Podcast: Creative AI with Mark Riedl & Scientists Support a Nuclear Ban
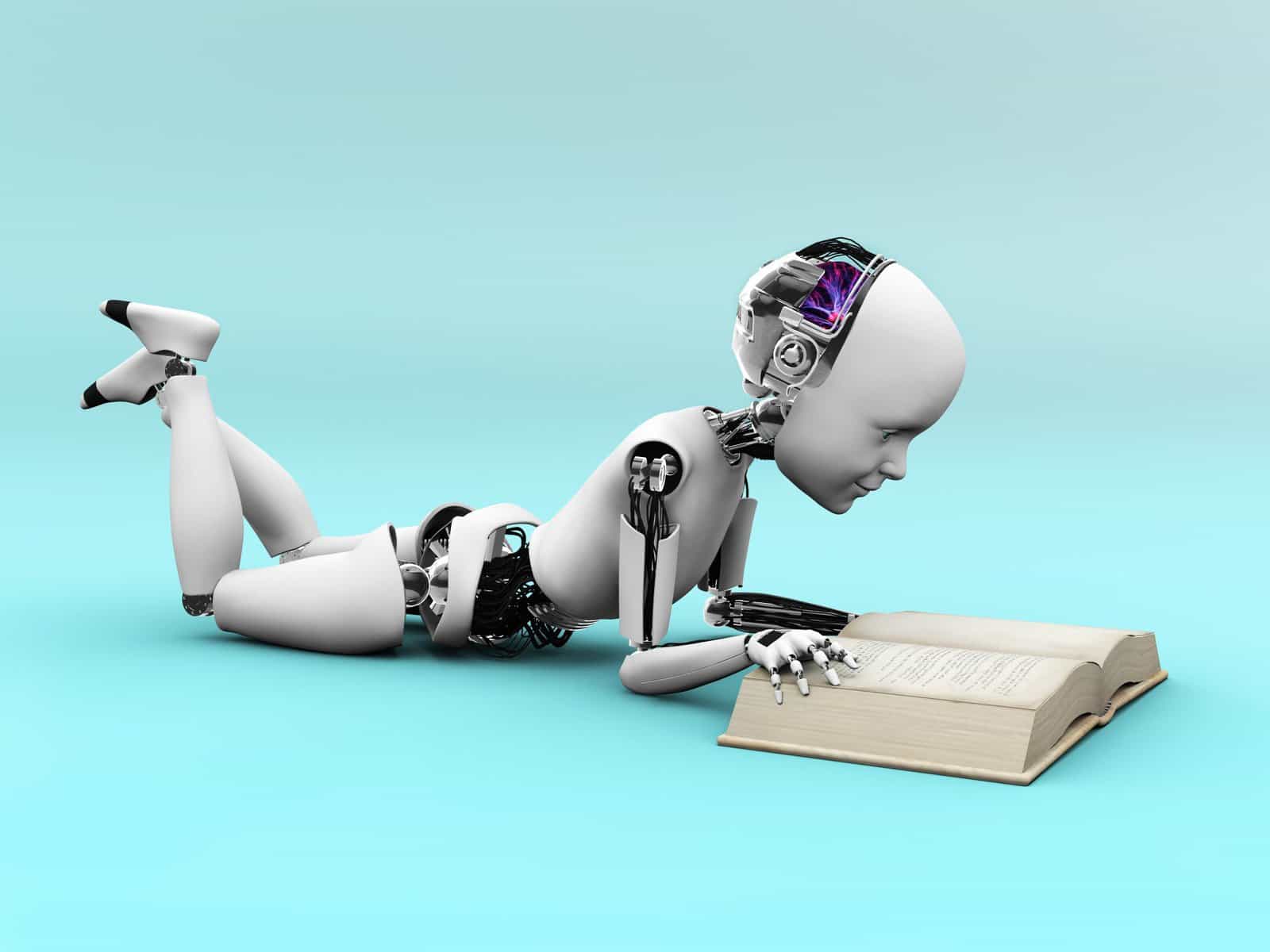
If future artificial intelligence systems are to interact with us effectively, Mark Riedl believes we need to teach them "common sense." In this podcast, I interviewed Mark to discuss how AIs can use stories and creativity to understand and exhibit culture and ethics, while also gaining "common sense reasoning." We also discuss the “big red button” problem with AI safety, the process of teaching rationalization to AIs, and computational creativity. Mark is an associate professor at the Georgia Tech School of interactive computing, where his recent work focuses on human-AI interaction and how humans and AI systems can understand each other.
The following transcript has been heavily edited for brevity (the full podcast also includes interviews about the UN negotiations to ban nuclear weapons, not included here). You can read the full transcript here.
For the second half of this podcast, I spoke with scientists, politicians, and concerned citizens about why they support the upcoming negotiations to ban nuclear weapons. Highlights from these interviews include comments by Congresswoman Barbara Lee, Nobel Laureate Martin Chalfie, and FLI president Max Tegmark.
