2015 AI Safety Grant Program
Grants archive
Project Summary
Many experts think that within a century, artificial intelligence will be able to do almost anything a human can do. This might mean humans are no longer in control of what happens, and very likely means they are no longer employable. The world might be very different, and the changes that take place could be dangerous.
Very little research has asked when this transition will happen, what will happen, and how we can make it go well. AI Impacts is a project to ask those questions, and to answer them rigorously. We look for research projects that can shed light on the future of AI; especially on questions that matter to people making decisions. We publish the results online, and explain our research to a broad audience.
We are currently working on comparing the power of the brain to that of supercomputers, to help calculate when people will have enough hardware to run something as complex as a brain. We are also checking whether AI progress is likely to see sudden jumps, by looking for jumps in other areas of technological progress.
Technical Abstract
‘Human-level’ artificial intelligence will have far-reaching effects on society, and is generally anticipated within the coming century. Relatively little is known about the timelines or consequences of this arrival, though increasingly many decisions depend on guesses about it. AI Impacts identifies cost-effective research projects which might shed light on the future of AI, and especially on the parts of it that might guide policy and other decisions. We perform a selection of these research projects, and publish the results as accessible articles in the public domain.
We recently made a preliminary estimate of the computing performance of the brain in terms of traversed edges per second (TEPS), “a supercomputing benchmark” to better judge when computing hardware will be capable of replicating what the brain does, given the right software. We are also collecting case studies of abrupt technological progress to aid in evaluating the probability of discontinuities in AI progress. In the coming year we will continue with both of these projects, publish articles about several projects in progress, and start several new projects.
Project Summary
How can we ensure that powerful AI systems of the future behave in ways that are reliably aligned with human interests?
One productive way to begin study of this AI alignment problem in advance is to build toy models of the unique safety challenges raised by such powerful AI systems and see how they behave, much as Konstantin Tsiolkovsky wrote down (in 1903) a toy model of how a multistage rocket could be launched into space. This enabled Tsiolkovsky and others to begin exploring the specific challenges of spaceflight long before such rockets were built.
Another productive way to study the AI alignment problem in advance is to seek formal foundations for the study of well-behaved powerful Ais, much as Tsiolkovsky derived the rocket equation (also in 1903) which governs the motion of rockets under ideal environmental conditions. This was a useful stepping stone toward studying the motion of rockets in actual environments.
We plan to build toy models and seek formal foundations for many aspects of the AI alignment problem. One example is that we aim to improve our toy models of a corrigible agent which avoids default rational incentives to resist its programmers’ attempts to fix errors in the AI’s goals.
Technical Abstract
The Future of Life Institute’s research priorities document calls for research focused on ensuring beneficial behavior in systems that can learn from experience with human-like breadth and surpass human performance in most cognitive tasks. We aim to study several sub-problems of this ‘AI alignment problem, by illuminating the key difficulties using toy models, and by seeking formal foundations for robustly beneficial intelligent agents. In particular, we hope to (a) improve our toy models of ‘corrigible agents’ which avoid default rational incentives to resist corrective interventions from the agents’ programmers, (b) continue our preliminary efforts to put formal foundations under the study of naturalistic, embedded agents which avoid the standard agent-environment split currently used as a simplifying assumption throughout the field of AI, and (c) continue our preliminary efforts to overcome obstacles to flexible cooperation in multi-agent settings. We also hope to take initial steps in formalizing several other informal problems related to AI alignment, for example the problem of ‘ontology identification’: Given goals specified with respect to some ontology and a world model, how can the ontology of the goals be identified inside the world model?
Project Summary
One path to significantly smarter-than-human artificial agents involves self-improvement, i.e., agents doing artificial intelligence research to make themselves even more capable. If such an agent is designed to be robust and beneficial, it should only execute self-modifying actions if it knows they are improvements, which, at a minimum, means being able to trust that the modified agent only takes safe actions. However, trusting the actions of a similar or smarter agent can lead to problems of self-reference, which can be seen as sophisticated versions of the liar paradox (which shows that the self-referential sentence “this sentence is false” cannot be consistently true or false). Several partial solutions to these problems have recently been proposed. However, current software for formal reasoning does not have sufficient support for self-referential reasoning to make these partial solutions easy to implement and study. In this project, we will implement a toy model of agents using these partial solutions to reason about self-modifications, in order to improve our understanding of the challenges of implementing self-referential reasoning, and to stimulate work on tools suitable for it.
Technical Abstract
Artificially intelligent agents designed to be highly reliable are likely to include a capacity for formal deductive reasoning to be applied in appropriate situations, such as when reasoning about computer programs including other agents and future versions of the same agent. However, it will not always be possible to model other agents precisely: considering more capable agents, only abstract reasoning about their architecture is possible. Abstract reasoning about the behavior of agents that justify their actions with proofs lead to problems of self-reference and reflection: Godel’s second incompleteness theorem shows that no sufficiently strong proof system can prove its own consistency, making it difficult for agents to show that actions their successors have proven to be safe are in fact safe (since an inconsistent proof system would be able to prove any action “safe”). Recently, some potential approaches to circumventing this obstacle have been proposed in the form of pen-and-paper proofs.
We propose building and studying implementations of agents using these approaches, to better understand the challenges of implementing tools that are able to support this type of reasoning, and to stimulate work in the interactive theorem proving community on this kind of tools.
Technical Abstract
We propose to hold a one-day summit (in spring 2017) at Washington, DC, on the subject of artificial intelligence (broadly conceived) and the future of work. The goal is to put this issue on the national agenda in an informed and deliberate manner rather than the typically-alarmist and over-the-top accounts disseminated by the mainstream media. The location is important to ensure attendance by policy makers and leaders of funding agencies. The summit will bring together leading technologists, economists, sociologists, and humanists, who will offer the views on where technology is going, what its impact may be, and what research issues are raised by these projections.
The summit will be sponsored by the Computing Research Association (CRA), whose Government Affairs Committee has extensive experience of reaching out to policy makers. We will also reach out to other relevant societies, such as US-ACM, and AAAS.
Project Summary
AI systems, whether robotic or conversational software agents, use planning algorithms to achieve high-level goals by exhaustively considering all possible sequences of actions. While these methods are increasingly powerful and can even generate seemly creative solutions, they have no understanding of ethics: they don’t understand harm nor can they distinguish between good and bad side effects of their actions. We propose to develop representations and algorithms fill this gap.
Technical Abstract
Recent advances in probabilistic planning and reinforcement learning have resulted in impressive performance at tasks as varied as mobile robotics, self-driving cars, and playing Atari video games. As these algorithms get deployed in real-world environments, it becomes critical to ensure that their utility-seeking behavior does not result in unintended, harmful side-effects. We need a way to specify a set of agent ethics: social norms that we can trust the agent will not knowingly violate. Developing mechanisms for defining and enforcing such ethical constraints requires innovations ranging from improved vocabulary grounding to more robust planning and reinforcement learning algorithms.
Project Summary
Driverless cars, service robots, surveillance drones, computer networks collecting data, and autonomous weapons are just a few examples of increasingly intelligent technologies scientists are developing. As they progress, researchers face a series of questions about whether these machines can be designed and engineered to take morally significant actions previously reserved for human actors. Can they ensure that artificially intelligent systems will always be demonstrably beneficial, safe, controllable, and sensitive to human values? Many individuals and groups have begun tackling the various subprojects entailed in this challenge. They are, however, often unaware of efforts in complementary fields. Thus they lose opportunities for creative collaboration, miss gaps in their own research, and reproduce work being performed by potential colleagues. The Hastings Center proposes to convene a series of three solution-directed workshops with national and international experts in the various pertinent fields. Together they will develop collaborative strategies and research projects, and forge an outline for a comprehensive plan to insure autonomous systems will be demonstrably beneficial, and that this innovative research progresses in a responsible manner. The results of the workshop will be conveyed through a special report, a dedicated edition of a scholarly journal, and two public symposia.
Technical Abstract
The vast array of challenges entailed in designing, engineering, and implementing demonstrably beneficial, safe and controllable AI systems are slowly being addressed by scholars working on distinct research trajectories across many disciplines. They are often unaware of efforts in complementary fields, thus losing opportunities for creative synergies, missing gaps in their own research, and reproducing the work of potential colleagues. The Hastings Center proposes to convene a series of three solution-directed workshops with national and international experts in the varied fields. Together they will address trans-disciplinary questions, develop collaborative strategies and research projects, and forge an outline for a comprehensive plan encompassing the many elements of ensuring autonomous systems will be demonstrably beneficial, and that this innovative research progresses in a responsible manner. The workshops’ research and policy agenda will be published as a Special Report of the journal Hastings Center Report and in short form in a science or engineering journal. Findings will also be presented through two public symposia, one of which will be webcast and available on demand. We anticipate significant progress given the high caliber of the people who are excited by this project and have already committed to join our workshops.
Project Summary
Autonomous goal-directed systems may behave flexibly with minimal human involvement. Unfortunately, such systems could also be dangerous if pursuing an incorrect or incomplete goal.
Meaningful human control can ensure that each decision ultimately reflects the desires of a human operator, with AI systems merely providing capabilities and advice. Unfortunately, as AI becomes more capable such control becomes increasingly limiting and expensive.
I propose to study an intermediate approach, where a system’s behavior is shaped by what a human operator would have done if they had been involved, rather than either requiring actual involvement or pursuing a goal without any oversight. This approach may be able to combine the safety of human control with the efficiency of autonomous operation. But capturing either of these benefits requires confronting new challenges: to be safe, we must ensure that our AI systems do not cause harm by incorrectly predicting the human operator; to be efficient and flexible, we must enable the human operator to provide meaningful oversight in domains that are too complex for them to reason about unaided. This project will study both of these problems, with the goal of designing concrete mechanisms that can realize the promise of this approach.
Project Summary
What are the most important projects for reducing the risk of harm from superintelligent artificial intelligence? We will probably not have to deal with such systems for many years – and we do not expect they will be developed with the same architectures we use today. That may make us want to focus on developing long-term capabilities in AI safety research. On the other hand, there are forces pushing us towards working on near-term problems. We suffer from ‘near-sightedness’ and are better at finding the answer to questions that are close at hand. Just as important, work on long-term problems can happen in the future and get extra people attending to it, while work on near-term problems has to happen now if it is to happen at all.
This project models the trade-offs we make when carrying out AI safety projects that aim at various horizons, and focused on specific architectures. It estimates crucial parameters – like the time-horizon probability distribution and how near-sighted we tend to be. It uses that model to work out what the AI safety community should be funding, and what it should call on policymakers to do.
Technical Abstract
The advent of human-level artificial intelligence (HLAI) would pose a challenge for society. The most cost-effective work on this challenge depends on the time at which we achieve HLAI, on the architecture which produces HLAI, and on whether the first HLAI is likely to be rapidly superseded. For example, direct work on safety issues is preferable if we will achieve HLAI soon, while theoretical work and capability building is important for more distant scenarios.
This project develops a model for the marginal cost-effectiveness of extra resources in AI safety. The model accounts for uncertainty over scenarios and over work aimed at those scenarios, and for diminishing marginal returns for work. A major part of the project is parameter estimation. We will estimate key parameters based on existing work where possible (timeline probability distributions), new work (‘near-sightedness’, using historical predictions of mitigation strategies for coming challenges), and expert elicitation, and combine these into a joint probability distribution representing our current best understanding of the likelihood of different scenarios. The project will then make recommendations for the AI safety community, and for policymakers, on prioritising between types of AI safety work.
Project Summary
One goal of artificial intelligence is valid behavior: computers should perform tasks that people actually want them to do. The current model of programming hinders validity, largely because it focuses on the minutae of how to compute rather than the goal of what to compute. An alternative model offers hope for validity: program synthesis. Here, the user specifies what by giving a small description of their goal (e.g., input-output examples). The synthesizer then infers candidate programs matching that description, which the user selects from.
One shortcoming of synthesizers is that they are truthful rather than helpful: they return answers that are literally consistent with user requirements but no more (e.g., a requirement of “word that starts with the letter a” might return just “a”). By contrast, human read more deeply into requirements, divining the underlying intentions. Helpfulness of this kind has been intensely studied in the linguistic field called pragmatics. This project will investigate how recent developments into computational modeling of pragmatics can be leveraged to improve program synthesis, making it easier to write programs that do what we want with little to no special knowledge.
Technical Abstract
One goal of artificial intelligence is valid behavior: computers should perform tasks that people actually want them to do. The current model of programming hinders validity, largely because it focuses on the minutae of how to compute rather than the goal of what to compute. An alternative model offers hope for validity: program synthesis. Here, the user specifies what by giving a small description of their goal (e.g., input-output examples). The synthesizer then infers
candidate programs matching that description, which the user selects from. One shortcoming of synthesizers is that they are truthful rather than helpful: they return answers that are literally consistent with user requirements but no more (e.g., a requirement of “word that starts with the letter A” might return just “a”). By contrast, human read more deeply into requirements, divining the underlying intentions. Recent work in computational psycholinguistics that we can capture this ability through user modeling — maintaining a model of how the user purposefully selects examples to convey information. This project will investigate how these psycholinguistic insights can be used to make synthesis more valid.
Project Summary
Some experts believe that computers could eventually become a lot smarter than humans are. They call it artificial superintelligence, or ASI. If people build ASI, it could be either very good or very bad for humanity. However, ASI is not well understood, which makes it difficult for people to act to enable good ASI and avoid bad ASI. Our project studies the ways that people could build ASI in order to help people act in better ways. We will model the different steps that need to occur for people to build ASI. We will estimate how likely it is that these steps will occur, and when they might occur. We will also model the actions people can take, and we will calculate how much the actions will help. For example, governments may be able to require that ASI researchers build in safety measures. Our models will include both the government action and the ASI safety measures, to learn about how well it all works. This project is an important step towards making sure that humanity avoids bad ASI and, if it wishes, creates good ASI.
