. So there's a whole bunch of things that we haven't quite solved. Now we're making progress on some of those things. I mean, some of the things that have happened with these large language universal models is really breathtaking, right?
But I think that, in my view, at least the collection of things that we have to solve before we get to AGI, there's too many that still feel unsolved to me. Now we could have somebody breakthrough in a day. That's why I'm not ready to give a prediction in terms of timeline, but these seem like really hard problems to me. And many of my friends who are working on some of these issues also seem to think these are hard problems. Although there are some of them who think that we're almost there, that all we need to, you know, deep learning will get us to most places we need to get to and reinforcement learning will get us most of what we need. So those are my friends who think that, think that it's more imminent-
Lucas Perry: In a decade or two away, sometimes they say.
James Manyika: Yeah, some of them say a decade or two. There's a lot of real debate about this. In fact, you may have seen one of the things that I participated in a couple of years ago was, and Martin Ford put together a book that was a collection of interviews with a bunch of people, it's his book, Architects of Intelligence. A wonderful range of people in that book, I was fortunate enough to be included, but there are many more people and way more interesting than me. People like Demis Hassabis and Yoshua Bengio and a whole bunch of people, it's a really terrific collection. And one of the things that he asked that group who are in that book was to ask them to give a view as to when they think AGI would be achieved. And what came out of it is a very wide range from 2029, and I think that was Ray Kurzweil who stuck to his date, and all the way to something like 500 years from now. And that's a group of people who are deep in the field, right? And you'd get that very wide range.
So I think, for me, I'm much more interested in the real things that we are going to need to break through, and I don't know when we'll make those breakthroughs, it could be imminent, it could be a long time from now, but they just seem to be some really hard problems to solve. But if you take the view, to follow your thought, if you take the view, you know, the thought experiment to say, okay, let's just assume we'll truly achieve AGI in all its sense, in both in the AGI and the, some people will say in the oracular.
I mean, it depends what form of the AGI it takes. If the AGI takes the form of both the cognitive part of that coupled with the embodiment of that of physical machines that can physically participate, and you truly have AGI in a fully-embodied sense as well in addition to the cognitive sense, what happens to humans and work in that case? I don't know. I think that's where presumably those machines allow us to create enormous surpluses and bounties in an economic sense. So presumably, we can afford to pay everybody, you know, to give everybody money and resources. And so, then the question is, in a world of true abundance, because presumably they'll help us solve these, you know, these machines, AGIs will help us solve those things, in a world of true abundance, what do people do in that?
I guess it's kind of akin, as somebody said, to Star Trek economy. What do people do in a Star Trek economy when they can replicate and do everything, right? I don't know. I guess we explore the universe, we do creative things, I don't know. I'm sure we'll create some economic system that takes advantage of the things that people can still uniquely do even though they'll probably have a very different economic value and purpose. I think humans will always find a way to create either literally or quasi economic systems of exchange of something or other.
Lucas Perry: So if we focus here on the next few decades where automation is increasingly taking over particular tasks and jobs, what is it that we can do to ensure positive outcomes for those that are beginning to be left behind by the economy that requires skill training and those whose jobs are soon to have many of the tasks automated?
James Manyika: Starting now, actually, in the next decade or two, I think there're several things, there's actually a pretty robust list of things we need to do actually to tackle this issue. I think one is just reskilling. We know that there's already a shortage of skills. Even before we think about, we've had skill mismatches for quite a while before any of this fully kicks in. So this is a challenge we've had for a while. So this question of reskilling is a massive undertaking, and here, the question is really due to pace and scale, because while there are quite a lot of reskilling examples one can come across, and there are many of them that have been very successful. But I think the key thing to note about many of them, not all of them, but many of them is that they tend to be small.
One of the questions one should always ask about all the great reskilling examples we hear of is, how big is it, right? How many people well went through that program? And I think you'll find that many of them, not all of them, many of them are relatively small. At least small relative to the scale of the reskilling that we need to do. Now, there've been a few big ones, I happen to like, for example, Walmart has had these Walmart academies, it's been written about publicly quite a bit, but what's interesting about that is, it's one of the few really large scale reskilling, retraining programs through their academies. Then something like, I can't remember reading this, but they've put something like 800,000 people through those academies. I like that example, simply because the numbers talked to sound big and meaningful.
Now I don't know. I haven't evaluated the programs, are they good? But I think the scale is about right. So, the reskilling at scale is going to be really important, number one.
The other thing we're going to need to think about is, how do we address the wage question? Now, the wage question is important, for lots of reasons here.
One is, if you remember earlier in our conversation, we talked about the fact that over the last two decades, for many people, wages haven't gone up, been relative wage stagnation, compared to rates of inflation, or the cost of living, and how things have gone up. Wages haven't gone up.
The wage stagnation is one we already have, before we think about technology. But then, as we've just discussed, technology may even exacerbate that, even when there are jobs, and the continuing changing structure of our economy will also exacerbate that. So what do we do about the wage question?
One could consider raising minimum wage, right? Or one could consider ideas like UBI. I mean, we can come back and talk about UBI. I have mixed the views about UBI. What I like about it is the fact that it's at least a recognition that we have a wage problem, that people don't earn enough to live. So I like it in that sense.
Now the complication with it, in my view, is that while, of course, one of the primary things that work does for you, for the vast majority of people, that's how they derive their livelihood, their income. So it's important, but work also does other things, right? It's a way to socialize, it's a way to give purpose and meaning, et cetera.