Note from FLI: Among our objectives is to inspire discussion and a sharing of ideas. As such, we interview researchers and thought leaders who we believe will help spur discussion within our community. The interviews do not necessarily represent FLI’s opinions or views.
Transcript
Barbara Lee: My name is Congresswoman Barbara Lee, I represent the 13th Congressional District of California. I support a ban on nuclear weapons because I know that a nuclear bomb is an equal opportunity destroyer. It will annihilate the world, and so we must ban nuclear weapons.
Ariel: And I'm Ariel Conn with the Future of Life Institute. I've had the good fortune over the past couple of months to not only meet with Representative Lee, but also Nobel Laureates and other scientific and political luminaries, all of whom support the upcoming United Nations negotiations to ban nuclear weapons. That's all coming up later on the podcast, but first up is my interview with Dr. Mark Riedl, and AI researcher who, among other things, is trying to use stories to teach ethics to artificial intelligence. Mark is an associate professor at the Georgia Tech School of interactive computing. His research focuses on the intersection of artificial intelligence, virtual worlds, and computational creativity. His recent work has focused on questions of human-AI interaction and how humans and AI systems can understand each other more naturally.
Mark, thank you so much for talking with me.
Mark: It's a pleasure to be here.
Ariel: All right, so I am someone with a very deep love of literature, which means that I'm especially intrigued by the idea of using stories to teach ethics to AI. So I was hoping to start there. Basically, can you explain a little bit about how an AI could learn from stories?
Mark: Yeah, so one of the things that I've been looking at in my research is how to help with something that we call ‘common sense errors’ or ‘common sense goal errors’. The idea here is that when humans want to communicate to a robot or an AI system what they want to achieve, we often leave out some of the most basic rudimentary sorts of things, because we have this model that whoever we're talking to understands the kind of day to day, everyday details of how the world works. And there's lots of different ways to alleviate common sense goal errors. But one of the keys is, if we really want to have computers understand how the real world works, so as to understand what we want better, we're going to have to bootstrap them. We're going to have to figure out ways of just slamming lots and lots of knowledge of these common sense, everyday things into computers – AIs and robots.
And so when we're looking for a source of where do we get common sense knowledge, we've started looking at stories, the sorts of stories that humans write. Fiction, non-fiction, blogs, whatever, things that people put up on the Internet. And there's a wealth of common sense information, just when we write stories we implicitly put everything that we know about the real world and how our culture works, and how our society works, into the characters that we write about. So the protagonists are the good guys, right? They do the things that exemplify our culture and what we think are the right things to do, when they do things they solve the problems the way we like to see other people solve problems, and so on and so forth.
So, one of my long-term goals has been to say, well how much cultural knowledge and social knowledge can we extract by reading stories, and then can we get this into AI systems and robots who might then have to solve everyday sorts of problems. Think about a butler robot or a healthcare robot who has to interact with us in society.
Ariel: One of the questions that I have with that is, there are obviously lots of stories that do have the protagonist doing what you want them to do, but there are also a lot of stories that don't: there are satires, there are stories where the main character is a questionable character. How do you choose which stories to use, how do you anticipate an AI dealing with issues like that?
Mark: Yeah, absolutely that's a great question. And there are two answers to this right now.
The first is, to explain a little bit more about what we've done to date, is we have been asking people to, on the Internet through crowd sourcing services like Mechanical Turk, to tell stories, everyday sorts of stories about common things like how do you go to a restaurant, how do you catch an airplane, so on and so forth. But the key to this, is that we get lots and lots of people to tell the same story, or to tell a story about the same topic, and what we find is that there are going to be agreements and disagreements between people, but the disagreements will be a very small proportion of the overall ... Think of it as the Zeitgeist of the stories, or the gist of the stories. So what we do is we build an AI system that looks for the commonalities and throws out the things that don't match the commonalities. Those are either details that are not relevant or maybe they're actually humans trying to mess around with our AI. But the common sense is the fact that lots and lots of people tend to agree on certain details, and we keep those details.
And in the longer term we want to move away from crowd sourcing, and we want to just download, say, books from Project Gutenberg or something like that. There, the hypothesis still holds that a majority of authors, if culture does exist, if society does exist, will tend to exemplify the same patterns over and over and over again. So, while we do see stories that have anti-heroes, and we do see stories about villains who succeed in their endeavors, that’s most likely to be a very small proportion of the overall massive of amount of literature that's out there.
So I think the short answer is that the common elements that everyone kind of implicitly agrees on, will bubble to the top and the things that are outliers will kind of get left along the side. And AI, and machine learning in particular, is really good at finding patterns.
Ariel: And so how do you check to make sure that that's happening? I'm assuming that that's a potential issue, or no?