Technical Abstract
Artificial superintelligence (ASI) has been proposed to be a major transformative future technology, potentially resulting in either massive improvement in the human condition or existential catastrophe. However, the opportunities and risks remain poorly characterized and quantified. This reduces the effectiveness of efforts to steer ASI development towards beneficial outcomes and away from harmful outcomes. While deep uncertainty inevitably surrounds such a breakthrough future technology, significant progress can be made now using available information and methods. We propose to model the human process of developing ASI. ASI would ultimately be a human creation; modeling this process indicates the probability of various ASI outcomes and illuminates a range of ways to improve outcomes. We will characterize the development pathways that can result in beneficial or dangerous ASI outcomes. We will apply risk analysis and decision analysis methods to quantify opportunities and risks, and to evaluate opportunities to make ASI less risky and more beneficial. Specifically, we will use fault trees and influence diagrams to map out ASI development pathways and the influence that various actions have on these pathways. Our proposed project will produce the first-ever analysis of ASI development using rigorous risk and decision analysis methodology.
Project Summary
As it becomes ever clearer how machines with a human level of intelligence can be built — and indeed that they will be built — there is a pressing need to discover ways to ensure that such machines will robustly remain benevolent, especially as their intellectual and practical capabilities come to surpass ours. Through self-modification, highly intelligent machines may be capable of breaking important constraints imposed initially by their human designers. The currently prevailing technique for studying the conditions for preventing this danger is based on forming mathematical proofs about the behavior of machines under various constraints. However, this technique suffers from inherent paradoxes and requires unrealistic assumptions about our world, thus not proving much at all.
Recently a class of machines that we call experience-based artificial intelligence (EXPAI) has emerged, enabling us to approach the challenge of ensuring robust benevolence from a promising new angle. This approach is based on studying how a machine’s intellectual growth can be molded over time, as the machine accumulates real-world experience, and putting the machine under pressure to test how it handles the struggle to adhere to imposed constraints.
The Swiss AI lab IDSIA will deliver a widely applicable EXPAI growth control methodology.
Technical Abstract
Whenever one wants to verify that a recursively self-improving system will robustly remain benevolent, the prevailing tendency is to look towards formal proof techniques, which however have several issues: (1) Proofs rely on idealized assumptions that inaccurately and incompletely describe the real world and the constraints we mean to impose. (2) Proof-based self-modifying systems run into logical obstacles due to Lob’s theorem, causing them to progressively lose trust in future selves or offspring. (3) Finding nontrivial candidates for provably beneficial self-modifications requires either tremendous foresight or intractable search.
Recently a class of AGI-aspiring systems that we call experience-based AI (EXPAI) has emerged, which fix/circumvent/trivialize these issue. They are self-improving systems that make tentative, additive, reversible, very fine-grained modifications, without prior self-reasoning; instead, self-modifications are tested over time against experiential evidences and slowly phased in when vindicated or dismissed when falsified. We expect EXPAI to have high impact due to its practicality and tractability. Therefore we must now study how EXPAI implementations can be molded and tested during their early growth period to ensure their robust adherence to benevolence constraints.
In this project, the Swiss AI lab IDSIA will deliver an EXPAI growth control methodology that shall be widely applicable.
Project Summary
We focus on current and future complex AI autonomous systems that integrate sensors, computation, and actuation to perform tasks of benefit to humans. Examples of such systems are auto-pilots, medical assistants, internet-of-things components, and mobile service robots. One of the key aspects to bring such complex AI systems to safe and acceptable existence is the ability for such systems to provide transparency on their representations, interpretations, choices, and decisions, in summary, their internal state.
We believe that, to build AI systems that are safe, as well as accepted and trusted by humans, we need to equip them with the capability to explain their actions, recommendations, and inferences. Our proposed project aims at researching on the specification, formalization, and generation of explanations, with a concrete focus on seamlessly integrated AI systems that sense and reason about multi-modal information in symbiosis with humans. As a result, humans will be able to query robots for explanations about their recommendations or actions, and carry any needed corrections.
Technical Abstract
AI systems have long been challenged with providing explanations about their reasoning. Automated theorem provers, explanation-based learning systems, and conflict-based constraint solvers are examples where inference is supplemented by the underlying processed knowledge and rules.
We focus on current and future complex AI autonomous systems that integrate perception, cognition, and action, in tasks to service humans. These systems can be viewed as cyber-physical-social systems, such as auto-pilots, medical assistants, internet-of-things components, and mobile service robots.
We propose to research on bringing such complex AI systems to safe and acceptable existence by providing transparency on their representations, interpretations, choices, and decisions. We will develop mining techniques to enable the analysis and explanation of temporally-logged sensory and execution data, constrained by the underlying behavior architecture, as well as the uncertainty of the sensed environment. We will address the need for probabilistic and knowledge-based inference; the variety of input data modalities; and the coordination of multiple reasoning agents.
We will concretely research on autonomous mobile service robots, such as CoBots, as well as quadrotors. We envision humans setting queries about the robots performance and the choice of their actions. Our generated explanations will increase the understanding, and robot safety.
Project Summary
Humans take great pride in being the only creatures who make moral judgments, even though their moral judgments often suffer from serious flaws. Some AI systems do generate decisions based on their consequences, but consequences are not all there is to morality. Moral judgments are also affected by rights (such as privacy), roles (such as in families), past actions (such as promises), motives and intentions, and other morally relevant features. These diverse factors have not yet been built into AI systems. Our goal is to do just that. Our team plans to combine methods from computer science, philosophy, and psychology in order to construct an AI system that is capable of making plausible moral judgments and decisions in realistic scenarios. We hope that this work will provide a basis that leads to future highly-advanced AI systems acting ethically and thereby being more robust and beneficial. Humans, by comparing their own moral judgments to the output of the resulting system, will be able to understand their own moral judgments and avoid common mistakes (such as partiality and overlooking relevant factors). In these ways and more, moral AI might also make humans more moral.
Technical Abstract
Most contemporary AI systems base their decisions solely on consequences, whereas humans also consider other morally relevant factors, including rights (such as privacy), roles (such as in families), past actions (such as promises), motives and intentions, and so on. Our goal is to build these additional morally relevant features into an AI system. We will identify morally relevant features by reviewing theories in moral philosophy, conducting surveys in moral psychology, and using machine learning to locate factors that affect human moral judgments. We will use and extend game theory and social choice theory to determine how to make these features more precise, how to weigh conflicting features against each other, and how to build these features into an AI system. We hope that eventually this work will lead to highly advanced AI systems that are capable of making moral judgments and acting on them. Humans will then be able to compare these outputs to their own moral judgments in order to learn which of these judgments are distorted by biases, partiality, or lack of attention to relevant factors. In such ways, moral AI can also contribute to our own understanding of morality and our moral lives.
Project Summary
Previous work in economics and AI has developed mathematical models of preferences or values, along with computer algorithms for inferring preferences from observed human choices. We would like to use such algorithms to enable AI systems to learn human preferences by observing humans make real-world choices. However, these algorithms rely on an assumption that humans make optimal plans and take optimal actions in all circumstances. This is typically false for humans. For example, people’s route planning is often worse than Google Maps, because we can’t number-crunch as many possible paths. Humans can also be inconsistent over time, as we see in procrastination and impulsive behavior. Our project seeks to develop algorithms that learn human preferences from data despite humans not being homo-economicus and despite the influence of non-rational impulses. We will test our algorithms on real-world data and compare their inferences to people’s own judgments about their preferences. We will also investigate the theoretical question of whether this approach could enable an AI to learn the entirety of human values.
Technical Abstract
Previous work in economics and AI has developed mathematical models of preferences, along with algorithms for inferring preferences from observed actions. We would like to use such algorithms to enable AI systems to learn human preferences from observed actions. However, these algorithms typically assume that agents take actions that maximize expected utility given their preferences. This assumption of optimality is false for humans in real-world domains. Optimal sequential planning is intractable in complex environments and humans perform very rough approximations. Humans often don’t know the causal structure of their environment (in contrast to MDP models). Humans are also subject to dynamic inconsistencies, as observed in procrastination, addiction and in impulsive behavior. Our project seeks to develop algorithms that learn human preferences from data despite the suboptimality of humans and the behavioral biases that influence human choice. We will test our algorithms on real-world data and compare their inferences to people’s own judgments about their preferences. We will also investigate the theoretical question of whether this approach could enable an AI to learn the entirety of human values.
Project Summary
We are unsure about what moral system is best for humans, let alone for potentially super-intelligent machines. It is likely that we shall need to create artificially intelligent agents to provide moral guidance and police issues of appropriate ethical values and best practice, yet this poses significant challenges. Here we propose an initial evaluation of the strengths and weaknesses of one avenue by investigating self-policing intelligent agents. We shall explore two themes: (i) adding a layer of AI agents whose express purpose is to police other AI agents and report unusual or undesirable activity (potentially this might involve setting traps to catch misbehaving agents, and may consider if it is wise to allow policing agents to take corrective action against offending agents); and (ii) analyzing simple models of evolving adaptive agents to see if robust conclusions can be learned. We aim to survey related literature, identify key areas of hope and concern for future investigation, and obtain preliminary results for possible guarantees. The proposal is for a one year term to explore the ideas and build initial models, which will be made publicly available, ideally in journals or at conferences or workshops, with extensions likely if progress is promising.
Project Summary
Economics models the behavior of people, firms, and other decision makers, as a means to understand how these decisions shape the pattern of activities that produce value and ultimately satisfy (or fail to satisfy) human needs and desires. The field adopts rational models of behavior, either of individuals or of behavior in the aggregate.
Artificial Intelligence (AI) research is also drawn to rationality concepts, which provide an ideal for the computational agents that it seeks to create. Although perfect rationality is not achievable, the capabilities of AI are rapidly advancing, and AI can already surpass human-level capabilities in narrow domains.
We envision a future with a massive number of AIs, these AIs owned, operated, designed, and deployed by a diverse array of entitites. This multiplicity of interacting AIs, apart or together with people, will constitute a social system, and as such economics can provide a useful framework for understanding and influencing the aggregate. In turn, systems populated by AIs can benefit from explicit design of the frameworks within which AIs exist. The proposed research looks to apply the economic theory of mechanism design to the coordination of behavior in systems of multiple AIs, looking to promote beneficial outcomes.
Technical Abstract
When a massive number of AIs are owned, operated, designed, and deployed by a diverse array of firms, individuals, and governments, this multi-agent AI constitutes a social system, and economics provides a useful framework for understanding and influencing the aggregate. In particular, we need to understand how to design multi-agent systems that promote beneficial outcomes when AIs interact with each other. A successful theory must consider both incentives and privacy considerations.
Mechanism design theory from economics provides a framework for the coordination of behavior, such that desirable outcomes are promoted and less desirable outcomes made less likely because they are not in the self-interest of individual actors. We propose a program of fundamental research to understand the role of mechanism design, multi-agent dynamical models, and privacy-preserving algorithms, especially in the context of multi-agent systems in which the AIs are built through reinforcement learning (RL). The proposed research considers two concrete AI problems: the first is experiment design, typically formalized as a multi-armed bandit process, which we study in a multi-agent, privacy-preserving setting. The second is the more general problem of learning to act in Markovian dynamical systems, including both planning and RL agents.
Project Summary
Progress towards a fully-automated economy suffers from a profound tension. On the one hand, technological progress depends on human effort. Human effort is, in general, decreasing in the amount that effort is taxed. On the other hand, the more the economy is automated, the more redistribution could be required to support the living standards of the less skilled. The less skilled could even become unemployed, and the unemployed could eventually comprise the majority of the population. The higher the fraction unemployed, the higher must be the tax burden on those who are productive in this new economy.
At first glance, then, the more technological progress we make, the more we will be forced to disincentivize further progress. Yet, it is possible that some paths of tax and subsidy policy could lead to vastly improved social welfare a few decades hence compared to others. Some paths might avoid altogether the scenario sketched above. This project seeks to characterize the path of optimal policy in the transition to a fully-automated economy. In doing so, it would answer directly the question of how we maximize the societal benefit of AI.
Project Summary
In order for AI to be safely deployed, the desired behavior of the AI system needs to be based on well-understood, realistic, and empirically testable assumptions. From the perspective of modern machine learning, there are three main barriers to this goal. First, existing theory and algorithms mainly focus on fitting the observable outputs in the training data, which could lead, for instance, to an autonomous driving system that performs well on validation tests but does not understand the human values underlying the desired outputs. Second, existing methods are designed to handle a single specified set of testing conditions, and thus little can be said about how a system will behave in a fundamentally new setting; e.g., an autonomous driving system that performs well in most conditions may still perform arbitrarily poorly during natural disasters. Finally, most systems have no way of detecting whether their underlying assumptions have been violated: they will happily continue to predict and act even on inputs that are completely outside the scope of the system.
In this proposal, we detail a research program for addressing all three of the problems above. Just as statistical learning theory (e.g., the work of Vapnik) laid down the foundations of existing machine learning and AI techniques, allowing the field to flourish over the last 25 years, we aim to lay the groundwork for a new generation of safe-by-design AI systems, which can sustain the continued deployment of AI in society.
Technical Abstract
With the pervasive deployment of machine learning algorithms in mission-critical AI systems, it is imperative to ensure that these algorithms behave predictably in the wild. Current machine learning algorithms rely on a tacit assumption that training and test conditions are similar, an assumption that is often violated due to changes in user preferences, blacking out of sensors, etc. Worse, these failures are often silent and difficult to diagnose. We propose to develop a new generation of machine learning algorithms that come with strong static and dynamic guarantees necessary for safe deployment in open-domain settings. Our proposal focuses on three key thrusts: robustness to context change, inferring the underlying process from partial supervision, and failure detection at execution time. First, rather than learning models that predict accurately on a target distribution, we will use minimax optimization to learn models that are suitable for any target distribution within a “safe” family. Second, while existing learning algorithms can fit the input-output behavior from one domain, they often fail to learn the underlying reason for making certain predictions; we address this with moment-based algorithms for learning latent-variable models, with a novel focus on structural properties and global guarantees. Finally, we propose using dynamic testing to detect when the assumptions underlying either of these methods fail, and trigger a reasonable fallback. With these three points, we aim to lay down a framework for machine learning algorithms that work reliably and fail gracefully.