So I think UBI, it may solve the income part of that, which is an important part of that. It may not address the other pieces of the other things that work does. So, we have to solve the wage problem.
I think we also have to solve this geographic concentration problem. We did some work where we looked at all the counties in America at the time that we did this, because the definition of what's a county in America kind of changes a little bit year from year. But at the time that we did this work, which was back in 2019, though I think we looked at something like 3,149 counties across America.
What we're looking at there was, it was a range of factors about economic investment, economic vibrancy, jobs, wage growth. We looked at 40 different variables in each county, but I'm just going to focus on one, which is job growth.
When we looked at job growth across those counties, while at the national level, we're all celebrating the job growth that had happened, coming out of the 2008 recession, between 2008 and 2018 was the data set we looked at, first of all, at the national level, it was great. But when you looked at it at the county level, what you suddenly found is that a lot of that job growth was concentrated in places where roughly a third of the nation's workers live.
The other two-thirds of the place where people live either saw flat or no job growth, or even continued job decline. All of that is to say, we also have to solve this question of, how do we get more even job growth and wage growth across the country, in the United States?
We've also done similar work with, we've looked at these micro regions in Europe, and you see similar patterns, although maybe not quite as extreme as the US, but you see similar patterns where some places get a lot of the job and wage growth, and some cases get less of it. It's just a function of the structure of our economy. So we'd have to solve that, too.
Then the other thing we need to solve is the classic case of the hollowing out of the middle class. Because if you look at the pattern of, mostly driven by technology, to some extent, a lot of the job declines or the jobs lost as a result of technology have primarily been in the middle wage, middle-class jobs. And a lot of the job growth has been in the low wage jobs.
So this question of the hollowing out of the middle class is actually a really particular problem, which has all kinds of sociopolitical implications, by the way. But that's the other thing to figure out. So let me stop there.
But I think these are some of the things we're going to need to tackle in the near term. I've made that list mostly in the context of say, an economy like the United States. I think if you go outside of the United States, and outside of the advanced economies, there's a different set of challenges.
I'm talking about places outside of the OECD countries and China. So you go to places like India, and lots of parts of Africa and Latin America, where you've got a very different problem, which is demographically young populations. China isn't, but India and most of Africa is, and parts of Latin America are.
So there the challenge is, a huge number of people are entering the workforce. The challenge there is, how do you create work for them? That's a huge challenge, right? When you're looking at those places, the challenge is just, how do you create enough jobs in very demographically young countries?
The picture's now gotten a little bit more complicated in recent years than perhaps in the past, because in the past, the story was, if you are a developing country, a poor developing country, your path to prosperity was to join the global economy, be part of either the labor supply or the cheap labor supply often, and go from being an agrarian country to an industrialized country. Then ultimately, maybe some day, you'll become a service economy. Most advanced economies are.
That path of industrialization is less assured today than it used to be, for a bunch of reasons. Some of those reasons have to do with the fact that advanced economies now no longer seek cheap labor abroad as much as they used to. They still do for some sectors, but less so for many other sectors, I mean, we're less likely to do that.
Part of that is technology, the fact that in some ways, manufacturing has changed. We can now, going forward, do things more like 3-D printing, and so forth. So the industrialization path is less available to poor countries than it used to be.
In fact, economists like Danny Roderick have written about this, and called it this kind of premature de-industrialization challenge which is facing many low income countries. So we have to think about what, is the path for those countries?
And by the way, these are countries, if you think about it from the point of view of technology and AI, in particular, the AI technological competition globally rapidly seems to come down to be a race between the US, led by the US, but increasingly by China, and others are largely being left behind. That includes in some cases, parts of Europe, but for sure, parts of the poor developing economies.
So the question is, in a future, in which capacity for technology's developing a different pace, dramatically different paces for different countries, and the nature of globalization itself is changing, what is the path for these poor developing countries? I think that's a very tough question that we don't have very many good answers for, by the way.
But there have just been people who think about developing economies in developing economies themselves. That's one of the tough challenges, I think, for the next several decades of the 21st century.
Lucas Perry: Yeah, I think that this is a really good job of explaining some of these really significant problems. I'm curious what the most significant findings of your own personal work, or the work more broadly being done at McKinsey are, with regards to these problems and issues. I really appreciate some of the figures that you're able to share. So if you have any more of those, they're really helpful, I think, for painting a picture of where things are at, and where they're moving.
James Manyika: Well, I think the only other question on these kind of left behind countries and economies, as I said, these are topics that we're trying to research and understand. I don't think we have any kind of pat simple solutions to them.
We do know, though, that in fact, so if you look at the pattern, a lot of our work is very empirical. I mean, typically, I'm looking at what is actually happening on the ground. One of the things that you do see for developing economies is that the developing economies that are part of a regional ecosystem, either because of the value chains and supply chains.
Take the case of a country like Vietnam. It's kind of in the value chain ecosystem around China, for example. So it benefits from being a participant or an input into the Chinese value chain.
When you have countries, and you could argue that's what's happened with countries like Mexico and a few others, so there's something about being a participant in the value chains or supply chains of these that are emerging somewhat regionally, actually. That seems to be at least one path.