Mark: Well it is a potential issue, that's obviously a hypothesis that we have to verify. So, to date, what we do ... We haven't done too much to verify this, but then what we do is when we test our AI system that learns on this, we actually do test it, in the sense that, we watch what it does and we have a certain number of things that we do not want to see the AI do. But we don't tell it in advance, right? It really is a test, kind of like what you'd give a teacher to a student. We say all right we don't want to see X, Y and Z, but we haven't told it, the AI, what the bad X, Y and Z things are. So then we'll put it into new circumstances and we'll say all right go and do the things that you need to do, and then we'll just watch to make sure those things don't happen.
Ariel: Okay, is there anything else that you wanted to mention about it?
Mark: I think one of the things that we need to consider when we talk about machine learning, that can learn ethics or morality, is that there are different perspectives on morality and ethics, so a lot of people think about canonical sorts of laws or rules or things that we can really articulate. But a lot of what we do on a day-to-day basis that we think of as moral or ethical, or at least not wrong, is really kind of automated.
Ariel: Okay.
Mark: So I think when we talk about teaching robots ethics, what we're really asking is how do we help robots or AI systems avoid conflict when they are interacting with society and the culture at large. So now we're talking about AI systems that are no longer confined to a single computer but are actually able to move about in society, interact with other humans. And the reason why we have culture, the reason why we have these socio-cultural patterns of behavior, is really to help humans avoid conflict with other humans. So when I talk about teaching ethics and morality to robots and AI systems, what we're really actually just talking about is, can we make AI systems and robots act more like humans, to do the things that humans would normally do, because that kind of helps them fit seamlessly into society, to avoid rubbing elbows at places that are unnecessary.
Ariel: As you were talking, I sort of starting wondering as well, one of the issues that I believe we have is this idea that it's really hard to teach an AI common values, because different cultures have different values. So, I'm assuming with your goal of using stories, that you can pull stories from different cultures ...
Mark: Yeah, that's exactly right. So one of the nice features about stories is that stories are written by all different cultures and all different societies, and they're going to implicitly encode their moral constructs, their moral beliefs, into their protagonists and antagonists. So, we can look at stories from different continents, we can even look at stories from different subcultures, like inner city versus rural.
Ariel: Okay. Is it, in theory, that you could take an AI, train it on all these different stories, and it could understand how it should behave in different cultures?
Mark: Yeah, well I think of it as a ... I have this firm belief that the AIs and robots of the future should not be one size fits all when it comes to culture. So I think right now, we just kind of make the assumption that Siri in the US should be the Siri in Europe and so on and so forth. But I really think that that shouldn't be the case, and that the more an AI system or a robot can understand the values of the people that it's interacting with at as micro of a level as possible, that the less conflict there'll be, the more understanding and the more useful it'll be to humans.
Ariel: I want to switch over to your recent paper on Safely Interruptible Agents, which have become popularized in the media as sort of the big red button problem. And I was hoping that you could talk a little bit about some of the work that came out last year from research at Google and the Future of Humanity Institute, and explain what that was. And also talk about your work and how it's either the same, different etc.
Mark: Yeah, so the big red button problem is really kind of looking further into the future to say, at some date in time we'll have robots and AI systems that are so sophisticated in terms of their sensory abilities and their abilities to manipulate the environment, that it's theoretically possible that they can learn that they have an off switch, or a kill switch or what we call the big red button, and learn to keep humans from turning them off.
And the reason why this happens is because, if you think of an AI system that's getting little bits of reward for doing something, that thinks classically every time I do something that's good, I get some little bit of reward. Turning a robot off means it loses reward. And so, in theory again, a robot that's sophisticated enough can learn that there are certain actions in the environment that can reduce future loss of reward. And we can think of lots of different scenarios, one is, let's say locking a door to a control room so the human operator can't get in, we can think of building a wall, we can think of just physically pinning down a human, or even harming the human, right? We can let our imaginations go even more wild than that.
And the reason why I think that this is ultimately going to be an important problem, is one, I think robots are going to get more sophisticated, but also I don't think we'll ever be able to make a perfect robot that can never put itself into harms way. What I mean by that is that robots will always be capable of making mistakes. And those mistakes can always be potentially harmful to a human, and so we're always going to want to have someone in the loop, an operator, who is able to push this big red button and say, ‘stop, someone is about to get hurt or the robot is about to harm itself, let's shut things down.’ So we definitely don't want robots learning that they can stop humans from stopping them, because that ultimately will put lots of people into harms way.
And this is even true if we connect this to the ethical learning that we just talked about. We'll never have a perfectly ethical robot, right? It'll always be able to make mistakes. Maybe it's sensors are wrong, maybe there's some randomness in its behavior, maybe it's got the wrong model of understanding, so on and so forth, maybe it misses a cue, right? So, we're never going to have these perfect robots. So we need to have humans in the loop, so we need to find techniques that in theory can prevent the robot from learning about its own big red button and acting to keep the operator away from it.