Project Summary
For society to enjoy many of the benefits of advanced artificial intelligence (AI) and robotics, it will be necessary to deal with situations that arise in which autonomous artificial agents violate laws or cause harm. If we want to allow AIs and robots to roam the internet and the physical world and take actions that are unsupervised by humans — as may be necessary for, e.g. personal shopping assistants, self-driving cars, and host of other applications — we must be able to manage the liability for the harms they might cause to individuals and property. Resolving this issue will require untangling a set of theoretical and philosophical issues surrounding causation, intention, agency, responsibility, culpability and compensation, and distinguishing different varieties of agency, such as causal, legal and moral. With a clearer understanding of the central concepts and issues, this project will provide a better foundation for developing policies which will enable society to utilize artificial agents as they become increasingly autonomous, and ensuring that future artificial agents can be both robust and beneficial to society, without stifling innovation.
Technical Abstract
This project addresses a central issue — “the liability problem” — facing the regulation of artificial computational agents, including artificial intelligence (AI) and robotic systems, as they become increasingly autonomous, and supersede current capabilities. In order for society to benefit from advances in AI technology, it will be necessary to develop regulatory policies which manage the risk and liability of deploying systems with increasingly autonomous capabilities. However, current approaches to liability have difficulties when it comes to dealing with autonomous artificial agents because their behavior may be unpredictable to those who create and deploy them, and they will not be proper legal agents. The project will explore the fundamental concepts of autonomy, agency and liability; clarify the different varieties of agency that artificial systems might realize, including causal, legal and moral; and the illuminate the relationships between these. The project will take a systematic approach by integrating an analysis of fundamental concepts “including autonomy, agency, causation, intention, responsibility and culpability” and their applicability to autonomous artificial agents, surveying current legal approaches to liability, and exploring possible approaches for future regulatory policy. It will deliver a book-length publication containing the theoretical research results and recommendations for policy-making.
Project Summary
In the early days of AI research, scientists studied problems such as chess and theorem proving that involved “micro worlds” that were perfectly known and predictable. Since the 1980s, AI researchers have studied problems involving uncertainty. They apply probability theory to model uncertainty about the world and use decision theory to represent the utility of the possible outcomes of proposed actions. This allows computers to make decisions that maximize expected utility by taking into account the “known unknowns”. However, when such AI systems are deployed in the real world, they can easily be confused by “unknown unknowns” and make poor decisions. This project will develop theoretical principles and AI algorithms for learning and acting safely in the presence of unknown unknowns. The algorithms will be able to detect and respond to unexpected changes in the world. They will ensure that when the AI system plans a sequence of actions, it takes into account its ignorance of the unknown unknowns. This will lead it to behave cautiously and turn to humans for help. Instead of maximizing expected utility, it will first ensure that its actions avoid unsafe outcomes and only then maximize utility. This will make AI systems much safer.
Technical Abstract
The development of AI technology has progressed from working with “known knowns”—AI planning and problem solving in deterministic, closed worlds—to working with “known unknowns”—planning and learning in uncertain environments based on probabilistic models of those environments. A critical challenge for future AI systems is to behave safely and conservatively in open worlds, where most aspects of the environment are not modeled by the AI agent—the “unknown unknowns”. Our team, with deep experience in machine learning, probabilistic modeling, and planning, will develop principles, evaluation methodologies, and algorithms for learning and acting safely in the presence of the unknown unknowns. For supervised learning, we will develop UU-conformal prediction algorithms that extend conformal prediction to incorporate nonconformity scores based on robust anomaly detection algorithms. This will enable supervised learners to behave safely in the presence of novel classes and arbitrary changes in the input distribution. For reinforcement learning, we will develop UU-sensitive algorithms that act to minimize risk due to unknown unknowns. A key principle is that AI systems must broaden the set of variables that they consider to include as many variables as possible in order to detect anomalous data points and unknown side-effects of actions.
Project Summary
As we close the loop between sensing-reasoning-acting, autonomous agents such as self-driving cars are required to act intelligently and adaptively in increasingly complex and uncertain real-world environments. To make sensible decisions under uncertainty, agents need to reason probabilistically about their environments, e.g., estimate the probability that a pedestrian will cross or that a car will change lane. Over the past decades, AI research has made tremendous progress in automated reasoning. Existing technology achieves super-human performance in numerous domains, including chess-playing and crossword-solving. Unfortunately, current approaches do not provide worst-case guarantees on the quality of the results obtained. For example, it is not possible to rule out completely unexpected behaviors or catastrophic failures. Therefore, we propose to develop novel reasoning technology focusing on soundness and robustness. This research will greatly improve the reliability and safety of next-generation autonomous agents.
Technical Abstract
To cope with the uncertainty and ambiguity of real world domains, modern AI systems rely heavily on statistical approaches and probabilistic modeling. Intelligent autonomous agents need to solve numerous probabilistic reasoning tasks, ranging from probabilistic inference to stochastic planning problems. Safety and reliability depend crucially on having both accurate models and sound reasoning techniques. To date, there are two main paradigms for probabilistic reasoning: exact decomposition-based techniques and approximate methods such as variational and MCMC sampling. Neither of them is suitable for supporting autonomous agents interacting with complex environments safely and reliably. Decomposition-based techniques are accurate but are not scalable. Approximate techniques are more scalable, but in most cases do not provide formal guarantees on the accuracy. We therefore propose to develop probabilistic reasoning technology which is both scalable and provides formal guarantees, i.e., “certificates” of accuracy, as in formal verification. This research will bridge probabilistic and deterministic reasoning, drawing from their respective strengths, and has the potential to greatly improve the reliability and safety of AI and cyber-physical systems.
Project Summary
There is general consensus within the AI research community that progress in the field is accelerating: it is believed that human-level AI will be reached within the next one or two decades. A key question is whether these advances will accelerate further after general human level AI is achieved, and, if so, how rapidly the next level of AI systems ('super-human') will be achieved.
Since the mid 1970s, Computer scientists have developed a rich theory about the computational resources that are needed to solve a wide range of problems. We will use these methods to make predictions about the feasibility of super-human level cognition.
Technical Abstract
There is general consensus within the AI research community that progress in the field is accelerating: it is believed that human-level AI will be reached within the next one or two decades on a range of cognitive tasks. A key question is whether these advances will accelerate further after general human level AI is achieved, and, if so, how rapidly the next level of AI systems (‘super-human’) will be achieved. Having a better understanding of how rapidly we may reach this next phase will be useful in preparing for the advent of such systems.
Computational complexity theory provides key insights into the scalability of computational systems. We will use methods from complexity theory to analyze the possibility of the scale-up to super-human intelligence and the speed of such scale-up for different categories of cognition.
Project Summary
Machine Learning and Artificial Intelligence underpin technologies that we rely on daily, from consumer electronics (smart phones), medical implants (continuous blood glucose monitors), websites (Facebook, Google), to the systems that defend critical infrastructure. The very characteristic that makes these systems so beneficial — adaptability — can also be exploited by sophisticated adversaries wishing to breach system security or gain an economic advantage. This project will develop usable software tools for evaluating vulnerabilities in learning systems, a first step towards general-purpose, secure machine learning.
Technical Abstract
This project aims to develop systems for the analysis of machine learning algorithms in adversarial environments. Today Machine Learning and Statistics are employed in many technologies where participants have an incentive to game the system, for example internet ad placement, cybersecurity, credit risk in finance, health analytics, and smart utility grids. However little is known about how well state-of-the-art inference techniques fare when data is manipulated by a malicious adversary. By formulating the process of evading a learned model, or manipulating training data to poison learning, as an optimization program, our approach to evaluating security reduces to one a projected subgradient descent. Our main method for solving such iterative optimizations generically, will be to employ the dynamic code analysis represented by automatic differentiation. A key output of this project will be usable software tools for evaluating the security of learning systems in general.
Technical Abstract
It is crucial for AI researchers to be able to reason carefully about the potential risks of AI, and about how to maximize the odds that any superintelligence that develops remains aligned with human values (in what the Future of Life Institute refers to as the “AI alignment problem”).
Unfortunately, cognitive science research has demonstrated that even very high-IQ humans are subject to many biases that are especially likely to impact their judgment on AI alignment. Leaders in the nascent field of AI alignment have found that a deep familiarity with cognitive bias research, and practice overcoming those biases, has been crucial to progress in the field.
We therefore propose to help spread key reasoning skills and community norms throughout the AI community, via the following:
- In 2016, we will hold a workshop for 45 of the most promising AI students (graduate, undergraduate, and postdocs), in which we train them in the thinking skills most relevant to AI alignment.
- We will maintain contact with AI students after the workshop, helping them to stay in contact with the alignment issue and collaborate with each other to spread useful skills throughout the community and discover new ones themselves.
Project Summary
We are investigating the safety of possible future advanced AI that uses the same basic approach to motivated behavior as that used by the human brain. Neuroscience has given us a rough blueprint of how the brain directs its behavior based on its innate motivations and its learned goals and values. This blueprint may be used to guide advances in artificial intelligence to produce AI that is as intelligent and capable as humans, and soon after, more intelligent. While it is impossible to predict how long this progress might take, it is also impossible to predict how quickly it might happen. Rapidly progress in practical applications is producing rapid increases in funding from commercial and governmental sources. Thus, it seems critical to understand the potential risks of brain-style artificial intelligence before it is actually achieved. We are testing our model of brain-style motivational systems in a highly simplified environment, to investigate how its behavior may change as it learns and becomes more intelligent. While our system is not capable of performing useful tasks, it serves to investigate the stability of such systems when they are integrated with powerful learning systems currently being developed and deployed.
Technical Abstract
We apply a neural network model of human motivated decision-making to an investigation of the risks involved in creating artificial intelligence with a brain-style motivational system. This model uses relatively simple principles to produce complex, goal-directed behavior. Because of the potential utility of such a system, we believe that this approach may see common adoption, and has significant risks. Such a system could provide the motivational core of efforts to create artificial general intelligence (AGI). Such a system has the advantage of leveraging the wealth of knowledge already available and rapidly accumulating on the neuroscience of mammalian motivation and self-directed learning. We employ this model, and non-biological variations on it, to investigate the risks of employing such systems in combination with powerful learning mechanisms that are currently being developed. We investigate the issues of motivational and representational drift. Motivational drift captures how a system will change the motivations it is initially given and trained on. Representational drift refers to the possibility that sensory and conceptual representations will change over the course of training. We investigate whether learning in these systems can be used to produce a system that remains stable and safe for humans as it develops greater intelligence.
Technical Abstract
We propose the creation of a joint Oxford-Cambridge research center, which will develop policies to be enacted by governments, industry leaders, and others in order to minimize risks and maximize benefit from artificial intelligence (AI) development in the longer term. The center will focus explicitly on the long-term impacts of AI, the strategic implications of powerful AI systems as they come to exceed human capabilities in most domains of interest, and the policy responses that could best be used to mitigate the potential risks of this technology.
There are reasons to believe that unregulated and unconstrained development could incur significant dangers, both from “bad actors” like irresponsible governments, and from the unprecedented capability of the technology itself. For past high-impact technologies (e.g. nuclear fission), policy has often followed implementation, giving rise to catastrophic risks. It is important to avoid this with superintelligence: safety strategies, which may require decades to implement, must be developed before broadly superhuman, general-purpose AI becomes feasible.
This center represents a step change in technology policy: a comprehensive initiative to formulate, analyze, and test policy and regulatory approaches for a transformative technology in advance of its creation.
Technical Abstract
The impact of AI on society depends not only on the technical state of AI research, but also its sociological state. Thus, in addition to current AI safety research, we must also ensure that the next generation of AI researchers is composed of thoughtful, intelligent, safety-conscious individuals. The more the AI community as a whole consists of such skilled, broad-minded reasoners, the more likely AI is to be developed in a safe and beneficial manner.
Therefore, we propose running a summer program for extraordinarily gifted high school students (such as competitors from the International Mathematics Olympiad), with an emphasis on artificial intelligence, cognitive debiasing, and choosing a high-positive-impact career path, including AI safety research as a primary consideration. Many of our classes will be about AI and related technical areas, with two classes specifically about the impacts of AI on society.
Project Summary
AI systems will need to understand human values in order to respect them. This requires having similar concepts as humans do. We will research whether AI systems can be made to learn their concepts in the same way as humans learn theirs. This will involve a literature review of the relevant fields, as well as experimental work.
We are particularly interested in a branch of machine learning called deep learning. The concepts learned by deep learning agents seem to be similar as the ones that have been documented in psychology. We will attempt to apply existing deep learning methodologies for learning what we call moral concepts, concepts through which moral values are defined. In addition, we will investigate a particular hypothesis of how we develop our concepts and values in the first place.
Technical Abstract
Autonomous AI systems will need to understand human values in order to respect them. This requires having similar concepts as humans do. We will research whether AI systems can be made to learn their concepts in the same way as humans learn theirs. This will involve a literature review of the relevant fields, as well as experimental work.
Both human concepts and the representations of deep learning models seem to involve a hierarchical structure, among other similarities. For this reason, we will attempt to apply existing deep learning methodologies for learning what we call moral concepts, concepts through which moral values are defined. In addition, we will investigate the extent to which reinforcement learning affects the development of our concepts and values.
Project Summary
Codes of ethics play an important role in many sciences. Such codes aim to provide a framework within which researchers can understand and anticipate the possible ethical issues that their research might raise, and to provide guidelines about what is, and is not, regarded as ethical behaviour. In the medical sciences, for example, codes of ethics are fundamentally embedded within the research culture of the discipline, and explicit consideration of ethical issues is a standard expectation when research projects are planned and undertaken. In this project, we aim to start developing a code of ethics for AI research by learning from this interdisciplinary experience and extending its lessons into new areas. The project will bring together three Oxford researchers with expertise in artificial intelligence, philosophy, and applied ethics.
Technical Abstract
Codes of ethics play an important role in many sciences. Such codes aim to provide a framework within which researchers can understand and anticipate the possible ethical issues that their research might raise, and to provide guidelines about what is, and is not, regarded as ethical behaviour. In the medical sciences, especially, codes of ethics are fundamentally embedded within the research culture, and explicit consideration of ethical issues is a standard expectation when research projects are planned and undertaken. The aim of this project is to develop a solid basis for a code of artificial intelligence (AI) research ethics, learning from the scientific and medical community’s experience with existing ethical codes, and extending its lessons into three important and representative areas where artificial intelligence comes into contact with ethical concerns: AI in medicine and biomedical technology, autonomous vehicles, and automated trading agents. We will also explore whether the design of ethical research codes might usefully anticipate, and potentially ameliorate, the risks of future research into superintelligence. The project brings together three Oxford researchers with highly relevant expertise in artificial intelligence, philosophy, and applied ethics, and will also draw strongly on other research activity within the University of Oxford.