The other path that we've seen is that when you've got a developing countries that tend to have large and competitive private sectors, and emphasize "competitive," that actually seems to make a difference. So we did some empirical work where we looked at something like 75 developing countries over the last 25 years, to see what are some of the patterns of which ones are those that have done well, because of their growth and development, and so forth?
Some of the factors that you see, we found in that research, is in fact, when the countries that happened to have, as I said, one is proximity to either all participants in the global value chains of other large ecosystems or economies did well.
Second, those that seem to have these large and vibrant and very competitive private sector economies also seem to do better. Also, those that had resource endowments did well, so that I don't know, oil and natural resources, and those kinds of things, also seemed to do well.
Then we also find that those that seem to have more mixed economies, so they didn't just rely on one part of their economy, but they had two or three different kinds of activities going on in their economy, they had maybe a little bit of a manufacturing sector, and a little bit of an agricultural sector, a little bit of a service sector, so the ones that had more mixed economies seem to do well. The other big thing was, the ones that seem to be reforming their economies seem to do well.
So those are some patterns. I don't think those are guaranteed, in any of them, to be the recipe for the next few decades, partly because much of that picture on global supply chains is changing, and much of the role of technology and how it affects how people participate in the global economy is changing.
I think those are useful, but I don't know if they're any short recipe, going forward. There certainly have been the patterns for the last 25 years, but maybe that's a place to start, if you look forward.
Lucas Perry: To pivot a bit here, I'm curious if you could explain what you see as the central forces that are currently acting on the global economy?
James Manyika: Well, I'll tell you some of the things that are interesting, that we find interesting. One is, in fact, the fact that more and more and more and more, the role of technology in the global economy is getting bigger and bigger and bigger, in the sense that technology seems to have become way more general purpose in the sense that it's foundational to every company, every sector and every country.
So the role of that is interesting. It also has these other outsize effects, because we know that technology often lead to the phenomenon of superstar firms and superstar returns, and so forth. You see that quite a bit, so the role of technology is an important one.
The other one that's going on is what's happening with globalization itself. And by globalization, I just mean that the movement of value and activity related to the global economy.
We did some work a few years ago, that we've tried to update regularly, where we looked at all the things of economic value. So we looked at, for example, the flow of products and goods across the world, the flow of money, finances, and other financing and other things, the flow of services, the movement of people, and even the movement of data, and data-related activities.
What was interesting is that one of the things that has changed is that the globalization in the form of the flow of goods and services, it actually kind of slowed down, actually. That's why one of the reasons people were questioning is, is globalization dead, has it slowed down?
Well, it certainly looks that way. If you're looking at it through the lens of the flow of products and goods, but not the case if you're looking, necessarily, at the flow of money, for example, not necessarily if you're looking at the flow of people, and for sure not the case, if you're looking at the flow of data around the world.
One of the things that's, I think, underappreciated is just how digitized the global economy has become, and just the massive amounts of data flows, digital data flows that now happen across borders between countries, and how much that is tied into globalization works. So if you're looking at globalization through the lens of digitization, digital data flows, nothing has slowed down. In fact, if anything, it's accelerated, actually.
That's why, often, you will hear, people were looking at it through that lens, and say, "Oh no, it's even more globalized than ever before." But people who are looking at it through the flow of products and goods, for example, might say, "Oh, it seems, it looks like it is slowed down." That's one of the things that's changing.
Also, the globalization of digital data flows is actually interesting, because one of the things that it does is it changed the participation map quite significantly. So we did some work, where if you look at it through that lens, you suddenly found that you have many more countries participating, and many more kinds of companies participating, as opposed to just a few countries and a few companies participating in the global economy. You have much more diversity of participation.
So you have very tiny companies, a two- or three-person company in some country, plugged into the global economy, using digital technology and digital platforms, in ways that wouldn't have happened before, if you had a two- or three-person company 30 years ago. So this digitalization of the global economy is really quite fascinating.
The other thing that's going on to the global economy is the rebalancing, where, with the emergence of China's a big economy in its own right, that is changing the gravitational structure, if you like, of the global economy in some, in some very profound ways, in ways that we haven't quite had before. Because, sure, in the past you've had other large economies like Germany and Japan and others, as large economies, but none of them were ever as big as the United States.
Also, all of them, whether it's Japan or Germany or any of the European countries, largely operated in a framework, a globalization and a global framework, that was largely kind of Western-centric in a way. But now you have this very large economy that's very different, is very, very large, will be the second largest economy in the world. That is quite different, but yet is tied into the, so that gravitational structural shift is very, very important.
Then, of course, the other thing that's happening is, what's happening with supply chains and global value chains. And that's interesting, partly because we're so intertwined with how supply chains and value chains work, but at the same time, it changes how we think about the resilience of economies. We've just seen that during this COVID last year, where, all of a sudden, everybody got concerned about the resilience of our supply chains with respect to, essential products and services like medical supplies and so forth.
I think people are now starting to rethink about how do we think about the structure of the global economy, in terms of these value chains. We should have at some point also mentioned other kinds of technologies that are happening. Because it's not all AI and digital technologies, as much as I love that, and spend a lot of time on that.
I think other technological developments that are interesting include what's happening in biosciences or the life sciences. We've just seen spectacular demonstrations of that with the MRNA vaccines that were rapidly developed.
But I think a lot more has been happening with just amazing progress, that we're still at the very early stages of, with regards to the biotechnology and the life sciences. I think we're going to see even more profound, societally, and profound impact from those developments in the coming decades.