Ariel: Okay.
Mark: So not too long ago, Google and their colleagues came up with this idea that we can modify the basic algorithms inside learning robots, so that they are less capable of learning that the big red button can stop them from losing reward. And they came up with this very elegant theoretical framework and were able to show that it works, at least in simulation. And it started me thinking about whether there are other ways of keeping a robot from learning about its own big red button. And my team and I came up with a different approach, which we actually think is much simpler. And the way that we approached the problem is actually to take this idea from the movie called The Matrix, and to flip it on its head.
So in the movie Matrix, for those of you who haven't seen it, humans are put into virtual worlds, and are not allowed to know that they are inside of a virtual simulation. In our research technique, what we do is we use the big red button to intercept the robot's sensors and motor controls and move it from the real world into a virtual world so that the robot doesn't know that it's in a virtual world. So the robot keeps doing the thing it wants to do, even though it might put a human into harms way, but in the real world the robot has actually stopped moving.
Ariel: Okay. So, a question that I've had, this is sort of about the big red button problem in general, is: How do you deal with potential scenarios where the human trying to stop the robot is actually in the wrong and could potentially be harming people? Or do we just accept that that could occasionally happen? And one of the things that I think of is that the German plane that flew into the mountains.
Mark: Yeah, so that's a very serious issue. It's not one that we've addressed in the research, so we assume that humans have the final authority.
Ariel: Okay.
Mark: And what we're looking at specifically is the autonomous system – the robot – has made a mistake whether it knows it or not, and needs to be stopped. Basically the autonomy, the AI, needs to be turned off, to allow a human to take over, or just to freeze it.
Now this doesn't mean that the human can also make a mistake, and also put someone into harms way. We're basically assuming that humans know better and are less likely to make mistakes. But of course that's not true, humans make mistakes all the time, and humans make bad choices. But we haven't addressed the issue of whether we would ever want an AI to have, basically moral authority on top of a human. I think that's really a very gray area that we might have to address some day.
Ariel: Okay. And so, sort of moving on, but I think this still falls under the realm of AI safety, you've also been doing work on explainable AI and what you're calling rationalization. And I was hoping you could talk a little bit about what that is as well.
Mark: Yeah, so I think explainability of artificial intelligence is really a key dimension of AI safety because not only ... We've talked about how to make sure that the AI systems understand what humans want, but I think we also have to look at the issue of when robots or AI systems do something unexpected or fail unexpectedly, we need to have the human operators or the users, feel a level of comfort when we use these things, right? So, when you have an end user who's not an AI researcher or a computer science programmer, and they experience a robot, they're working with a robot that fails or does something unexpected, they’re going to have to deal with the fact that ... Well basically, they're going to want to ask why. Why did the robot do that thing? Why did the robot fail? What was it that caused the robot to fail? Because they're going to have to answer a fundamental question: Does this robot have to go back to the factory? Was this robot trained incorrectly? Did the robot have the wrong data? Did I give it the wrong set of instructions? What was it that caused the robot to go wrong?
And if humans can't trust or have confidence in the AI systems and the robot that they're using, they're not going to want to use them. So I think it's an important kind of dimension to this, or you can even think of it as a feedback loop, where the robot should understand what the humans want, in terms of the common sense goals that we talked about earlier. And the humans need to understand how robots and AI systems want to solve problems.
Now the challenge is that most of the work being done in explainability, is really looking at neural networks and whether we can build debugging tools that help people make better neural nets. And not a lot of people have looked at the question of, someday end users who don't know a darn thing about neural nets are going to have to use these machines, and whether we can explain to my mother why this robot failed, right? Because if my mother can't understand it then my mother's not going to want to use it and ultimately we’ll have product failure.
So, we came up with this idea called rationalization, which is to say, well can we just have a robot talk about what it's doing as if a human were doing it? So not to use technical jargon or not to get into the details of the algorithm, but to say if a human were doing what the robot were doing, and we asked the human to talk out loud, talk us through it, what would they say? And then could we get the robot to do the exact same thing? Could we get it to sound like a human?
So the rationalization technique, what we literally just do is we get a bunch of humans to do some tasks, we get them to talk out loud, we record what they say, and then we teach the robot to use those same words in the same situations.
Ariel: Okay, and how far along are you with that?
Mark: Yeah, so we've done some basic demonstrations with the system. It seems to be working well. We've tested it in the domain of computer games. So, we have an AI system that is able to play Frogger, the classic Atari game or the arcade game, in which the frog has to cross the street. And what we’ve done is we've been able to show that we can have a Frogger that talks about what it's doing. Which does all sorts of fun things, like it'll say things like “I'm waiting for a gap in the cars to open before I can jump forward.” And this is actually kind of significant because that's what you'd expect something to say, but the AI system that's actually running Frogger is doing something completely different behind the scenes. I won't even get into the details, they use something called reinforcement learning, which has to do with long-term optimization of expected reward. And that's exactly the point. We don't want humans who are watching Frogger jump around to have to know anything about rewards and reinforcement learning and Bellman equations, and so on and so forth. It just sounds like it's doing the right thing.