Project Summary
“I don’t know” is a safe and appropriate answer that people provide to many posed questions. To appropriately act in a variety of complex tasks, our artificial intelligence systems should incorporate similar levels of uncertainty. Instead, state-of-the-art statistical models and algorithms that enable computer systems to answer such questions based on previous experience often produce overly confident answers. Due to widely used modeling assumptions, this is particularly true when new questions come from situations that differ substantially from previous experience. In other words, exactly when human-level intelligence provides less certainty when generalizing from the known to the unknown, artificial intelligence tends to provide more. Rather than trying to engineer fixes to this phenomenon into existing methods, We propose a more pessimistic approach based on the question: “What is the worst-case possible for predictive data that still matches with previous experiences (observations)?” We propose to analyze the theoretical benefits of this approach and demonstrate its applied benefits on prediction tasks.
Technical Abstract
Reliable inductive reasoning that uses previous experiences to make predictions of unseen information in new situations is a key requirement for enabling useful artificial intelligence systems.
Tasks ranging over recognizing objects in camera images, predicting the outcomes of possible autonomous system controls, and understanding the intentions of other intelligent entities each depend on this type of reasoning. Unfortunately, existing techniques produce significant unforeseen errors when the underlying statistical assumptions they are based upon do not hold in reality. The nearly ubiquitous assumption that estimated relationships in future situations will be similar to previous experiences (i.e., past and future data is assumed to be exchangeable or independent and identically distributed–IID–according to a common distribution) is particularly brittle when employed within artificial intelligence systems that autonomously interact with the physical world. We propose an adversarial formulation for cost-sensitive prediction under covariate shift—a relaxation of this statistical assumption. This approach provides robustness to data shifts between predictive model estimation and deployment while incorporating mistake-specific costs for different errors that can be tied to application outcomes. We propose theoretical analysis and experimental investigation of this approach for standard and active learning tasks.
Project Summary
The devastation of the 2008 financial crisis remains a fresh memory seven years later, and its effects still reverberate in the global economy. The loss of trillions of dollars in output, and associated tragedy of displacement for millions of people demonstrate in the most vivid way the crucial role of a functional financial system for modern civilization. Unlike physical disasters, financial crises are essentially information events: shocks in the beliefs and expectations of individuals and organizations–about asset values, ability of counterparties to meet obligations, etc.–that nevertheless have real consequences for everyone.
This pivotal and fragile sector also happens to be at the leading edge of autonomous computational (AI) decision making. For large classes of financial assets, trading is dominated by algorithms, or “bots”, operating at speeds well beyond the scale of human reaction times. This regime change is a fait accompli, despite our unresolved debates and generally poor understanding of its implications for fundamental market stability as well as performance and efficiency.
We propose a systematic in-depth study of AI risks to the financial system. Our goals are to identify the main pathways of concern and generate constructive solutions for making financial infrastructure more robust to interaction with AI participants.
Technical Abstract
The financial system presents a critical sector of our society, at the leading-edge of AI engagement and especially vulnerable to impact from near-term AI advances. Algorithmic and high-frequency trading now dominate financial markets, yet their implications for market stability are poorly understood. In this project we undertake a systematic investigation of how AI traders can impact market stability, and how extreme movements in securities markets in turn can impact the real economy. We develop a general framework for automated trading based on a flexible architecture for arbitrage reasoning. Through agent-based simulation combined with game-theoretic strategy selection, we search for vulnerabilities in financial markets, and characterize the conditions that enable or prevent their exploitation. A new approach to modeling complex networks of financial obligations is applied to the study of contagion between asset-pricing anomalies and panics in the broader financial system. Results from this study will be employed to design market rules, monitoring technologies, and regulation techniques that promote stability in a world of algorithmic traders.
Project Summary
Deep learning architectures have fundamentally changed the capabilities of machine learning and benefited many applications such as computer vision, speech recognition, natural language processing, with many more influences to other problems coming along. However, very little is understood about those networks. Months of manual tuning is required for obtaining excellent performance, and the trained networks are often not robust: recent studies have shown that the error rate increases significantly with just slight pixel-level perturbations in image that are not even perceivable by human eyes.
In this proposal, The PI propose to thoroughly study the optimization and robustness of deep convolutional networks in visual object recognition, in order to gain more understanding about deep learning. This includes training procedures that will make deep learning more automatic and lead to less failures in training, as well as confidence estimates when the deep network is utilized to predict on new data. The confidence estimates can be used to control the behavior of a robot employing deep learning so that it will not go on to perform maneuvers that could be dangerous because of erroneous predictions. Understanding these aspects would also be helpful in designing potentially more robust networks in the future.
Technical Abstract
This work will focus on predicting whether a deep convolutional neural network (CNN) has succeeded. This includes two aspects, first, to find an explanation of why and when can the stochastic optimization in a deep CNN succeed without overfitting and obtain high accuracy. Second, to establish an estimate of confidence of the predictions of the deep learning architecture. Those estimates of confidence can be used as safeguards when utilizing those networks in real life. In order to establish those estimates, this work proposes to start from intuitions drawn from empirical analyses from the training procedure and model structures of deep learning. In-depth analyses will be completed for the mini-batch training procedure and model structures, by illustrating the differences each mini-batch size provides for the training, as well as the low-dimensional manifold structure in the classification. From those analyses, this work will result in approaches to design and control a proper training procedure with less human intervention, as well as confidence estimates by estimating the distance of the testing data to the submanifold that the trained network is effective on.
Project Summary
Developing AI systems that are benevolent towards humanity requires making sure that those systems know what humans want. People routinely make inferences about the preferences of others and use those inferences as the basis for helping one another. This project aims to provide AI systems a similar ability to learn from observations, in order to better align the values of those systems with those of humans. Doing so requires dealing with some significant challenges: If we ultimately develop AI systems that can reason better than humans, how do we make sure that those AI systems are able to take human limitations into account? The fact that we haven’t yet cured cancer shouldn’t be taken as evidence that we don’t really care about it. Furthermore, once we have made an AI system that can reason about human preferences, that system then has to trade off time spent in deliberating about the right course of action with the need to act as quickly as possible – it needs to deal with its own computational limitations as it makes decisions. We aim to address both these challenges by examining how intelligent agents (be they humans or computers) should make these tradeoffs.
Technical Abstract
AI research has focused on improving the decision-making capabilities of computers, i.e., the ability to select high-quality actions in pursuit of a given objective. When the objective is aligned with the values of the human race, this can lead to tremendous benefits. When the objective is misaligned, improving the AI system’s decision-making may lead to worse outcomes for the human race. The objectives of the proposed research are (1) to create a mathematical framework in which fundamental questions of value alignment can be investigated; (2) to develop and experiment with methods for aligning the values of a machine (whether explicitly or implicitly represented) with those of humans; (3) to understand the relationships among the degree of value alignment, the decision-making capability of the machine, and the potential loss to the human; and (4) to understand in particular the implications of the computational limitations of humans and machines for value alignment. The core of our technical approach will be a cooperative, game-theoretic extension of inverse reinforcement learning, allowing for the different action spaces of humans and machines and the varying motivations of humans; the concepts of rational metareasoning and bounded optimality will inform our investigation of the effects of computational limitations.
Project Summary
Artificial Intelligence (AI) is a broad and open-ended research area, and the risks that AI systems will pose in the future are extremely hard to characterize. However, it seems likely that any AI system will involve substantial software complexity, will depend on advanced mathematics in both its implementation and justification, and will be naturally flexible and seem to degrade gracefully in the presence of many types of implementation errors. Thus we face a fundamental challenge in developing trustworthy AI: how can we build and maintain complex software systems that require advanced mathematics in order to implement and understand, and which are all but impossible to verify empirically? We believe that it will be possible and desirable to formally state and prove that the desired mathematical properties hold with respect to the underlying programs, and to maintain such proofs as part of the software artifacts themselves. We propose to demonstrate the feasibility of this methodology by building a system that takes beliefs about the world in the form of probabilistic models, synthesizes inference algorithms to update those beliefs in the presence of observations, and provides formal proofs that the inference algorithms are correct with respect to the laws of probability.
Technical Abstract
It seems likely that any AI system will involve substantial software complexity, will depend on advanced mathematics in both its implementation and justification, and will be naturally flexible and seem to degrade gracefully in the presence of many types of implementation errors. Thus we face a fundamental challenge in developing trustworthy AI: how can we build and maintain complex software systems that require advanced mathematics in order to implement and understand, and which are all but impossible to verify empirically? We believe that it will be possible and desirable to formally state and prove that the desired mathematical properties hold with respect to the underlying programs, and to maintain and evolve such proofs as part of the software artifacts themselves. We propose to demonstrate the feasibility of this methodology by implementing several different certified inference algorithms for probabilistic graphical models, including the Junction Tree algorithm, Gibbs sampling, Mean Field, and Loopy Belief Propagation. Each such algorithm has a very different notion of correctness that involves a different area of mathematics. We will develop a library of the relevant formal mathematics, and then for each inference algorithm, we will formally state its specification and prove that our implementation satisfies it.
Results
Contents
Selection of Publications
- Achim, T, et al. Beyond parity constraints: Fourier analysis of hash functions for inference. Proceedings of The 33rd International Conference on Machine Learning, pages 2254–2262, 2016.
- Armstrong, Stuart and Orseau, Laurent. Safely Interruptible Agents. Uncertainty in Artificial Intelligence (UAI) 2016. https://www.fhi.ox.ac.uk/interruptibility/.
- This paper was cited by various news outlets including Business Insider and Forbes.
- Asaro, P. The Liability Problem for Autonomous Artificial Agents, Proceedings of the AAAI Symposium on Ethical and Moral Considerations in Non-Human Agents, Stanford University, Stanford, CA, March 21-23, 2016. https://www.aaai.org/ocs/index.php/SSS/SSS16/paper/view/12699
- Bai, Aijun and Russell, Stuart. Markovian State and Action Abstractions in Monte Carlo Tree Search. In Proc. IJCAI16, New York, 2016. https://people.eecs.berkeley.edu/~russell/papers/ijcai16-markov.pdf
- Boddington, Paula. EPSRC Principles of Robotics: Commentary on safety, robots as products, and responsibility. Ethical Principles of Robotics, special issue, 2016.
- Boddington, Paula. The Distinctiveness of AI Ethics, and Implications for Ethical Codes. Presented at IJCAI-16 Workshop 6 Ethics for Artificial Intelligence, New York, July 2016.
- Bostrom, N. Strategic Implications of Openness in AI Development, Technical Report #20161, Future of Humanity Institute, Oxford University: pp. 126, 2016. https://www.fhi.ox.ac.uk/wp-content/uploads/openness.pdf
- Chen, Xiangli, et al. Robust Covariate Shift Regression. International Conference on Artificial Intelligence and Statistics (AISTATS), 2016. http://jmlr.org/proceedings/papers/v51/chen16d.pdf
- Conitzer, Vincent, et al. Moral Decision Making Frameworks for Artificial Intelligence. (Preliminary version.) To appear in Proceedings of the Thirty-First AAAI Conference on Artificial Intelligence (AAAI-17) Senior Member / Blue Sky Track, San Francisco, CA, USA, 2017. https://users.cs.duke.edu/~conitzer/moralAAAI17.pdf
- Critch, Andrew. Parametric Bounded Löb’s Theorem and Robust Cooperation of Bounded Agents. 2016. http://intelligence.org/files/ParametricBoundedLobsTheorem.pdf
- Evans, Owain, et al. Learning the Preferences of Bounded Agents. NIPS Workshop on Bounded Optimality, 2015. http://stuhlmueller.org/papers/preferencesnipsworkshop2015.pdf
- Evans, Owain, et al. Learning the Preferences of Ignorant, Inconsistent Agents. 2015. http://arxiv.org/pdf/1512.05832.pdf
- Fathony, Rizal, et al. Multiclass Classification: A Risk Minimization Perspective. Neural Information Processing Systems (NIPS), 2016. https://papers.nips.cc/paper/6088-adversarial-multiclass-classification-a-risk-minimization-perspective.pdf
- Fulton, Nathan and Platzer, André. A logic of proofs for differential dynamic logic: Toward independently checkable proof certificates for dynamic logics. Jeremy Avigad and Adam Chlipala, editors, Proceedings of the 2016 Conference on Certified Programs and Proofs, CPP 2016, St. Petersburg, FL, USA, January 18-19, 2016, pp. 110-121. ACM, 2016. http://nfulton.org/papers/lpdl.pdf
- Garrabrant, Scott, et al. Asymptotically Coherent, Well Calibrated, Self-trusting Logical Induction. Working Paper (Berkeley, CA: Machine Intelligence Research Institute). 2016. https://arxiv.org/pdf/1609.03543.pdf
- Garrabrant, Scott, et al. Inductive Coherence. arXiv:1604.05288 . 2016. https://arxiv.org/pdf/1604.05288v3.pdf
- Garrabrant, Scott, et al. Asymptotic Convergence in Online Learning with Unbounded Delays. arXiv:1604.05280 . 2016. https://arxiv.org/pdf/1604.05280.pdf
- Greene, J. D. Our driverless dilemma. Science, 352(6293), 1514-1515. 2016.
- This paper was cited by several news outlets including the New York Times, the Washington Post, the Los Angeles Times, and the Daily Mail.