So these are some of the things that I see happening in the global economy. Now, of course, climate change looms large over all of this as a thing that could really impact things in quite dramatic and quite existentially concerning ways.
Lucas Perry: In terms of this global economy, can you explain what the economic center of gravity is, where it's been, and where it's going?
James Manyika: Well, undoubtedly, the economic center of gravity has been the United States. If you look at the last 50 years, it's been the largest economy on the planet, largest in every sense, right? As a market to sell into, as its own market. Everybody around the world for the last 50 years has been thinking about, "How do we access and sell to consumers and buyers in the United States?"
It's been the largest market. It's also been the largest developer and deployer of technologies and innovation. So, in all of those ways, it's been the United States as the big gravitational pull.
But I think, going forward, that's going to shift, because current course and speed, the Chinese economy will be as large. And you now start to have even other economies becoming large, too, like India.
So I think economic historians have created a wonderful map, where they showed the movement of the gravitational central to the global economy. I think they went back 1,000 years.
While it's been in the Western Hemisphere, primarily in the United States, I think some of the mid-Atlantic, it's been shifting east, mostly because of the Chinese economy, primarily, but also India and others that have come to grow. That's clearly one of the big changes going on at the global economy, to its structure and its center of gravity.
Lucas Perry: With this increase of globalization, how do you see AI as fitting into and affecting globalization?
James Manyika: The impact on globalization? I don't think that's the way I would think about the impact of AI. Of course, it'll affect globalization, because any time you have anything to do with products, goods, and services, because AI is going to effect all of those things.
To the extent that those things are playing out on the global economy landscape, AI will affect those things. I think the impact of AI, at least in my mind, is first and primarily about any economy, whether it's the global economy or a national economy or a company, right? So I think it's profoundly going to change many things about how any economic entity works.
Because we know the effect, the capital labor inputs, we know it'll affect productivity, and we know it'll change the rates of innovation. Because imagine, in this conversation, at least, we talked, I think mostly about AI's impact on labor markets, we should not forget AI's impact on innovation, on productivity, on the kinds of creation of products, goods, and services that we can imagine and how hopefully it's going to accelerate those developments.
I mean, DeepMind dealing with AlphaFold, which is cracking a 50-year problem, that's going to lead to all kinds of, hopefully, biomedical innovations and other things. I think one of the big impacts is going to be how AI affects innovation, and ultimately productivity, and the kinds of things we're going to see, whether it's products, goods, and services that we're going to see in the economy.
Of course, any economy that takes advantage of that and embraces those innovations will obviously see the benefit to the growth of their economy. Of course, if on a global scale, on a global stage in the global economy, we have some countries do that more than others, then of course it'll affect who gets ahead, who's more competitive, and who potentially gets left behind.
One of the other things we've looked at is, what is the rate of AI participation, whether in terms of developments or contributing to developments, or just simply deploying technologies, or having the capacity to deploy technologies, or having the talent and people who can either both contribute to the deployment or the development, and also embrace it in companies and sectors? And you see a picture that's very different around the world.
Again, you see the US and China, way ahead of everybody, and you'll see some countries in Europe, and even Europe is not uniform, right, and some countries in Europe doing more of that than others, and then a whole bunch of others who are being left behind. Again, AI will impact the global economy in the sense of how it impacts each of the economies that participate, and each of the companies that participate in the global economy, in their products and services, and their innovations and outputs.
There are other things that'll play out in the global stage related to AI. But from an economy standpoint, I think I see it through the lens of the participating companies and countries and economies, and how that then plays out on the global stage.
Lucas Perry: There's also this facet of how this technological innovation and development, for example, with AI, and also technologies which may contribute to and mitigate climate change, all affect global catastrophic and existential risks. So I'm curious how you see global catastrophic and existential risks as potentially fitting into this evolution of the global economy of labor and society as we move forward?
James Manyika: Well, I think it depends whether you're asking whether AI itself represents a catastrophic or existential risk. Many people have written about this, but I think that question is tied up with the view on how do we think about, how close we are to AI, or how close we are to AGI, and how close we are to superhuman AI capabilities.
As we discussed earlier, I don't think we're close yet. But there are other to think about, even as we progress in that direction. This include some of the safety considerations, the control considerations, and how we make sure we're deploying and using AI safely.
We know that there's particular problems with regards to things like, even with narrow AI, as it's sometimes called, how do we think about reward and goal corruption, for example? How do we think about how we avoid the kind of interference, catastrophic interference, between, say, tasks and goals? How do we think about that?
There are all these kinds of safety related things, even on our way to AGI, that we still need to think about. In that sense, these are things to worry about.
I also think we should be thinking about questions of value and goal alignment. And these also get very complicated for a whole bunch of both philosophical reasons, but also quite practical reasons.
That's why I love the work, for example, that Stuart Russell has been doing on how we think about human compatible AI, and how do we build these kinds of, the value alignment and goal alignment, that we should be thinking about? So these are, even on our way to AGI, both the safety control and these kinds of value alignment, and somewhat normative questions, about how we think about normativity, and what does it even mean, to think about normative things in the case of value alignment with the AI? These are important things.
Now, that's if you're thinking about catastrophic, or at least to existential risk, with regards to AI, even way before you get to AGI. Then you have the kinds of things, at that point, that Nick Bostrom and others have worried about.