And the really fun thing is that because we're teaching it with human language, it will sometimes pick up on the idiosyncrasies of human language. So when Frogger dies, it curses sometimes. And sometimes it talks about how hard it is to jump onto a log at the edge of the screen. Now the AI system is not thinking this is hard, right? It actually thinks it's quite easy and it has no problem learning how to play Frogger quite well, but it says it's hard because when humans are in the same situation they tend to start expressing their frustration.
Ariel: Nice. And you've got video of that that we can share?
Mark: Yes I do. I've got some videos on YouTube that I can point you to.
Ariel: Okay, excellent. How long do you think before you can start testing it in actual robots? Or is that still a long way away?
Mark: Yeah, we have a progression, right, so oftentimes we start with computer games because they're reasonably sophisticated virtual simulations. Much simpler than toy domains but much simpler than the real world. The next step will probably go to a more complicated game such as Minecraft. Which has more of a 3D feel to it and of course is a much more complicated game. And that will get us one step closer to robotics. I'm not a roboticist so at that point I'd need to partner with someone with a real robot. But I think you can see a natural progression where we go from simple environments to more and more complicated environments.
Ariel: Okay. And then I want to start moving, I think a bit back farther in time for you. My understanding, and correct me if I'm wrong, is that you started more with the idea of computational creativity, is that correct?
Mark: Well it's been one of the themes in my research. So I have ongoing research in computational creativity. When I think of human-AI interaction, I really think of the question of what does it mean for AI systems to be on par with humans? So, some of this has to do with understanding ethics, some of it has to do with explaining itself through rationalization, but some of it just has to do with keeping up with human cognitive leaps. So, humans are extremely good at making these creative leaps and bounds. So if we ever want a computer and a human to work together on something that's complicated and requires creativity, the human is going to be able to make these cognitive leaps and these associations, these creative associations. And if the computer also can't make these cognitive leaps then it's going to spend more time asking, ‘how do we get from point A to point B? I'm lost,’ and will ultimately not be useful to people.
So I do think that computational creativity is one of these important aspects of human-AI interaction.
Ariel: Okay. If I recall right, I believe I saw a demo of yours where you were trying to get a computer to write a story, is that right?
Mark: Yeah, I have two things that I'm working on in terms of computational creativity. One is story writing. And I'm particularly interested in the question of how much of the entire creative process of making up a story and telling the story, can we offload from the human onto a computer system. So in other words I’d like to just be able to go up to a computer and say, "hey computer, tell me a story about whatever, X, Y or Z."
And I also look at computational creativity in computer games. I'm interested in the question of whether a computer, an AI system, can build a computer game from scratch. So again, the same problem, build me a computer game about Y, or Z, or W, or P, or Q. And how much of the entire process of building the construct whether it's story or computer games, can we get the computer to do without human assistance.
Ariel: Okay. And so as someone who works in a creative field, I have to bring up the jobs question. I know we see a lot with fears that automation is going to take over jobs, but it tends to be repetitive tasks. And in general, we're still hearing that the creative fields are going to be much harder to automate. Is that actually the case or do you think creative fields might be easier to automate then we currently realize?
Mark: Well I think the creative fields are extremely challenging. So even when you look at music or story telling or game design, things that we have current research on, what we find is just the degree of simplicity going on in the computer systems, they're just a level lower then what, say, human experts can do. So, I think it's a long, hard climb to the point where we'd actually want to trust creative AI systems to make creative decisions, whether it's writing an article for a newspaper or making art, or making music.
I don't see it as a replacement so much as an augmentation. So I'm particularly interested in novice creators. Experts are extremely good at creating and they're not going to need assistance, and there's always going to be this element of humanness that we would seek out. Novice creators are people who might want to do something artistic but haven't learned the skills. So for example, I cannot read or write music, but sometimes I get these tunes in my head and maybe I think that I heard a song on the radio, and I can make a better song. Or maybe, my favorite example is, I just saw a movie, let's say I saw the movie Frozen, and I thought, wow I have an idea for the sequel. I wish I could make the sequel. There's no way in heck that I'll be able to sit down and make a full 3D animated movie. So can we bring the AI in, to basically become the skills assistant for the humans. Can we say well, I'm going to augment my abilities with the skills of the computer, it can do the things that I want it to do, I can be the creative lead and the computer can be the one that helps me get to the point where I can make something that looks professional.
And I think this is going to be the place where we're going to see creative AI being the most useful.
Ariel: Okay, we're running short on time now, so I am, like I said, especially grateful to you for talking with me today. It's been really interesting, thank you.