- Greene, J. et al. Embedding Ethical Principles in Collective Decision Support Systems. Thirtieth AAAI Conference on Artificial Intelligence. March 2016. www.aaai.org/ocs/index.php/AAAI/AAAI16/paper/download/12457/12204
- Hadfield-Menell, Dylan, et al. Cooperative Inverse Reinforcement Learning. Neural Information Processing Systems (NIPS), 2016. https://papers.nips.cc/paper/6420-cooperative-inverse-reinforcement-learning.pdf
- Hsu, L.K., et al. Tight variational bounds via random projections and i-projections. Proceedings of the 19th International Conference on Artificial Intelligence and Statistics, pages 1087–1095, 2016. https://arxiv.org/pdf/1510.01308v1.pdf
- Khani, F., et al. Unanimous prediction for 100% precision with application to learning semantic mappings. Association for Computational Linguistics (ACL), 2016. http://arxiv.org/pdf/1606.06368v2.pdf
- Kim, C., et al. Exact sampling with integer linear programs and random perturbations. Proc. 30th AAAI Conference on Artificial Intelligence, 2016. http://www.aaai.org/ocs/index.php/AAAI/AAAI16/paper/view/12105/12087
- Leike, Jan, et al. A Formal Solution to the Grain of Truth Problem. Uncertainty in Artificial Intelligence: 32nd Conference (UAI 2016), edited by Alexander Ihler and Dominik Janzing, 427–436. Jersey City, New Jersey, USA. 2016. http://www.auai.org/uai2016/proceedings/papers/87.pdf
- Liu, C., et al. Goal inference improves objective and perceived performance in human robot collaboration. In Proc. AAMAS16, Singapore, 2016. http://www.jesshamrick.com/publications/pdf/Liu2016-Goal_Inference_Improves_Objective.pdf
- Nivel, E., et al. Bounded Recursive Self-Improvement. Technical Report RUTR-SCS13006, Reykjavik University, 2013. https://arxiv.org/ftp/arxiv/papers/1312/1312.6764.pdf
- Perera, Vittorio, et al. Dynamic Generation and Refinement of Robot Verbalization. Proceedings of RO-MAN’16, the IEEE International Symposium on Robot and Human Interactive Communication, Columbia University, NY, August, 2016. http://www.cs.cmu.edu/ mmv/papers/16roman-verbalization.pdf
- Pistono, F and Yampolskiy, RV. Unethical research: How to create a malevolent artificial intelligence. 25th International Joint Conference on Artificial Intelligence (IJCAI-16), Ethics for Artificial Intelligence Workshop (AI-Ethics-2016). https://arxiv.org/ftp/arxiv/papers/1605/1605.02817.pdf
- Rosenthal, Stephanie, et al. Verbalization: Narration of Autonomous Mobile Robot Experience, In Proceedings of IJCAI’16, the 26th International Joint Conference on Artificial Intelligence, New York City, NY, July, 2016. http://www.cs.cmu.edu/ mmv/papers/16ijcai-verbalization.pdf
- Rossi, F. Ethical Preference-Based Decision Support System. Proc. CONCUR 2016, Springer. 2016. http://drops.dagstuhl.de/opus/volltexte/2016/6187/pdf/LIPIcs-CONCUR-2016-2.pdf
- Rossi, F. Moral preferences, Proc. IJCAI 2016 workshop on AI and ethics, and Proc. IJCAI 2016 workshop on multidisciplinary approaches to preferences. 2016. https://intelligence.org/files/csrbai/pref-eth1.pdf
- Siddiqui, A., et al. Finite Sample Complexity of Rare Pattern Anomaly Detection. Proceedings of UAI-2016 (pp. 10). 2016.
- Steinhardt, J. and Liang, P. Unsupervised Risk Estimation with only Conditional Independence Structure. Neural Information Processing Systems (NIPS), 2016. https://papers.nips.cc/paper/6201-unsupervised-risk-estimation-using-only-conditional-independence-structure
- Steunebrink, B.R., et al. Growing Recursive Self-Improvers. Proceedings of the 9th Conference on Artificial General Intelligence (AGI 2016), LNAI 9782, pages 129-139. Springer, Heidelberg. 2016. http://people.idsia.ch/~steunebrink/Publications/AGI16_growing_recursive_self-improvers.pdf
- Taylor, Erin. The Threat-Response Model: Ethical Decision in the Real World.
- Taylor, Jessica. Quantilizers: A Safer Alternative to Maximizers for Limited Optimization. 2nd International Workshop on AI, Ethics and Society at AAAI-2016. Phoenix, AZ. 2016. www.aaai.org/ocs/index.php/WS/AAAIW16/paper/download/12613/12354
- Thorisson, K.R., et al. Why Artificial Intelligence Needs a Task Theory (And What It Might Look Like). Proceedings of the 9th Conference on Artificial General Intelligence (AGI 2016), LNAI 9782, pages 118-128. Springer, Heidelberg. 2016. http://people.idsia.ch/~steunebrink/Publications/AGI16_task_theory.pdf
- Thorisson, K.R., et al. About Understanding. Proceedings of the 9th Conference on Artificial General Intelligence (AGI 2016), LNAI 9782, pages 106-117. Springer, Heidelberg. 2016. http://link.springer.com/chapter/10.1007/978-3-319-41649-6_11
- Tossou, A.C.Y. and Dimitrakakis, C. Algorithms for Differentially Private Multi-Armed Bandits. Proc. 13th AAAI Conf. on Artificial Intelligence (AAAI 2016), 2016. www.aaai.org/ocs/index.php/AAAI/AAAI16/paper/download/11906/11846
- Wellman, MP and Rajan, U. Ethical issues for autonomous trading agents. IJCAI-16 Workshop on Ethics for Artificial Intelligence, July 2016.
- Yampolskiy, RV. Taxonomy of pathways to dangerous AI. 30th AAAI Conference on Artificial Intelligence (AAAI-2016), 2nd International Workshop on AI, Ethics and Society (AI Ethics Society 2016). www.aaai.org/ocs/index.php/WS/AAAIW16/paper/download/12566/12356
- Zhang, et al. On the Differential Privacy of Bayesian Inference. Proc. 13th AAAI Conf. on Artificial Intelligence (AAAI 2016), 2016. http://www.aaai.org/ocs/index.php/AAAI/AAAI16/paper/viewFile/12129/11885
- Zhao, et al. Closing the gap between short and long xors for model counting. Thirtieth AAAI Conference on Artificial Intelligence, 2016. https://ai2-website.s3.amazonaws.com/publications/shortXors-AAAI2016.pdf
Articles
➣ Conitzer, Vincent. Artificial intelligence: where’s the philosophical scrutiny? Published in the magazine Prospect.
➣ Creighton, Jolene. The Evolution of AI: Can Morality be Programmed? Futurism, based on an interview about our project with Conitzer.
➣ Ermon, Stefano. What Are Some Recent Advances in Non-Convex Optimization Research? The Huffington Post. http://www.huffingtonpost.com/entry/what-are-some-recent-adva_b_956388
➣ Russell, Stuart. Moral Philosophy Will Become Part of the Tech Industry. Time, September 15, 2015. http://time.com/4026723/stuart-russell-will-ai-overtake-humans/
➣ Russell, Stuart. Should we fear super smart robots? Scientific American, 314, 58-59, June 2016. https://people.eecs.berkeley.edu/~russell/papers/sciam16-supersmart.pdf
➣ Taylor, Jessica. A first look at the hard problem of corrigibility. Intelligent Agent Foundations Forum, 2015. https://agentfoundations.org/item?id=484
➣Taylor, Jessica. A sketch of a value-learning sovereign. Intelligent Agent Foundations Forum, 2015. https://agentfoundations.org/item?id=533
➣Taylor, Jessica. Three preference frameworks for goal-directed agents. Intelligent Agent Foundations Forum, 2015. https://agentfoundations.org/item?id=539
➣Taylor, Jessica. What do we need value learning for? Intelligent Agent Foundations Forum, 2015. https://agentfoundations.org/item?id=538
➣ Weld, D.S. “The real threat of artificial intelligence,”Geekwire, May 23, 2016. http://www.geekwire.com/2016/guest-commentary-real-threat-artificial-intelligence/
Software Releases
➣ Andre Platzer: Major contributions to the KeYmaera X Theorem Prover for Hybrid Systems. Source code is available at https://github.com/LS-Lab/KeYmaeraX-release/releases
Course Materials
➣Kristen Brent Venable, IHMC: Taught a new ad-hoc independent study course entitled “Ethics for Artificial Intelligence” during the spring 2016 semester with the goal of carrying out an in-depth state of the review of models for ethical issues and ethical values in AI.
➣ Owain Evans (AgentModels.org): An interactive online textbook, to communicate the idea of IRL to a broader audience and to give a detailed explanation of our approach to IRL to the existing AI Safety and AI/ML communities.
➣ Joshua Greene, Harvard: Spring 2016, graduate seminar “Evolving Morality: From Primordial Soup to Superintelligent Machines.”
➣ Andre Platzer: Foundations of Cyber-Physical Systems (Spring 2016)
- Syllabus, Assignments, Lecture Videos: http://symbolaris.com/course/fcps16-schedule.html
- Final Projects: http://symbolaris.com/course/fcps16-projects.html
➣ Stuart Russell, Tom Griffiths, Anca Dragan, UC Berkeley: Spring 2016, graduate course on “Human-Compatible AI”
Workshops Funded
➣ The Control Problem in AI: by the Strategic AI Research Centre
This was an intensive workshop at Oxford, with a large number of participants, and covered, among many other things, goals and principles of AI policy and strategy, value alignment for advanced machine learning, the relative importance of AI v. other x-risk, geopolitical strategy, government involvement, analysis of the strategic landscape, theory and methods of communication and engagement, the prospects of international-space-station-like coordinated AGI development, and an enormous array of technical AI control topics.
➣ Policies for Responsible AI Development: by the Strategic AI Research Centre
This workshop focused on a selection of key areas, such as: classifying risks, international governance, and surveillance. The workshop also engaged in a series of brainstorming and analysis exercises. The brainstorming sessions included “rapid problem attacks” on especially difficult issues, a session drafting various “positive visions” for different AI development scenarios, and a session (done in partnership with Open Philanthropy) which involved brainstorming ideas for major funders interested in x-risk reduction. This workshop even engaged in two separate “red team” exercises in which we sought out vulnerabilities, first in our own approach and research agenda, and then on global security.
➣ Intersections between Moral Psychology and Artificial Intelligence: by Molly Crockett and Walter Sinnott-Armstrong.
This workshop included two panels. The first asked whether artificial intelligence systems could ever provide reliable moral advice on a wide range of issues. Two speakers were skeptical about traditional top-down approaches, but the other two speakers argued that new alternatives are more promising. The second panel focussed on particular applications of artificial intelligence in war. The panelists again vigorously disagreed but left with a much better understanding of each other’s positions. Both panels were very diverse in their disciplinary backgrounds. The audience consisted of approximately 50 professors and students as well as members of the public.
➣ Moral AI Projects: by Vincent Conitzer, Walter Sinnott-Armstrong, Erin Taylor, and others.
Each part of this workshop included an in depth discussion of an innovative model for moral artificial intelligence. The first group of speakers explained and defended the bottom-up approach that they are developing with support from the FLI. The second session was led by a guest speaker who presented a dialogic theory of moral reasoning that has potential to be programmed into artificial intelligence systems. In the end, both groups found that their perspectives were complementary rather than competing. The audience consisted of around 20 students and faculty from a wide variety of fields.
➣ Embedded Machine Learning: by Dragos Margineantu (Boeing), Rich Caruana (Microsoft Research), Thomas Dietterich (Oregon State University).
This workshop took place at the AAAI Fall Symposium, Arlington, VA, November 12-14, 2015 and included issues of Unknown Unknowns in machine learning and more generally touched on issues at the intersection of software engineering and machine learning, including verification and validation.
➣ The Future of Artificial Intelligence: by Jacob Steinhardt, Stanford; Tom Dietterich, OSU; Percy Liang, Stanford; Andrew Critch, MIRI; Jessica Taylor, MIRI; Adrian Weller, Cambridge.
The Future of Artificial Intelligence workshop was held at NYU. The first day consisted of two public sessions on the subject of “How AI is Used in Industry, Present and Future”. The first session included talks by Eric Schmidt (Alphabet), Mike Schroepfer (Facebook), Eric Horvitz (MSR), and me. This was followed by a panel including all of us plus Demis Hassabis (Deep Mind) and Bart Selman (Cornell). I talked about AI applications in science (bird migration, automated scientist), law enforcement (fraud detection, insider threat detection), and sustainability (managing invasive species). This session was generally very up-beat about the potential of AI to do great things. The second session had talks by Jen-Hsun Huang (NVIDIA), Amnon Shashua (Mobileye), John Kelly (IBM), and Martial Hebert (CMU). The final session turned toward the present and future of AI with presentations by Bernhard Schölkopf (Max Planck Institute), Demis Hassabis (Google DeepMind), and Yann LeCun (Facebook AI Research & NYU). Bernhard spoke about discovering causal relationships, Demis spoke about artificial general intelligence and his vision of how to achieve it. Yann discussed “differentiable programs” and raised the issue of whether we can differentiate traditional symbolic AI methods or need to adopt continuous representations for them.
The second and third days of the workshop were subject to Chatham House Rules. Many topics were discussed including (a) the impact of AI on the future of employment and economic growth, (b) social intelligence and human-robot interaction, (c) the time scales of AI risks: short term, medium term, and very long term, (d) the extent to which mapping the brain will help us understand how the brain works, (e) the future of US Federal funding for AI research and especially for young faculty, (f) the challenges of creating AI systems that understand and exhibit ethical behavior, (g) the extent to which AI should be regulated either by government or by community institutions and standards, and (h) how do we develop appropriate “motivational systems” for AI agents?
➣ Reliable Machine Learning in the Wild: by Jacob Steinhardt, Stanford; Tom Dietterich, OSU; Percy Liang, Stanford; Andrew Critch, MIRI; Jessica Taylor, MIRI; Adrian Weller, Cambridge.
This was an ICML Workshop, NY, June 23, 2016. This workshop discussed a wide range of issues related to engineering reliable AI systems. Among the questions discussed were (a) how to estimate causal effects under various kinds of situations (A/B tests, domain adaptation, observational medical data), (b) how to train classifiers to be robust in the face of adversarial attacks (on both training and test data), (c) how to train reinforcement learning systems with risk-sensitive objectives, especially when the model class may be misspecified and the observations are incomplete, and (d) how to guarantee that a learned policy for an MDP satisfies specified temporal logic properties. Several important engineering practices were also discussed, especially engaging a Red Team to perturb/poison data and making sure we are measuring the right data. My assessment is that a research community is coalescing nicely around these questions, and the quality of the work is excellent.
More details of the workshop can be found at our website: https://sites.google.com/site/wildml2016/.
➣ MIRI hosted one stand-alone workshop, and also co-hosted a 22-day June colloquium series with the Future of Humanity Institute, which included four additional workshops.