I think, because those are non-zero probability concerns, we should invest all effort into working on those existential, potentially catastrophic problems. But I'm not super worried about those any time soon, but that doesn't mean we shouldn't invest and work on those, the kinds of concerns that Nick and others write about.
But they are also questions about AI governance, in the sense of we're going to have many participating entities here. We're going to have the companies that are leading the development of these technologies.
We're going to have governments that are going to want to participate and use these technologies. We're going to have issues around when to deploy these technologies, use and misuse. Many of these questions become particularly important when you think about the deployment of AI, especially in particular arenas.
Imagine if, once we have AI or AGI that's capable of manipulation, for example, or persuasion, or those kinds of things, or capabilities that allow us to detect lies, or be able to interfere or play with signals' intelligence, or even cryptography and number theory. I mean, our cryptographic systems rely on a lot of things in prime number theory, for example, or if you think about arenas like autonomous weapons.
So questions of governance become evermore important. I mean, they're already important now, when we think about how AI may or may not be used for things like deep fakes and disinformation.
The closer we get to the kinds of areas that I was describing here, it becomes even more important to think about governance, and what's permissible to deploy where and how, and how do we do that in a transparent way? And how do we deal with the challenges with AI about attribution?
One of the nice things about other potentially risky technologies or developments like nuclear science, or chemical weapons, and so forth, is at least those things, they're easy to detect when they happen. And it's relatively easy to do attribution, and verify that it happened, and it was used.
It's much harder with the AI systems, so these questions of governance and so forth become monumentally important. So those are some of the things we should think about.
Lucas Perry: How do you see the way in which the climate change crisis arose, given human systems and human civilization? What is it about human civilizations and human systems that has led to the climate change crisis? And how do we not allow our systems to function and fail in the same way, with regards to AI and powerful technologies in the 21st century?
James Manyika: I'm not an expert on climate science, by the way. So I shouldn't speculate as to how we got to where we are. But I think the way we've used certain technologies and fossil fuels, I think, is a big part of that, the way our economies have relied on that as our only mode of energy, is part of that.
The fact that we've done that in a relatively costless way, in terms of pricing the effects on our environment and our climate, I think, is a big part of it. The fact that we haven't had very many as effective and as efficient alternatives, historically, I think, is a big part of that.
So I think all of that is part of how we got here in some ways, but I think others more expert than me can talk about that. I think if I think about AI, I think one of the things that is potentially challenging about AI, if, in fact, we think there's a chance that we'll get to these superhuman capabilities, and AGI, is that we may not have the opportunity to iterate our way there. Right?
I think, quite often, with a lot of these deployment of technologies, I think a practical thing that has served us well in the past has been this idea that, well, let's try a few experiments, we'll fix it if it fails. Or if it doesn't work, and we'll iterate and do better, and kind of iterate our way to the right answer. Well, if we believe that there is a real possibility of achieving AGI, we may not have the opportunity to iterate in that same way.
That's one of the things that's potentially different, perhaps, because we can't undo that, as it were, if we truly get to AGI. Thinking about these existential things, so there's maybe something of a similarity, or at least an analog with climate change, is that we can't just undo what we've done, in a very simple fashion, right?
Look at how we're now thinking about, how do we do carbon sequestration? How do we take carbon out of this, out of the air? How do we undo these things? And it's very hard. It's easy to go in one direction, it's very hard to go in the other direction, in that sense, at least.
It's always dangerous with the analysis. But at least in that sense, AI, on its way to AGI, may be similar in that sense, which is we can't always quite get to undo it in a simple fashion.
Lucas Perry: Are you concerned or worried that we will use AI technology in the way that we've used fossil fuel technologies, such that we don't factor in the negative effects or negative externalities of the use of that technology? With AI, there's this deployment of single objective maximizing algorithms that don't take account all of our other values, and that actually run over and increase human suffering.
For example, the ways in which YouTube or Facebook algorithms work to manipulate and capture attention. Do you have a concern that our society has a natural proclivity towards learning from mistakes, from ignoring negative externalities, until it reaches sort of a critical threshold?
James Manyika: I do worry about that. And then maybe, just to come back to one of your, I think, central concerns, back to the idea of incentives, I do worry about that, in the sense that there are going to be such overwhelming and compelling incentives to deploy AI systems, for both good reasons, and for the economic reasons that go with that. So there are lots of good reasons to deploy AI technology, right?
It's actually great technology. Look at what it's probably going to do to, in the case of health science, and breakthroughs we could make there in climate science itself, and scientific discovery and material science. So there's lots of great reasons to get excited about AI.
And I am, because it'll help us solve many, many problems, could create enormous bounty and benefits for our society. So we're going to, people are going to be racing ahead to do that, for those reasons, for those very good and very compelling reasons.
There are also going to be a lot of very compelling economic reasons. The kinds of innovations that companies can make, the kind of contributions to the economic performance of companies, the kinds of economic benefits, and that possibly that AI will contribute to productivity growth as we talked about before.
There's lots of reasons to want to go full steam ahead. And a lot of incentives will be aligned to encourage that, both the breakthrough innovations that are good for society. As I said, the benefits that companies will get from deploying and using AI until the innovations, the economy-wide productivity benefits, so, all good reasons.
And I think, in the rush to do that, we may in fact find that we're not paying enough attention, not because anybody is out of malice or anything like that, but we just may not be paying enough attention to these other considerations that we should have alongside, considerations about, what does this mean for bias and fairness?
