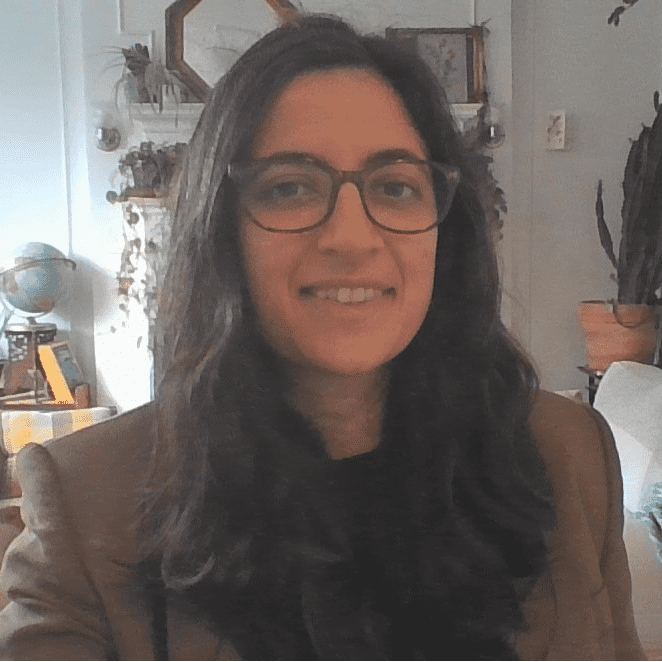
Tegan Maharaj
Why do you care about AI Existential Safety?
Life on earth has evolved over such a very long time, robust to such a huge range of earth conditions, that it’s easy to feel it will always be there, that it will continue in some way or another no matter what happens. But it might not. Everything *could* go wrong.
And in fact a lot is already going wrong — humans’ actions are changing the world more rapidly than it’s ever changed, and we are decimating the diversity of earth’s ecosystem. Time to adapt and multiple redundancy have been crucial to the adaptability and robustness of life in the past. AI systems afford the possibility of changing things even more rapidly, in ways we have decreasing understanding of and control over.
It’s not pleasant to think about everything going wrong, but once one accepts that it could, it sure feels better to try to do something to help make sure it doesn’t.
Please give one or more examples of research interests relevant to AI existential safety:
We are in a critical period of the development of AI systems, where we are beginning to see important societal issues with their use, but also great promise for societal good, generating widespread will to regulate & govern AI systems responsibly. I think there’s a real possibility of doing this right if we act now, and I hope to help make that happen.
These are my short (1-5 year) research foci:
(1) Theoretical results and experiments which help better understand robustness and generalization behaviour in more realistic settings, with a focus on representation learning and out-of-distribution data. E.g. average-case generalization and sample-complexity bounds, measuring OOD robustness, time-to-failure analysis, measuring ‘representativeness’.
(2) Practical methods for safe and responsible development of AI, with a focus on alignment and dealing with distributional shift. E.g. unit tests for particular (un)desirable behaviours that could enable 3rd-party audits, sandboxes for evaluating AI systems prior to deployment and guiding design of randomized control trials, generalization suites.
(3) Popularization and specification of novel problem settings, with baseline results, for AI systems addressing important societal problems (e.g. pricing negative externalities or estimating individual-level impact of climate change, pollution, epidemic disease, or polarization in content recommendation), with a focus on common-good problems.