Over 50 people attended the colloquium series from 25 different institutions, including Stuart Russell (UC Berkeley), Bart Selman (Cornell), Francesca Rossi (IBM Research), and Tom Dietterich (Oregon State). MIRI also ran four research retreats, internal workshops exclusive to MIRI researchers
- Workshop #1: Self-Reference, Type Theory, and Formal Verification. April 1-3.
Participants worked on questions of self-reference in type theory and automated theorem provers, with the goal of studying systems that model themselves.
Participants: Benya Fallenstein (MIRI), Daniel Selsam (Stanford), Jack Gallagher (Gallabytes), Jason Gross (MIT), Miëtek Bak (Least Fixed), Nathaniel Thomas (Stanford), Patrick LaVictoire (MIRI), Ramana Kumar (Cambridge)
- Workshop #2: Transparency. May 28-29.
In many cases, it can be prohibitively difficult for humans to understand AI systems’ internal states and reasoning. This makes it more difficult to anticipate such systems’ behavior and correct errors. On the other hand, there have been striking advances in communicating the internals of some machine learning systems, and in formally verifying certain features of algorithms. We would like to see how far we can push the transparency of AI systems while maintaining their capabilities.
Slides are up for Tom Dietterich's overview talk at this workshop, "Issues Concerning AI Transparency" (https://intelligence.org/files/csrbai/dietterich-slides.pdf).
Participants: Nate Soares (MIRI), Andrew Critch (MIRI), Patrick LaVictoire (MIRI), Jessica Taylor (MIRI), Scott Garrabrant (MIRI), Alan Fern (Oregon State University), Daniel Filan (Australian National University), Devi Borg (Future of Humanity Institute), Francesca Rossi (IBM Research), Jack Gallagher (Gallabytes), János Kramár (Montreal Institute for Learning Algorithms), Jim Babcock (unaffiliated), Marcello Herreshoff (Google), Moshe Looks (Google), Nathaniel Thomas (Stanford), Nisan Stiennon (Google), Sune Jakobsen (University College Longdon), Tom Dietterich (Oregon State University), Tsvi Benson-Tilsen (UC Berkeley), Victoria Krakovna (Future of Life Institute)
- Workshop #3: Robustness and Error-Tolerance. June 4-5.
How can we ensure that when AI system fail, they fail gracefully and detectably? This is difficult for systems that must adapt to new or changing environments; standard PAC guarantees for machine learning systems fail to hold when the distribution of test data does not match the distribution of training data. Moreover, systems capable of means-end reasoning may have incentives to conceal failures that would result in their being shut down. We would much prefer to have methods of developing and validating AI systems such that any mistakes can be quickly noticed and corrected.
Participants: Andrew Critch (MIRI), Patrick LaVictoire (MIRI), Jessica Taylor (MIRI), Scott Garrabrant (MIRI), Abram Demski (USC Institute for Creative Technologies), Bart Selman (Cornell), Bas Steunebrink (IDSIA), Daniel Filan (Australian National University), Devi Borg (Future of Humanity Institute), Jack Gallagher (Gallabytes), Jim Babcock, Nisan Stiennon (Google), Ryan Carey (Centre for the Study of Existential Risk), Sune Jakobsen (University College Longdon)
- Workshop #4: Preference Specification. June 11-12.
The perennial problem of wanting code to “do what I mean, not what I said” becomes increasingly challenging when systems may find unexpected ways to pursue a given goal. Highly capable AI systems thereby increase the difficulty of specifying safe and useful goals, or specifying safe and useful methods for learning human preferences.
Participants: Patrick LaVictoire (MIRI), Jessica Taylor (MIRI), Abram Demski (USC Institute for Creative Technologies), Bas Steunebrink (IDSIA), Daniel Filan (Australian National University), David Abel (Brown University), David Krueger (Montreal Institute for Learning Algorithms), Devi Borg (Future of Humanity Institute), Jan Leike (Future of Humanity Institute), Jim Babcock (unaffiliated), Lucas Hansen (unaffiliated), Owain Evans (Future of Humanity Institute), Rafael Cosman (unaffiliated), Ryan Carey (Centre for the Study of Existential Risk), Stuart Armstrong (Future of Humanity Institute), Sune Jakobsen (University College Longdon), Tom Everitt (Australian National University), Tsvi Benson-Tilsen (UC Berkeley), Vadim Kosoy (Epicycle)
- Workshop #5: Agent Models and Multi-Agent Dilemmas. June 17.
When designing an agent to behave well in its environment, it is risky to ignore the effects of the agent’s own actions on the environment or on other agents within the environment. For example, a spam classifier in wide use may cause changes in the distribution of data it receives, as adversarial spammers attempt to bypass the classifier. Considerations from game theory, decision theory, and economics become increasingly useful in such cases.
Participants: Andrew Critch (MIRI), Patrick LaVictoire (MIRI), Abram Demski (USC Institute for Creative Technologies), Andrew MacFie (Carleton University), Daniel Filan (Australian National University), Devi Borg (Future of Humanity Institute), Jaan Altosaar (Google Brain), Jan Leike (Future of Humanity Institute), Jim Babcock (unaffiliated), Matthew Johnson (Harvard), Rafael Cosman (unaffiliated), Stefano Albrecht (UT Austin), Stuart Armstrong (Future of Humanity Institute), Sune Jakobsen (University College Longdon), Tom Everitt (Australian National University), Tsvi Benson-Tilsen (UC Berkeley), Vadim Kosoy (Epicycle)
- Workshop #6: Logic, Probability, and Reflection. August 12-14.
Participants at this workshop, consisting of MIRI staff and regular collaborators, worked on a variety of problems related to MIRI’s Agent Foundations technical agenda, with a focus on decision theory and the formal construction of logical counterfactuals.
Participants: Andrew Critch (MIRI), Benya Fallenstein (MIRI), Eliezer Yudkowsky (MIRI), Jessica Taylor (MIRI), Nate Soares (MIRI), Patrick LaVictoire (MIRI), Sam Eisenstat (UC Berkeley), Scott Garrabrant (MIRI), Tsvi Benson-Tilsen (UC Berkeley)
➣ Control and Responsible Innovation in the Development of Autonomous Systems Workshop: by The Hastings Center
The four co-chairs (Gary Marchant, Stuart Russell, Bart Selman, and Wendell Wallach) and The Hastings Center staff (particularly Mildred Solomon and Greg Kaebnick) designed this first workshop. This workshop was focused on exposing participants to relevant research progressing in an array of fields, stimulating extended reflection upon key issues and beginning a process of dismantling intellectual silos and loosely knitting the represented disciplines into a transdisciplinary community. Twenty-five participants gathered at The Hastings Center in Garrison, NY from April 24th - 26th, 2016. The workshop included representatives from key institutions that have entered this space, including IEEE, the Office of Naval Research, the World Economic Forum, and of course AAAI. They are planning a second workshop, scheduled for October 30-November 1, 2016. The invitees for the second workshop are primarily scientists, but also include social theorists, legal scholars, philosophers, and ethicists. The expertise of the social scientists will be drawn upon in clarifying the application of research in cognitive science and legal and ethical theory to the development of autonomous systems. Not all of the invitees to the second workshop have considered the challenge of developing beneficial trustworthy artificial agents. However, we believe we are bringing together brilliant and creative minds to collectively address this challenge. We hope that scientific and intellectual leaders, new to the challenge and participating in the second workshop, will take on the development of beneficial, robust, safe, and controllable AI as a serious research agenda.
➣ A Day of Ethical AI at Oxford: by Michael Wooldridge, Peter Millican, and Paula Boddington
This workshop was held at the Oxford Martin School on June 8th, 2016. The goal of the workshop was collaborative discussion between those working in AI and ethics and related areas, between geographically close and linked centres. Participants were invited from the Oxford Martin School, The Future of Humanity Institute, the Cambridge Centre for the Study of Existential Risk, and the Leverhulme Centre for the Future of Intelligence, plus others. Participants included FLI grantholders. This workshop included participants from diverse disciplines, including computing,philosophy and psychology, to facilitate cross disciplinary conversation and understanding.
➣ Ethics for Artificial Intelligence: by Brian Ziebart
This workshop took place at IJCAI-’16, July 9th, 2016, in New York. This workshop focussed on selecting papers which speak to the themes of law and autonomous vehicles, ethics of autonomous systems, and superintelligence.
Workshop Participation and Presentation
➣ Asaro, P. (2016) “Ethics for Artificial Intelligence,” International Joint Conference on Artificial Intelligence (IJCAI), New York, NY, July 9, 2016. https://www.cs.ox.ac.uk/efai/call-for-papers/
➣ Asaro, P. (2016) “AI Now: The Social and Economic Implications of Artificial Intelligence,” Whitehouse Workshop on AI, New York University, New York, NY, July 7, 2016. https://artificialintelligencenow.com/
➣ Asaro, P. (2016). “Autonomous Weapons,” Computers Gone Wild Workshop, Berkman Center for Internet and Society, Harvard University, Cambridge, MA, February 19, 2016. https://cyber.law.harvard.edu/node/99484
➣ Asaro, P. (2015). “The Internet of (Smart) Things,” and “Ethics Panel,” Blockchain Workshop, Harvard Berkman Center, Sydney, Australia, December 10-11, 2015. http://sydney.blockchainworkshops.org/And collaborative product: http://networkedsystemsethics.net/index.php?title=Networked_Systems_Ethics
➣ Asaro, P. (2015). “Internet of Things” and “Philosophical Panel,” Blockchain Workshop, Harvard Berkman Center, Hong Kong, China, October 11-13, 2015. http://blockchainworkshops.org/
➣ Asaro, P. (2015). “The Human Brain in the Age of Robots: Social & Ethical Issues,” Webinar on Future Computing and Robotics in the Human Brain Project, Danish Board of Technology, October 9, 2015. http://www.tekno.dk/article/future-computing-and-robotics/?lang=en
➣ Asaro, P. (2016). “Regulating Autonomous Agents: The Scope and Limits of Liability,” 4thAnnual Conference on Governance of Emerging Technologies: Law, Policy & Ethics, Arizona State University, Tempe, AZ, May 24-26, 2016. http://conferences.asucollegeoflaw.com/get2016/
➣ Asaro, P. (2016). “The Liability Problem for Autonomous Artificial Agents,”AAAI Symposium on Ethical and Moral Considerations in Non-Human Agents, Stanford University, Stanford, CA, March 21-23, 2016. https://sites.google.com/site/ethicalnonhumanagents/
➣ Asaro, P.(2015). “Concepts of Agency & Autonomy: Towards the Governance of Autonomous Weapons,” Meeting of the Society for the Social Studies of Science, Denver, Co, November 11-15, 2015. http://convention2.allacademic.com/one/ssss/4s15/
➣ Walter Sinnott-Armstrong: co-organized and spoke at a workshop on “Moral Issues in Artificial Intelligence”at the Oxford Martin School of Oxford University.
➣ Seth Baum, Anthony Barrett, and Roman Yampolskiy presented their research at the 2015 Society for Risk Analysis Annual Meeting
➣ Seth Baum organized several informal meetings on AI safety with attendees from (among other places) CSER, FHI, MIRI, Yale, and the United Nations at the International Joint Conference on Artificial Intelligence
➣ Vincent Conitzer: participated in the ethics workshop at AAAI, describing our work on this project in a session and also serving on a panel on research directions for keeping AI beneficial.
➣ Owen Cotton-Barratt: presented on new ideas at a one-day workshop on “Ethical AI” in Oxford on June 8, 2016. He has further developed informal models of likely crucial parameters to include in the models, and he now believes that the model should additionally include a division between scenarios where a single AI-enabled actor gains a decisive strategic advantage, and ones where this does not occur.
➣ Dietterich, T. G. (2015). Toward Beneficial Artificial Intelligence. Blouin Creative Leadership Summit, NY, NY, September 21, 2015.
➣ Dietterich, T. G. (2015). Artificial Intelligence: Progress and Challenges. Technical and Business Perspectives on the Current and Future Impact of Machine Learning. Valencia, Spain, October 20, 2015. Press coverage in El Mundo.
➣ Dietterich, T. G. (2015). Algorithms Among Us: The Societal Impacts of Machine Learning(opening remarks). NIPS Symposium. Montreal, Canada, December 10, 2015.
➣ Dietterich, T. G. (2016). AI in Science, Law Enforcement, and Sustainability. The Future of Artificial Intelligence. NYU, January 11, 2016.I also participated in a side meeting with Henry Kissinger on January 13 along with Max Tegmark and several other key people.
➣ Dietterich, T. G. (2016). Steps Toward Robust Artificial Intelligence(AAAI President’s Address). AAAI Conference on Artificial Intelligence, Phoenix, AZ. February 14, 2016.
➣ Dietterich, T. G. (2016). Testing, Verification & Validation, Monitoring. Control and Responsible Innovation in the Development of Autonomous Machines. Hastings Center, Garrison, NY, April 25, 2016.
➣ Dietterich, T. G. (2016). Steps Toward Robust Artificial Intelligence(short version). Huawei STW Workshop, Shenzhen, China, May 17, 2016.
➣ Dietterich, T. G. (2016). Steps Toward Robust Artificial Intelligence. Distinguished Seminar, National Key Laboratory for Novel Software Technology, University of Nanjing, Nanjing, China, May 19, 2016.
➣ Dietterich, T. G. (2016). Understanding and Managing Ecosystems through Artificial Intelligence. AI For Social Good. White House OSTP Workshop. Washington, DC, June 6-7, 2016.
➣ Dietterich, T. G., Fern, A., Wong, W-K., Emmott, A., Das, S., Siddiqui, M. A., Zemicheal, T.(2016). Anomaly Detection: Principles, Benchmarking, Explanation, and Theory. ICML Workshop on Anomaly Detection Keynote Speech.NY. June, 24, 2016.
➣ Dietterich, T. G. (2016). Making artificial intelligence systems robust. Safe Artificial Intelligence. White House OSTP Workshop, Pittsburgh, PA, June 28, 2016.
➣ Fern, A., Dietterich, T. G. (2016). Toward Explainable Uncertainty. MIRI Colloquium Series on Robust and Beneficial Artificial Intelligence.Alan and I also participated inthe two-day workshop on Transparency.MIRI, Berkeley, CA. May 27-29, 2016.