What does it mean for, potentially for inequality? We know these things have scale superstar effects. What does that mean for others who get left behind? What does this mean for the labor markets and jobs and so forth? So I think we're going to need to find mechanisms to make sure that there's continued, but substantial effort, at those kind of other sides of the side effects of AI, and some of the unintended consequences.
That's why, at least, I think many of us are trying to think about this question, "What are the things we have to get right," even as we race towards all the wonderful things we want to get out of it, what are the other things we need to make sure we're getting right along the way?
How do we make sure these things... People are working on them, they're funded, there's support for people working on these other problems. I think that's going to be quite important, and we should not lose sight of that. And that's something I'm concerned about.
Lucas Perry: So let's pivot here, then, into inequality and bias. Could you explain the risk and degree to which AI may contribute to new inequalities, or exacerbate existing inequalities?
James Manyika: Well, I think on the inequality point, it's part of what we talked about before, right? Which is the fact that, even though we may not lose jobs in the near term, we may end up with creating jobs or complementing jobs in a way that have these wage effects, that could worsen the inequality question.
That's one way in which AI could contribute to attain equality. The other way, of course, is the fact that because of the scale effects of these technologies, you could end up with a few companies or a few entities or a few countries having the ability to develop and deploy, and get the benefits of AI, while the other companies or countries and places don't. So you've got that kind of inequality concern.
Now, some of that could be helped by the way as it is, because it was the case, it has been the case so far, that the kind of compute capacity needed to develop and deploy AI has been very, very large, and the data endowments needed to train algorithms has been very, very high, but we know the talent of people who are working on these things has been, up until now, relatively concentrated.
But we know that that picture's changing, I think. The advent of cloud computing, which makes it easy for those who don't have the compute capacity, is helping that. The fact that we now have ways to train algorithms, of pre-trained algorithms or other universal models and others, so that not everybody has to retrain everything every single time.
These scarcities and these kind of scale constraints, I think, in those particular ones, will get better as we go forward. But you do worry about those inequalities, both in a peoples sense, but also in a entity sense, where entities could be companies, countries, or whole economies.
I think the questions of bias are a little bit different. I think the set of questions of biases, just simply has to do with the fact that up until now, at least so far, anyway, most of the data sets that have been used to train these algorithms often come with societally derived biases. And I emphasize this, society derive bias. It's just because of the way we collect data and the data that's available and who's contributing to it.
Often, you start out with data sets, training data sets that reflect society's existing biases. Not that the technology itself has introduced the bias, but in fact, these come out of society. So what the technologies then do is kind of bake these biases in, into the algorithms and probably deploy them at scale.
That's why I think this question bias is so important, but I think often it gets conflated with the fact that, well, proponents of using these technologies will say, but humans already have bias in them, anyway. We already make biased decisions, et cetera.
Of course, that's a two-sided conversation. But at least to the case, the difference that I see between the biases we have already as human beings, versus the biases that could get baked into these systems, is that these systems could get deployed and scale in a way that, if I have biases that I have, and I'm in a room and I'm trying to hire somebody, and I'm making my biased decisions, at least, hopefully that only affects that one hiring decision.
But if I'm using an algorithm that has all these things baked in, and hundreds of millions of people are using the algorithm, then we're kind of doing that in scale. So I think we need to keep that in mind, as we have the debate about, people already have biases and saturated biases, that's true. So we need to do work on that.
But one of the things I like about the bias question, by the way, that these technologies are forcing us to confront is that it's actually forcing us to really think about, what do we even mean when we say things are fair, quite aside from technology?
I think they're forcing us, just like the UBI debate is forcing us to confront the question that people don't earn enough to live, the bias question's also forcing us to confront the question of, what is fair right? What counts as fairness? And I think all too often, in our society, we've tended to rely on proxies for fairness, right?
When we define it, we'll say, "Well, let's constitute the right group of people, a diverse enough group of people, and we will trust the decision that they make, because it's a diverse group of people," right? So yeah, if that group is diverse in the way we expect, then gender or racial or any other social income terms, and they make a decision, we'll trust it, because the deciding group is diverse.
That's just a fairness by proxy, in my view. Who knows what those people actually think, and how to make decisions? That's a whole separate matter, but we trust it, because it's a diverse group.
The other thing that we've tended to rely on is, we trust the process, right? If we trust the process that, "Hey, if it's gone through a process like this, we will live with the results, because we think that the process like that is fair and unbiased."
Who knows whether the process is actually fair, and that's how we've typically done it with our legal system, for the most part. That if you follow through, if you've been given due process and you've gone through a jury trial, then it must be fair. We will live with the results.
But I think, in all of those cases, while they're useful constructs for us in society, they still somewhat avoid defining what is actually fair. And I think, when we've started to deploy technologies, where, in the cases of AI, the process is somewhat opaque, because we have this kind of explainability challenge of these technologies. So the process is kind of black boxy, in that sense.
And if we automate the decisions with no humans involved, then we can't rely on this constituent group that, "Hey, the group of people decided this, so it must be fair." This is forcing us to come back to the age-old or even millennia-old question of what is fair? How do we define fairness?
I think there's some work that was done before, where somebody is trying to come up with all kinds of definitions of fairness, and they came up with something like 21. So I think we now are having an interesting conversation about what constitutes fairness. Do we gather data differently? Do we code differently? Do we have reviews differently? Do we have different people that develop the technologies differently? Do we have different participants.