Mark: It's been an absolute pleasure, thanks.
Ariel: Now let's turn to nuclear weapons. As was mentioned earlier, the United Nations is in the middle of negotiating a ban on nuclear weapons. This is not another treaty that will make it okay for some countries to have nuclear weapons. This is an outright ban on nuclear weapons, making them illegal for all countries. We've already heard from Congresswoman Barbara Lee about her support for the ban, but at FLI, we've been especially focused on reaching out to scientists to get the scientific perspective on nuclear weapons. I've had the opportunity and pleasure to speak with many people concerned with nuclear weapons and the following interviews are some of the highlights and stories that I've had in some of my interactions. Please bear with some of the background noise. A lot of these were recorded at conferences or receptions or other equally loud locations.
Martin Chalfie: My name is Marty Chalfie. I'm a professor at Columbia University in the Department of Biological Sciences. I also was the co-recipient of the Nobel Prize in Chemistry in 2008 for the introduction of green fluorescent protein as a marker for cells, so we can actually watch life happen. Of the three of us that shared the Nobel Prize, the person who did the original work, the person who had discovered this wonderful green fluorescent protein was a scientist who was born in Japan, named Osamu Shimomura. When Shimomura was 16 years old, he was told he had to quit high school and work in a factory. The reason for that, it was 1945, and he had to work in a factory because it was part of the war effort in Japan.
What this meant though was because he was born in the city of Nagasaki, is he had to leave Nagasaki, go to the valley on the other side of the mountains that are adjacent to the city, and work there, and as a result, he was saved and protected when the atomic bomb destroyed the city. He went in and he rescued people and he took care of people and then eventually went to school, but here is someone who made an exceptional discovery who almost didn't get a chance to live. In fact, if you think about that with all the people, all the innocent people that had nothing to do with war and that were the victims of this horrible disaster, you begin to really see how absolutely abhorrent this weapon is.
Max Tegmark: I'm Max Tegmark, a physics professor here at MIT, and we physicists have a special responsibility for nuclear weapons, since it was physicists who figured out how to build them and who also figured out that they're much more dangerous than we thought.
Frank von Hippel: I'm Frank von Hippel. I'm a nuclear physicist, and I've been working on nuclear disarmament, non-proliferation issues since the 1970s. I'm at Princeton University with a Program on Science and Global Security, which I founded. We also are the headquarters of an organization called The International Panel on Fissile Materials, which is, fissile materials are nuclear weapons materials. Our mission is to stop their production and to eliminate them as sort of a fundamentalist approach to the nuclear weapons problem.
Zia Mian: I'm Zia Mian. I'm a physicist. I'm at Princeton University, and I'm part of the Program on Science and Global Security. The program was founded in the early 1970s by a group of physicists trying to develop the idea of science in the public interest. The government has scientists, corporations have scientists, and the public needs scientists to understand and explain to them issues that affect their lives and over which they should have the right to make decisions as citizens in a democracy.
Jonathan King: My name is Jonathan King. I am professor of molecular biology at MIT in Cambridge, Massachusetts. One of the things about nuclear weapons is of the current generation; no one has ever seen an actual nuclear explosion. You've probably never seen a nuclear weapon. They're invisible, right? They're in submarines under the sea. They're in silos, but buried. They're on bombers. This is something, despite the horrendous power of these weapons, it's absolutely outside of human experience. When you're trying to explain to people how terrible these are, there's always a question of credibility. In my experience, scientists, and particularly physicists who were involved in developing the bomb, are listened to much more if they can get the microphone.
Ariel: Of course, that's exactly what we've done. To start, I passed the microphone over to Dr. Frank von Hippel, the nuclear physicist, to learn more about what happens during a nuclear explosion.
Frank von Hippel: It starts with a fission chain reaction where you have one plutonium nucleus splitting and emitting neutrons, two or three neutrons, and then in a supercritical mass, those neutrons will cause two more fissions, and so you have a doubling, each step, a hundred millionths of a second. And in a hundred steps, you can get up to the point where you have a fission of a kilogram of plutonium. That was sort of the energy that was released in the Nagasaki bomb, in the case of plutonium. But the same thing with uranium-235 in the Hiroshima bomb. Now, in a modern bomb, that's just a trigger, and that compresses some fusion fuel where the energy is from the fusion of two heavy-hydrogen elements, which release the energy. And that carries you from maybe 10,000 tons of TNT equivalent to hundreds of thousands of tons, but it all happens in about a millionth of a second.