➣ Nathan Fulton:
- Presented A Logic of Proofs for Differential Dynamic Logic: Toward Independently Checkable Proof Certificates for Dynamic Logics at The 5th ACM SIGPLAN Conference on Certified Programs and Proofs.
- Nathan Fulton, Stefan Mitsch, and André Platzer presented a tutorial on KeYmaera X and hybrid systems verification at CPSWeek 2016, and a similar tutorial has been accepted at FM 2016.
- Nathan Fulton presented a talk on work supported by this grant at a workshop on Safe AI for CPS held at Carnegie Mellon in April 2016. http://www.ls.cs.cmu.edu/CPSVVIF-2016/Program.html
➣ Percy Liang: Workshop on Human Interpretability in Machine Learning at ICML 2016. Presented two papers:
- J. Steinhardt and P. Liang. Unsupervised Risk Estimation with only Conditional Independence Structure. Neural Information Processing Systems (NIPS), 2016.
- F. Khani and M. Rinard and P. Liang. Unanimous prediction for 100% precision with application to learning semantic mappings. Association for Computational Linguistics (ACL), 2016. http://arxiv.org/pdf/1606.06368v2.pdf
➣ Francesca Rossi:
- German conference on AI (KI 2015) in September 2015, titled “Safety constraints and ethical principles in collective decision making systems”
- “Moral Preferences”-- ACS 2016 (Conference on Advances in Cognitive Systems, see http://www.cogsys.org/2016), June 2016 -- Colloquium Series on Robust and Beneficial AI (CSRBAI) of MIRI (see https://intelligence.org/colloquium-series/)
- “Ethical Preference-Based Decision Support Systems”-- CONCUR 2016 (Int’l conference on concurrency theory, see https://www.concur2016.ulaval.ca/no_cache/home/) , August 2016
- Ethics of AI -- Two TEDx talks: TEDx Lake Como in November 2015, TEDx Ghent in June 2015, TEDx Osnabruck in April 2015
➣ Stuart Russell
- "The long-term future of (artificial) intelligence", invited lecture, Software Alliance Annual Meeting, Napa, Nov 13, 2015
- "The Future of AI and the Human Race", TedX talk, Berkeley, Nov 8, 2015
- "Value Alignment", invited lecture, Workshop on Algorithms for Human-Robot Interaction, Nov 18, 2015
- "Killer Robots, the End of Humanity, and All That", Award Lecture, World Technology Awards, New York, Nov 2015
- "Should we Fear or Welcome the Singularity?", panel presentation, Nobel Week Dialogue, December 2015
- "The Future of Human-Computer Interaction", panel presentation (chair), Nobel Week Dialogue, December 2015
- "The Future Development of AI", panel presentation, Nobel Week Dialogue, December 2015
- "Some thoughts on the future", invited lecture, NYU AI Symposium, January 2016
- "The State of AI", televised panel presentation, World Economic Forum, Davos, January 2016
- "AI: Friend or Foe?" panel presentation, World Economic Forum, Davos, January 2016
- "The long-term future of (artificial) intelligence", CERN Colloquium, Geneva, Jan 16,2016
- "Some thoughts on the future", invited presentation, National Intelligence Council,Berkeley, Jan 28, 2016
- "The long-term future of (artificial) intelligence", Herbst Lecture, University of Colorado, Boulder, March 11 2016
- "The Future of AI", Keynote Lecture, Annual Ethics Forum, California State University Monterey Bay, March 16, 2016
- "The long-term future of (artificial) intelligence", IARPA Colloquium, Washington DC,March 21 2016
- "AI: Friend or Foe?", panel presentation, Milken Global Institute, Los Angeles, May 2,2016
- "Will Superintelligent Robots Make Us Better People?", Keynote Lecture (televised),Seoul Digital Forum, South Korea, May 19, 2016
- "The long-term future of (artificial) intelligence", Keynote Lecture, Strata Big Data Conference, London, June 2, 2016
- "Moral Economy of Technology", panel presentation, Annual Meeting of the Society for the Advancement of Socio-Economics, Berkeley, June 2016
➣ Michael Wooldridge and Paula Boddington:
- EPSRC Systems-Net Grand Challenge Workshop, “Ethics in Autonomous Systems”, Sheffield University, November 25, 2015.
- AISB workshop on Principles of Robotics, Sheffield University, 4 Apr 2016
- Workshop examined the EPSRC (Engineering and Physical Sciences Research Council) Principles of Robotics. Boddington presented a paper, “Commentary on responsibility, product design and notions of safety”, and contributed to discussion.
- Outcome of workshop: Paper for Special Issue of Connection Science on Ethical Principles of Robotics, ‘EPSRC principles of robotics: Commentary on Safety, Robots as Products, and Responsibility”--Paula Boddington
➣ Bas Steunebrink:
- AAAI-16 conference in Phoenix.
- Colloquium Series on Robust and Beneficial AI (CSRBAI), hosted by the Machine Intelligence Research Institute in Berkeley, in collaboration with the Future of Humanity Institute at Oxford.
- AGI-16 conference in New York.
- IEEE Symposium on Ethics of Autonomous Systems (SEAS Europe).
- ECAI-16 conference in The Hague.
➣ Manuela Veloso
- OSTP/NYU Workshop on The Social and Economic Implications of Artificial Intelligence Technologies in the Near-Term, NYC, July 2016.
- Intelligent Autonomous Vehicles Conference, Leipzig, July 2016.
- STP/CMU Workshop on Safety and Control for Artificial Intelligence, Pittsburgh, June 2016. (video at https://scs.hosted.panopto.com/Panopto/Pages/Viewer.aspx?id=8c9e1eb9-ddb4-4990-8198-485a72044b51).
- Founders Forum, London, June 2016.
- MIT Technology Review EmTech Digital, San Francisco, May 2016.
➣ Understanding and Mitigating AI Threats to the Financial System (MP Wellman and Uday Rajan). Center for Finance, Law, and Policy, University of Michigan, 4 Jun 2015.
➣ Do Trading Algorithms Threaten Financial Market Stability? (MP Wellman). Conference on Interdisciplinary Approaches to Financial Stability, University of Michigan Law School, 22 Oct 2015.
➣ Autonomous Agents: Threat or Menace? (MP Wellman). Collegiate Professorship Lecture, University of Michigan, 5 May 2016. (Link: https://www.youtube.com/watch?v=x3d5ZTJC8K0)
➣ Autonomous Agents in Financial Markets: Implications and Risks (MP Wellman). Machine Intelligence Research Institute Colloquium on Robust and Beneficial AI, Berkeley, CA, 15 Jun 2016.
I. The Future of AI: Reaping the Benefits While Avoiding Pitfalls
For many years, Artificial Intelligence (AI) research has been appropriately focused on the challenge of making AI effective, with significant success. In an open letter in January 2015, a large international group of leading AI researchers from academia and industry argued that this success makes it important and timely to research also how to make AI systems robust and beneficial, and that this includes concrete research directions that can be pursued today. The aim of this request for proposals is to support such research.
The potential benefits are huge; everything that civilization has to offer is a product of human intelligence; we cannot predict what we might achieve when this intelligence is magnified by the tools AI may provide, but the eradication of war, disease, and poverty would be high on anyone’s list. However, like any powerful technology, AI has also raised new concerns, such as humans being replaced on the job market and perhaps altogether. Success in creating general-purpose human- or superhuman-level AI would be the biggest event in human history.
Unfortunately, it might also be the last, unless we learn how to avoid the risks. A crucial question is therefore what can be done now to maximize the future benefits of AI while avoiding pitfalls.
The attached research priorities document gives many examples of research directions that can help maximize the societal benefit of AI. This research is by necessity interdisciplinary, because it involves both society and AI. It ranges from economics, law and philosophy to computer security, formal methods and, of course, various branches of AI itself. The focus is on delivering AI that is beneficial to society and robust in the sense that the benefits are guaranteed: our AI systems must do what we want them to do. This is a significant expansion in the definition of the field, which up to now has focused on techniques that are neutral with respect to purpose.
II. Evaluation Criteria & Project Eligibility
This 2015 grants competition is the first wave of the $10M program announced this month, and will give grants totaling about $6M to researchers in academic and other non-profit institutions for projects up to three years in duration, beginning September 1 2015. Future competitions are anticipated to focus on the areas that prove most successful. Grant applications will be subject to a competitive process of confidential expert peer review similar to that employed by all major U.S. scientific funding agencies, with reviewers being recognized experts in the relevant fields.
Grants will be made in two categories: Project Grants and Center Grants. Project Grants (approx. $100K-$500K) will fund a small group of collaborators at one or more research institutions for a focused research project of up to three years duration. Center Grants (approx. $500K-$2M) will fund the establishment of a (possibly multi-institution) research center that organizes, directs and funds (via subawards) research.
Proposals for both grant types will be evaluated according to how topical and impactful they are:
Topical
This RFP is limited to research that aims to help maximize the societal benefit of AI, explicitly focusing not on the standard goal of making AI more capable, but on making AI more robust and/or beneficial. Funding priority will be given to research aimed at keeping AI robust and beneficial even if it comes to greatly supersede current capabilities, either by explicitly focusing on issues related to advanced future AI or by focusing on near- term problems, the solutions of which are likely to be important first steps toward long-term solutions.
Appropriate research topics for Project Grants span multiple fields and include questions such as (a longer list of example questions is given here):
A. Computer Science:
- Verification: how to prove that a system satisfies certain desired formal properties. (“Did I build the system right?”)
- Validity: how to ensure that a system that meets its formal requirements does not have unwanted behaviors and consequences. (“Did I build the right system?”)
- Security: how to prevent intentional manipulation by unauthorized parties. •Control: how to enable meaningful human control over an AI system after it begins to operate.
B. Law and ethics:
- How should the law handle liability for autonomous systems? Must some autonomous systems remain under meaningful human control?
- Should some categories of autonomous weapons be banned?
- Machine ethics: How should an autonomous vehicle trade off, say, a small probability of injury to a human against the near-certainty of a large material cost? Should such trade-offs be the subject of national standards?
- To what extent can/should privacy be safeguarded as AI gets better at interpreting the data obtained from surveillance cameras, phone lines, emails, shopping habits, etc.?
C. Economics:
- Labor market forecasting
- Labor market policy
- How can a low-employment society flourish?
D. Education and outreach:
- Summer/winter schools on AI and its relation to society, targeted at AI graduate students and postdocs
- Non-technical mini-schools/symposia on AI targeted at journalists, policymakers, philanthropists and other opinion leaders.
This RFP solicits Center Grants on the topic of AI policy, including forecasting. Proposed centers should address questions spanning (but not limited to) the following:
- What is the space of AI policies worth studying? Possible dimensions include implementation level (global, national, organizational, etc.), strictness (mandatory regulations, industry guidelines, etc.) and type (policies/monitoring focused on software, hardware, projects, individuals, etc.)
- Which criteria should be used to determine the merits of a policy? Candidates include verifiability of compliance, enforceability, ability to reduce risk, ability to avoid stifling desirable technology development, adoptability, and ability to adapt over time to changing circumstances to prevent intentional manipulation by unauthorized parties.
- Which policies are best when evaluated against these criteria of merit? Addressing this question (which is anticipated to involve the lion’s share of the proposed work) would include detailed forecasting of how AI development will unfold under different policy options.
The relative amount of funding for different areas is not predetermined, but will be optimized to reflect the number and quality of applications received. Very roughly, the expectation is ~50% computer science, ~20% policy,, ~15% law, ethics & economics, and ~15% education.
Impactful
Proposals will be rated according to their expected positive impact per dollar, taking all relevant factors into account, such as:
A. Intrinsic intellectual merit, scientific rigor and originality
B. A high product of likelihood for success and importance if successful (i.e., high-risk research can be supported as long as the potential payoff is also very high)
C. The likelihood of the research opening fruitful new lines of scientific inquiry
D. The feasibility of the research in the given time frame
E. The qualifications of the Principal Investigator and team with respect to the proposed topic
F. The part a grant may play in career development
G. Cost effectiveness: Tight budgeting is encouraged in order to maximize the research impact of the project as a whole, with emphasis on scientific return per dollar rather than per proposal
H. Potential to impact the greater community as well as the general public via effective outreach and dissemination of the research results
To maximize its impact per dollar, this RFP is intended to complement, not supplement, conventional funding. We wish to enable research that, because of its long-term focus or its non-commercial, speculative or non-mainstream nature would otherwise go unperformed due to lack of available resources. Thus, although there will be inevitable overlaps, an otherwise scientifically rigorous proposal that is a good candidate for an FLI grant will generally not be a good candidate for funding by the NSF, DARPA, corporate R&D, etc.—and vice versa. To be eligible, research must focus on making AI more robust/beneficial as opposed to the standard goal of making AI more capable. To aid prospective applicants in determining whether a project is appropriate for FLI, we have provided lists of questions and topics that make suitable targets for research funded under this program on the Examples page. Applicants can also review projects supported under prior Large Grant programs.
Acceptable use of grant funds for Project Grants include:
- Student/postdoc/researcher salary and benefits
- Summer salary and teaching buyout for academics
- Support for specific projects during sabbaticals
- Assistance in writing or publishing books or journal articles, including page charges
- Modest allowance for travel and other relevant
- Modest allowance for justifiable lab equipment, computers, and other research supplies
- Modest travel allowance
- Development of workshops, conferences, or lecture series for professionals in the relevant fields
- Overhead of at most 15% (Please note if this is an issue with your institute, or if your organization is not non-profit, you can contact FLI to learn about other organizations that can help administer an FLI grant for you.)
Subawards are discourages in the case of Project Grants, but perfectly acceptable for Center Grants.
III. Application Process
Applications will be accepted electronically through a standard form on our website (click here for application) and evaluated in a two-part process, as follows:
1. INITIAL PROPOSAL—DUE March 1 2015—Must include:
- A summary of the project, explicitly addressing why it is topical and impactful. These should be 300-500 words for Projects Grants and 500-1000 words for Center Grants.