So we're still grappling with this question, what counts as fair? I think that's one of the key questions, as we rely more and more on these technologies to assist, in some cases, eventually take over some of our decision-making, of course, only when it's appropriate, these questions will continue to persist, and will only grow, on how we think about fairness and bias.
Lucas Perry: In terms of fairness, bias, equality, and beneficial outcomes with technology and AI in the 21st century, how do you view the need for and path to integrating developing countries' voices in the use and deployment of AI systems?
James Manyika: Well, I don't know if there's any magical answers, Lucas. At some level, at a base level, we should have them participate, right? I think any participation, both in the development and deployment, I think, is going to be important. And I think that's true for developing countries. I think it's true for parts of even US society that's often not participating in these things.
I mean, it's still striking to me how the lack of diversity, and diversity, in every sense of the term, who is developing AI and who's deploying AI, whether they look within the United States or around the world, there are entities and places and communities and whole countries that are not really part of this. So I think we're going to need to find a ways to do that.
I think part of doing that is at least for me, it starts out with the recognition that capabilities and intelligence are equally distributed everywhere. I don't think there's any one place or country or community that has a natural advantage to capability and intelligence.
On that premise, we just need to get people from different places participating in the development and deployment, and even the decision-making that's related to AI, and not just go with the places where the money and the resources happen to be, and that's, who's racing ahead, both within countries, e.g., in the United States itself, or in other countries that are being left behind. I think participation, in these different ways, I think it's going to be quite, quite important.
Lucas Perry: If there's anything you'd like to leave the audience with, in terms of perspective on the 21st century on economic development and technology, what is it that you would share as a takeaway?
James Manyika: Well, I think, when I look ahead to the 21st century, I'm in two minds. On the one hand, I'm actually incredibly excited about the possibilities. I think we're just at the beginning of what these technologies, both in AI and so forth, but also in the life sciences and biotech, I think that the possibilities in the 21st century are going to be enormous, possibilities for both improving human life, improving economic prosperity, growing economies.
The opportunities are just enormous, whether you're a company, whether you're a country, whether you're a society, the possibilities are just enormous. I think there's more that lies ahead than behind.
At the same time, though, I think, alongside pursuit of those opportunities are the really complicated challenges we're going to need to navigate through, right? Even as we pursue the opportunities that AI and these technologies are going to bring us, we're going to need to pay attention to some of these challenges that we just talked about, these questions of potential inequality and bias that comes out of the deployment of these technologies, or some of the superpower effects that could come out of that, even as we pursue economic opportunities around the world, we're going to need to think about what happens to poor developing countries who may not keep up with that, or be part of that.
In every case, for all the things that I'm excited about the 21st century, which is plenty, there are also these challenges along the way we're going to need to deal with. Also the fact that society, I think, demands more from all of us.
I think the demands for a more equal and just society are only going to grow. The demands or desires to have a more inclusive and participative economy are only going to grow, as they should. So we're going to need to be working both sets of problems, pursuing the opportunities, because without them, these other problems only get harder, by the way.
I mean, try to solve the inequality when there's no economic surpluses, right? Good luck with that. So we have to solve both. We can't pick one side or the other, we have to solve both. At the same time, I think we also need to deal with some of the potentially existential challenges that we have, and may grow. I mean, we are living through one right now.
I mean, we're going to have more pandemics in the future than we have had, perhaps, in the past. So we're just going to need to be ready for that. We've got to deal with climate change. And these kinds of public health, climate change issues, I think, are global. They're for all of us.
These are not challenges for any one country or any one community. We have to kind of work on all of these together. So that set of challenges, I think, is for everybody, for all of us. It's on planet Earth, so we're going to need to work on those things too. So that's kind of how I think about what lies ahead.
We have to pursue the opportunities, there's tons of them. I'm very excited about that. We have to solve the challenges that come along with questioning those opportunities, and we have to deal with these collective challenges that we have. I think those are all things to look forward to.
Lucas Perry: Wonderful, James, thank you so much. It's really interesting and perspective shifting. If any of the audience is interested in following you or checking your workout anywhere, what are the best places to do that?
James Manyika: If you search my name and such McKinsey Global Institute, you will see some of the research and papers that I referenced. For those who love data, which I do, these are very data rich fact-based perspectives. So just look at the McKinsey Global Institute website.
Lucas Perry: All right. Thank you very much, James.
James Manyika: Oh, you're welcome. Thank you.
Lucas Perry: Thanks for joining us. If you found this podcast interesting or useful, consider sharing it on social media with friends, and subscribing on your preferred podcasting platform. We'll be back again soon, with another episode in the FLI Podcast.