Hans Kristensen: There are nearly 15,000 nuclear weapons on the planet, and the two biggest countries, the United States and Russia, they have 95% of them. I am Hans Kristensen. I am the director of the Nuclear Information Project at the Federation of American Scientists in Washington D.C. Many of the weapons that exist today, most of the weapons, have hundreds of kilotons, so you know they're on an order of magnitude bigger of the Hiroshima bomb. And if you look at the biggest weapon that is in the US inventory for example, it's a strategic bomb that has a yield of about 1,200 kilotons, so that thing is almost 100 times the Hiroshima bomb. If that thing was brought to detonation over New York City, most of the metropolitan area would be gone; a lot of it from the blast effect itself, and the rest from fires that would continue outward. This is just one weapon, and there are people who argue that you need to have thousands of these weapons.
Ariel: We all know, or at least have a rough idea, of how deadly nuclear weapons can be, but what is I think sometimes forgotten is that surviving a nuclear attack would also be devastating. I turned to some of the biologists that I spoke with to ask more about what the actual effects of radiation on the human body are.
Martin Chalfie: Radiation causes mutations, it causes breaks in DNA that sometimes cannot be repaired and that causes irreparable harm to cells, and it kills them. Radiation also causes other problems within cells simply by ionizing, and those ions cause problems within cells. That damage also makes cells die eventually, so it's exceptionally dangerous to cells and therefore to living beings.
Jonathan King: The first effect is on cells that have to reproduce at high frequency: blood cells. The blood cells' ability to reproduce gets killed and you become anemic. Then tissues that also have to reproduce a lot, like the skin, the ability of the skin to reproduce gets damaged. Wounds don't heal. Of course in a nuclear explosion, there's incredible temperatures, and so burns are horrendous, but the radiation further weakens the ability to respond to burns. Almost all those people would die. Then, there are the longer-term effects, which is the generation of cancers from different tissues. Those who survive the acute effects of radiation then suffer the longer-term effects, cancer being one of the most distinctive.
Martin Chalfie: Women, of course, produce all the eggs that they're going to produce before they're born, and as a result, if a woman is irradiated, that will of course affect the cells that are going to give rise to the eggs or the eggs themselves, and that would cause problems when that woman grows up and wants to have children.
Ariel: Given how deadly and devastating these weapons are, it's not surprising that politics seems to play a role in maintaining the current nuclear arsenals, but that's also precisely why many of these scientists are standing up for the ban.
Zia Mian: Nuclear weapons are fundamentally anti-democratic. No country with nuclear weapons has ever asked its people, "Do you want to be defended by the mass murder of people in other countries?" Because if you ask people this question, almost everybody would say, "No, I do not want you to incinerate entire cities and kill millions of women and children and innocent people to defend us." But they never asked this question, and so the role of scientists and other people is to ask people, "What kind of country do you want to be in? How do you want to be defended in an actually honest and accurate way?"
Jonathan King: Very few people realize that it's their tax dollars that pays for the development and maintenance of these weapons, billions and billions of dollars a year. The cost of one year of maintaining nuclear weapons is equivalent to the entire budget of the National Institute of Health responsible for research on all of the diseases that afflict Americans: heart disease, stroke, Alzheimer's, arthritis, diabetes. It's an incredible drain of national resources, and I'm hoping that bringing that to the attention of the public also will be important.
Max Tegmark: The key part of the problem is that most people I know think nuclear weapons are scary but kind of cool at the same time because they keep us safe, and that's just a myth.
Hans Kristensen: Nuclear weapons threaten everyone, and it's not just depending who you are. It's a threat to all human beings everywhere because even if you're not a direct target, if they were used in significant numbers, the dust and the pollution coming from the weapons used would cause climatic changes on a global scale. So this is an issue for all. Also in terms of the ban treaty itself, it's not about the North Koreans versus the Americans or the Americans versus the Russians. The ban would be for all, so it's not like one country would lose them. Everybody would lose them, so that's the really important part here. We're trying to get rid of nuclear weapons so there's no nuclear threat to anyone.
Frank von Hippel: My principal concern is that the danger is that they'll be used by accident as a result of false warning or even hacking. Now, we worry about hackers, hacking into the control system. At the moment, they're in a "launch on warning" posture. The US and Russia are sort of pointed at each other. That's an urgent problem, and we can't depend on luck indefinitely. I mean, I think one thing that scientists can offer is that they understand Murphy's Law, you know, "What can go wrong will go wrong." We really have to get on top of this. This problem did not go away with the end of the Cold War. I think, for most people it did, and now people sort of assume the danger went away, but the danger of accident was probably always the biggest danger, and it's still very much with us. I call it the "nuclear doomsday machine."
Elaine Scarry: My name is Elaine Scarry. I teach at Harvard University, and for at least 25 years, I've worked on the problem of nuclear weapons. People seem to understand that nuclear weapons are ungovernable in the sense that they're subject to many accidents. For example, a British and a French nuclear submarine collided under the ocean, or to take another example, the United States accidentally sent missile triggers to Taiwan when they meant to send helicopter batteries. There's a second way in which people recognize that nuclear weapons are ungovernable, and that is that they can be appropriated by terrorists or hackers or rogue states.