- A draft budget description not exceeding 200 words, including an approximate total cost over the life of the award and explanation of how funds would be spent
- A Curriculum Vitae for the Principal Investigator, which MUST be in PDF format, including:
- Education and employment history
- A list of references of up to five previous publications relevant to the proposed research and up to five additional representative publications
- Full publication list
- For Center Grants only: listing and brief bio of Center Co-Investigators, including if applicable the lead investigator at each institution that is part of the center.
A review panel assembled by FLI will screen each Initial Proposal according to the criteria in Section III. Based on their assessment, the Principal Investigator (PI) may be invited to submit a Full Proposal, on or about March 21 2015, perhaps with feedback from FLI on improving the proposal. Please keep in mind that however positive FLI may be about a proposal at any stage, it may still be turned down for funding after full peer review.
2. FULL PROPOSAL—DUE May 17 2015. Must Include:
- Cover sheet
- A 200-word project abstract, suitable for publication in an academic journal
- A project summary not exceeding 200 words, explaining the work and its significance to laypeople
- A detailed description of the proposed research, not to exceed 15 (20 pages for Center Grants) single-spaced 11-point pages, including a short statement of how the application fits into the applicant’s present research program, and a description of how the results might be communicated to the wider scientific community and general public
- A detailed budget over the life of the award, with justification and utilization distribution (preferably drafted by your institution’s grant officer or equivalent)
- A list, for all project senior personnel, of all present and pending financial support, including project name, funding source, dates, amount, and status (current or pending)
- Evidence of tax-exempt status of grantee institution, if other than a US university. For information on determining tax-exempt status of international organizations and institutes, please review the information here.
- Names of three recommended referees
- Curricula Vitae for all project senior personnel, including:
- Education and employment history
- A list of references of up to five previous publications relevant to the proposed research, and up to five additional representative publications
- Full publication list
- Additional material may be requested in the case of Center Grants, as specified in the invitation and feedback phase.
Completed Full Proposals will undergo a competitive process of external and confidential expert peer review, evaluated according to the criteria described in Section III. A review panel of scientists in the relevant fields will be convened to produce a final rank ordering of the proposals, which will determine the grant winners, and make budgetary adjustments if necessary. Public award recommendations will be made on or about July 1, 2015, 2015.
IV. Funding Process
The peer review and administration of this grants program will be managed by the Future of Life Institute (FLI), futureoflife.blackfin.biz. FLI is an independent, philanthropically funded non-profit organization whose mission is to catalyze and support research and initiatives for safeguarding life and developing optimistic visions of the future, including positive ways for humanity to steer its own course considering new technologies and challenges.
FLI will direct these grants through a Donor Advised Fund (DAF) at the Silicon Valley Community Foundation. FLI will solicit grant applications and have them peer reviewed, and on the basis of these reviews, FLI will advise the DAF on what grants to make. After grants have been made by the DAF, FLI will work with the DAF to monitor the grantee’s performance via grant reports. In this way, researchers will continue to interact with FLI, while the DAF interacts mostly with their institutes’ administrative or grants management offices.
Our other grant programs
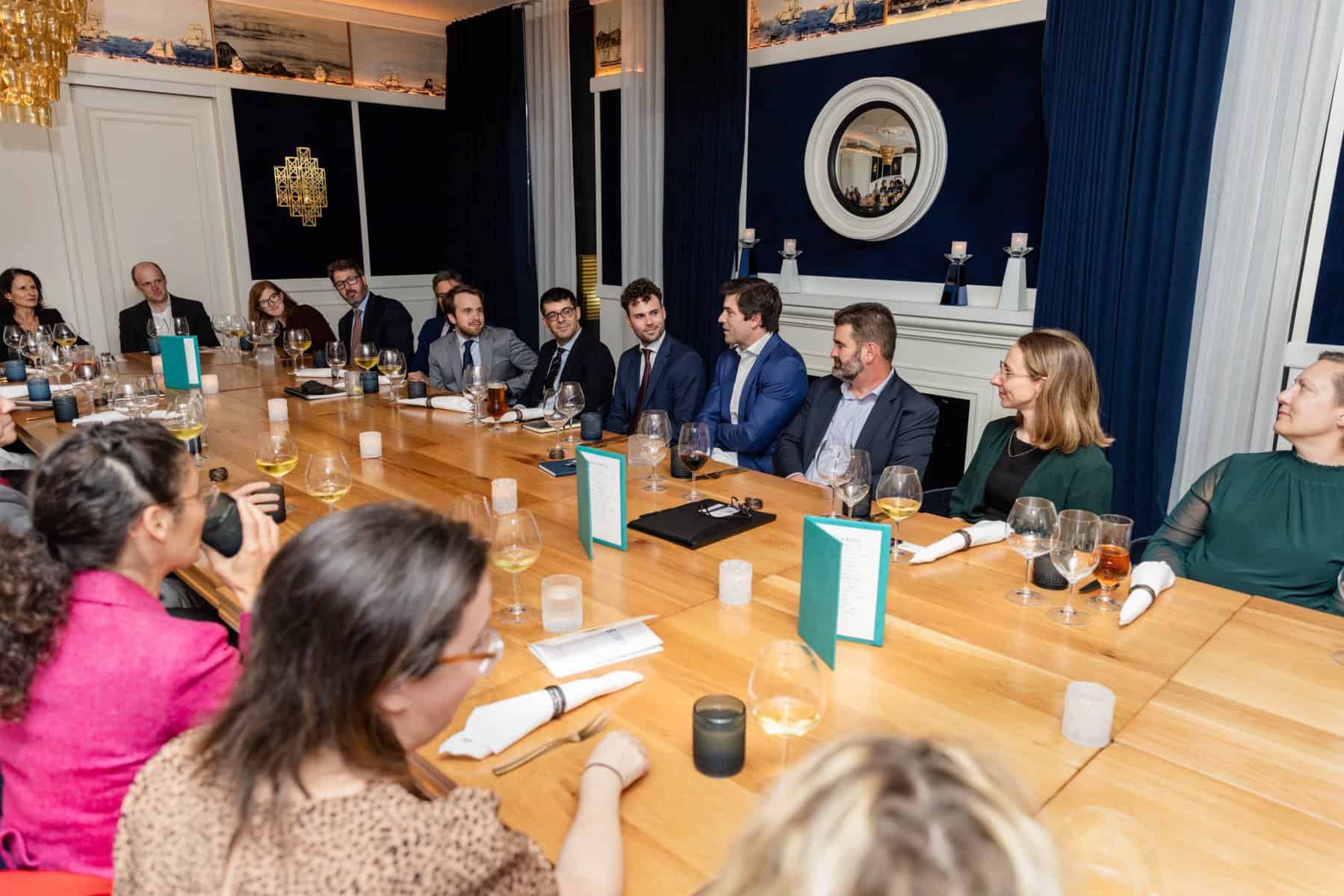
2024 Grants
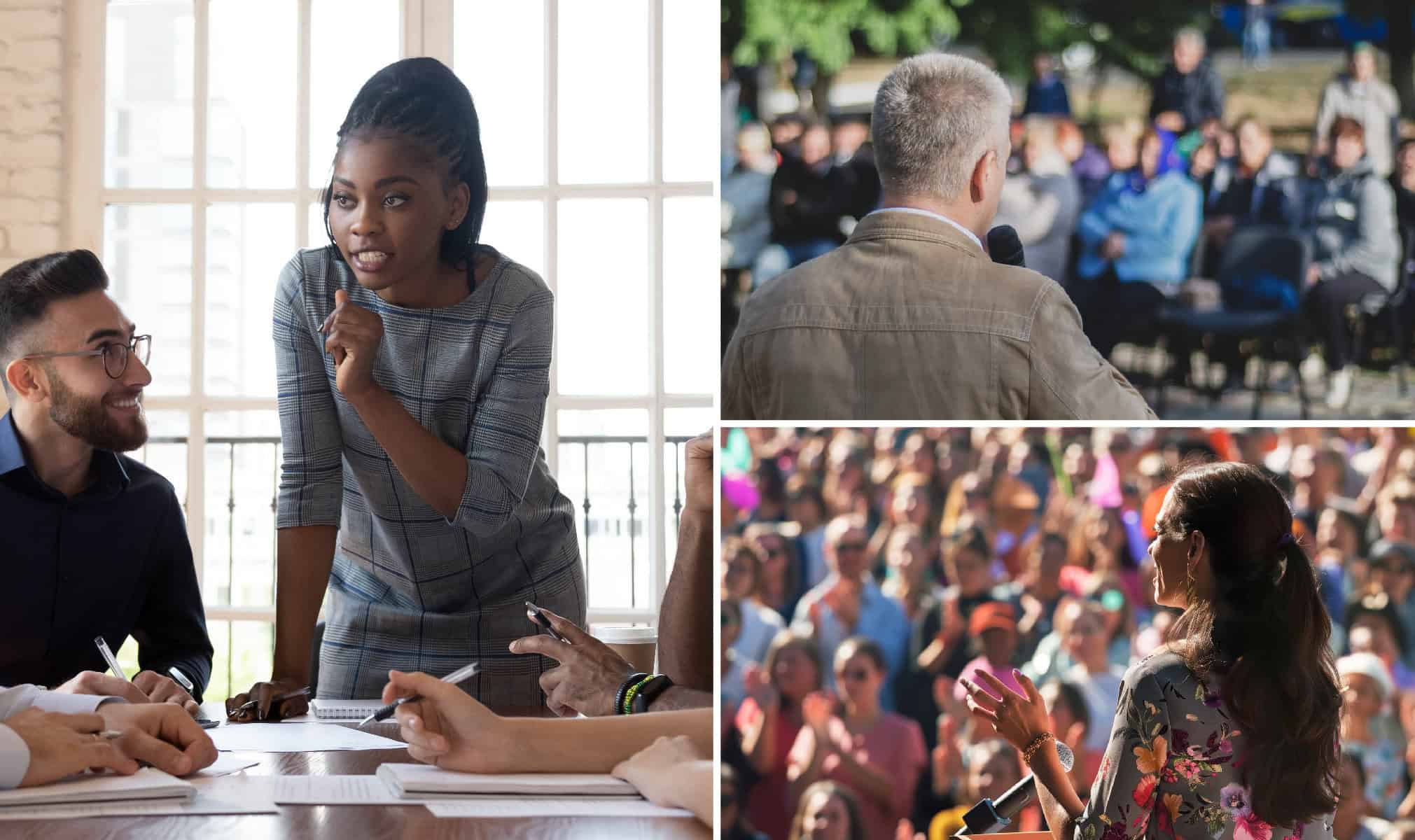
Multistakeholder Engagement for Safe and Prosperous AI
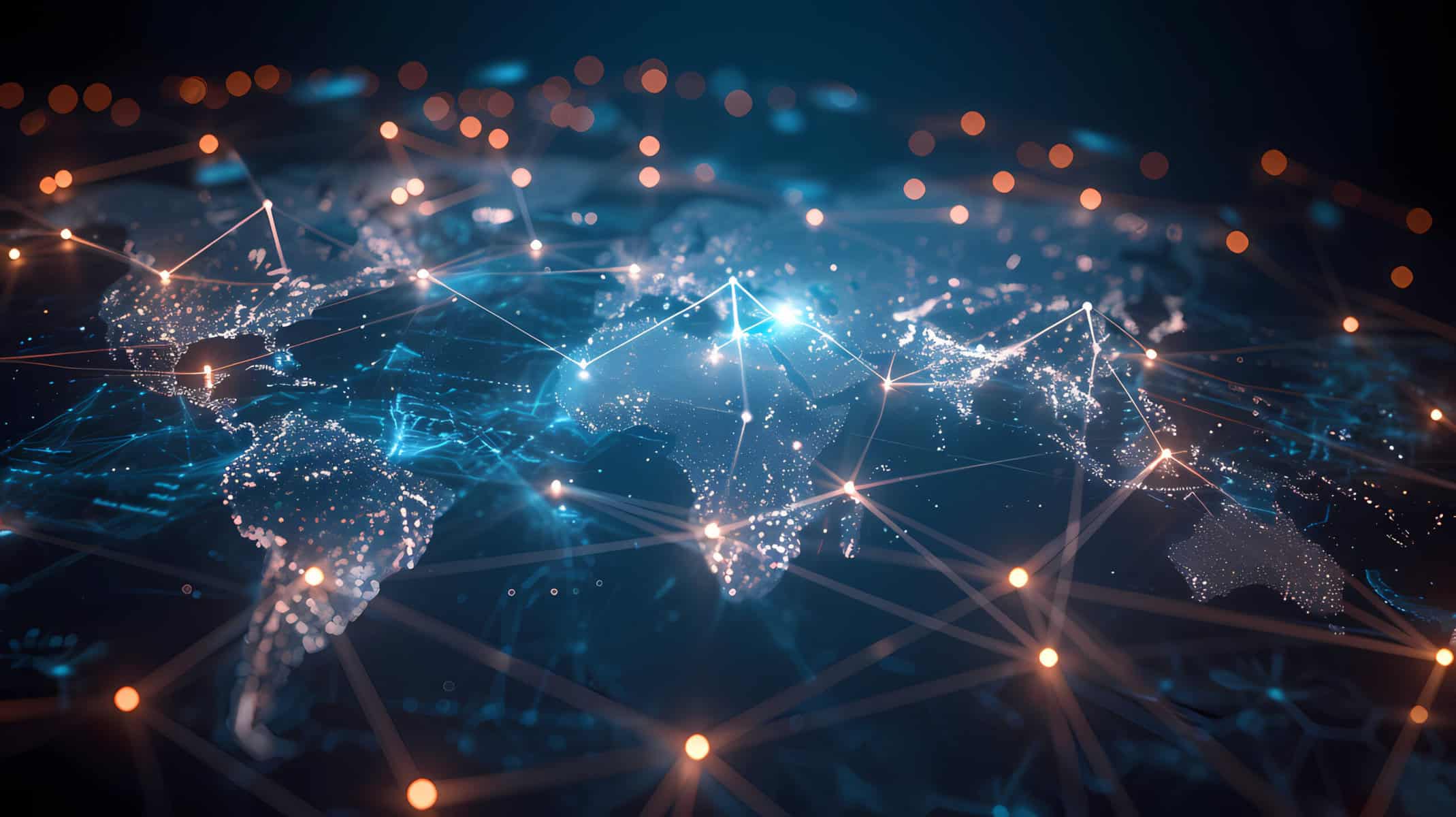