Related episodes
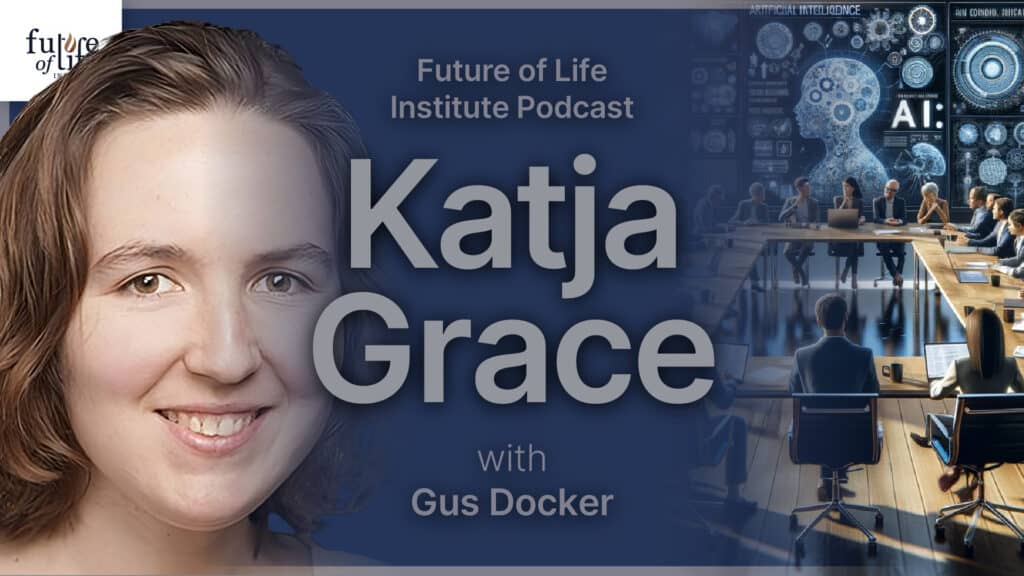
Katja Grace on the Largest Survey of AI Researchers
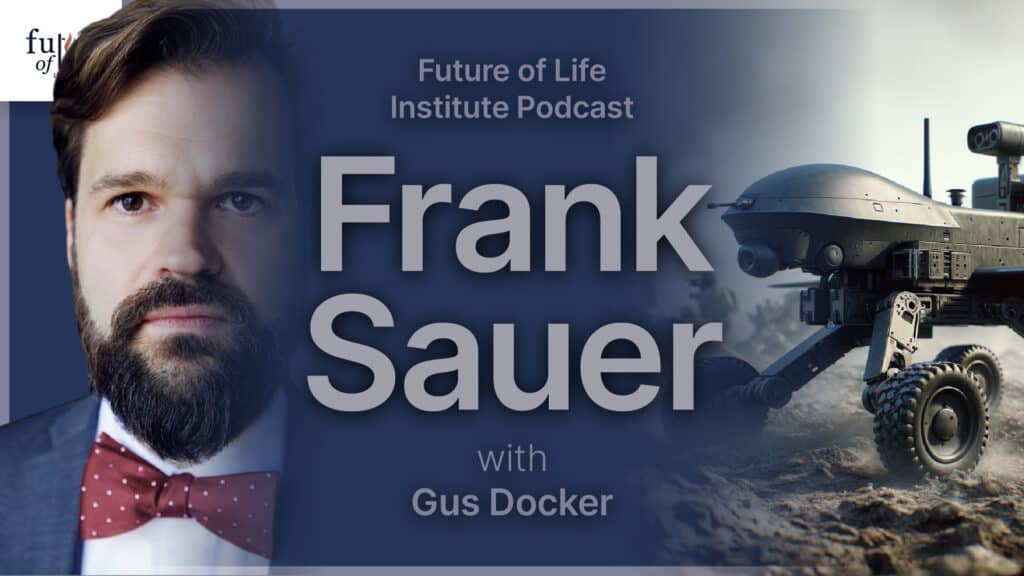
Frank Sauer on Autonomous Weapon Systems
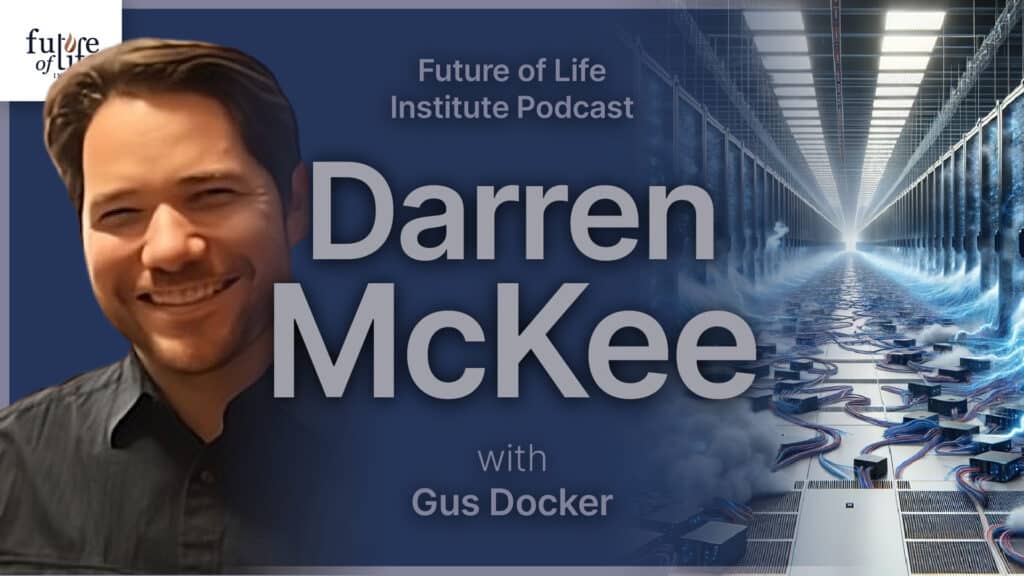