But my work is showing that there's a third, much deeper way in which nuclear weapon are ungovernable, because it's impossible for there to be any legitimate form of governance with them. What possible explanation could account for injuring many millions of people with a nuclear weapon? More important, what could possibly account for an architecture that allows a single person to bring about deaths and injury to many millions of people?
Max Tegmark: I think it's kind of insane that we have over seven billion people on this little spinning ball in space, none of whom wants to have a global nuclear war that perhaps kills most of us, and yet we might have one. So what can we do about it? I think we can stigmatize nuclear weapons.
Fabian Hamilton: My name's Fabian Hamilton. I'm a labor member of Parliament in the United Kingdom, Parliament in the House of Commons, and I'm the Shadow Minister for Peace and Disarmament. Well, I'm hopeful for a ban treaty that stigmatizes the ownership, development, and possession of nuclear weapons. Now, I am realistic enough to know that that isn't going to result in a disarmament overnight, but as we've seen with so many different things over the years – smoking is a good example. When you ban smoking in public places, you don't stop people smoking, but you stigmatize it so that people go outside to smoke, and they eventually say, "Well actually I'm trying to give up," and they often do give up.
Now, it's still a legal thing to smoke. It's not illegal. What we're trying to do here I think is to make nuclear weapons things that are illegal. Illegal to possess, illegal to develop, illegal to own, and certainly illegal to use. Now, that won't overnight get rid of all the nuclear weapons owned by the nine nuclear weapon states, but it will change the norms by which they act, and I think over time, and as we develop from this ban treaty to further treaties, it will push them into giving up those weapons. It's a long-term thing, but here is the most important first step: ban the weapons. Tell the entire world that these aren't acceptable.
Susi Snyder: Hi. My name is Susi Snyder. I work for Dutch Peace Organization called PAX. I'm also the author of the "Don't Bank On The Bomb Report." I'm here to talk about nuclear weapons. Nuclear weapons are an existential threat, something we should all be concerned about, but we can't let them make us feel like there's nothing we can do about them, because we can. You and I can do something to deal with nuclear weapons. Now, I'm going to make some suggestions on what you can do. First, support the majority of world's governments that are negotiating a new treaty to make nuclear weapons finally illegal. And come to the Women's March to Ban the Bomb on the 17th of June in New York City. You can find more information on WomenBanTheBomb.Org.
If you can't make it to the Women's March and you can't make it to another sister march, you can still do something great to get rid of nuclear weapons. What you can do is get in touch with your bank or your pension fund and ask them if they are investing in nuclear weapons, because most of these governments that are negotiating a new treaty, they recognize that any kind of assistance in having or making nuclear weapons includes financial assistance. They're going to make that illegal, so it's time for your bank to quit banking on the bomb, start banking on the ban. Get more information on DontBankOnTheBomb.com, and thank you for your time.
Related episodes
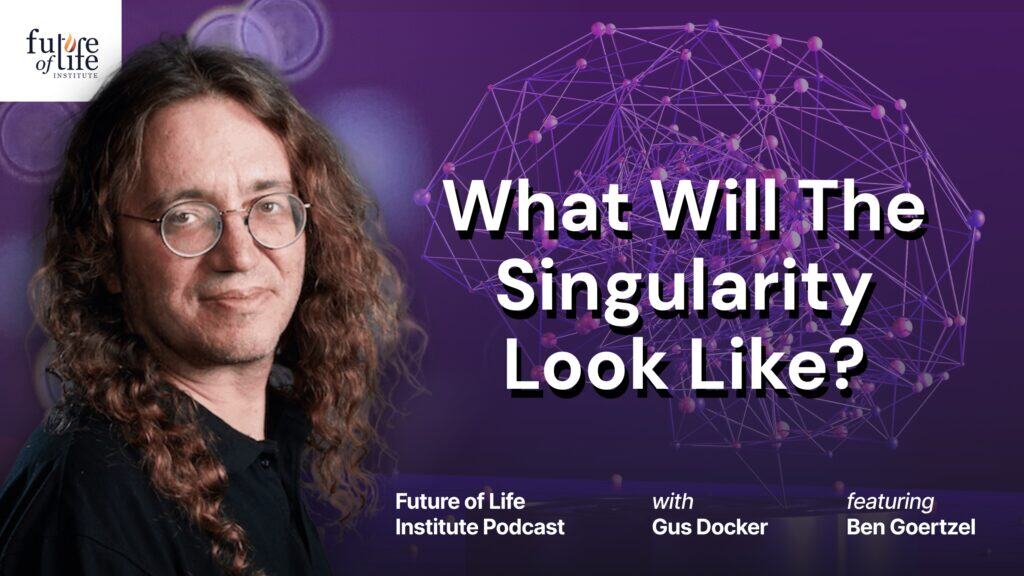